A Multi-Task Deep Learning System to Classify Diabetic Macular Edema for Different Optical Coherence Tomography Devices: A Multi-Center Validation Analysis
semanticscholar(2021)
摘要
Purpose: Diabetic macular edema (DME) is a primary cause of irreversible vision loss in individuals with diabetes mellitus (DM). We aimed to develop and validate a deep-learning (DL) based system that can classify DME (center-involved DME [CI-DME], non-CI-DME or normal), and non-DME retinal abnormalities (presence or absence of other retinal abnormalities) obtained from three types of commercially available optical coherence tomography (OCT) devices. Methods: Two versions of multi-task networks, one based on 3D ResNet34 and one based on 2D ResNet18, with uncertainty-driven deep multiple instance learning (UD-MIL) pipelined for images obtained from 3 commercially available OCT devices, were trained for classifying DME and other retinal abnormalities in the training/validation dataset of 73,746 OCT images (3,788 volumetric scans from Cirrus HD-OCT, 30,515 B-scans from Spectralis OCT, and 39,443 Bscans from Triton OCT) from 2,444 eyes of 1,238 subjects with DM from Hong Kong. External testing was performed on 7 unseen datasets of 26,981 images (3,218 volumetric scans from Cirrus HD-OCT, 18,295 B-scans from Spectralis OCT, and 5,468 B-scans from Triton OCT) from 3,402 eyes of 1,868 subjects with DM from Singapore, Hong Kong, US, China, and Australia. Results: For the classification of presence versus absence of any DME, the DL system achieved the area under the receiver operating characteristic curve (AUROC) of 0.937, 0.958, and 0.965 in primary datasets obtained from Cirrus, Spectralis, and Triton OCTs, respectively, and AUROCs > 0.906 in external unseen datasets. For classifying CI-DME versus non-CI-DME, the AUROCs were of 0.968, 0.951, and 0.975 in primary datasets obtained from Cirrus, Spectralis, and Triton OCTs, respectively, and AUROCs >0.894 in the external datasets. In addition, the DL system classified presence versus absence of other retinal abnormalities with AUROCs of 0.948, 0.949, and 0.938 in primary datasets obtained from Cirrus, Spectralis, and Triton OCTs, respectively, and AUROCs >0.898 in the external datasets. Conclusion: The excellent performance achieved with this multi-task DL system for automated classification of DME and other retinal abnormalities in OCT images, highlighted its potential as an efficient and effective DME screening tool that can save resources and speed up workflow substantially.
更多查看译文
AI 理解论文
溯源树
样例
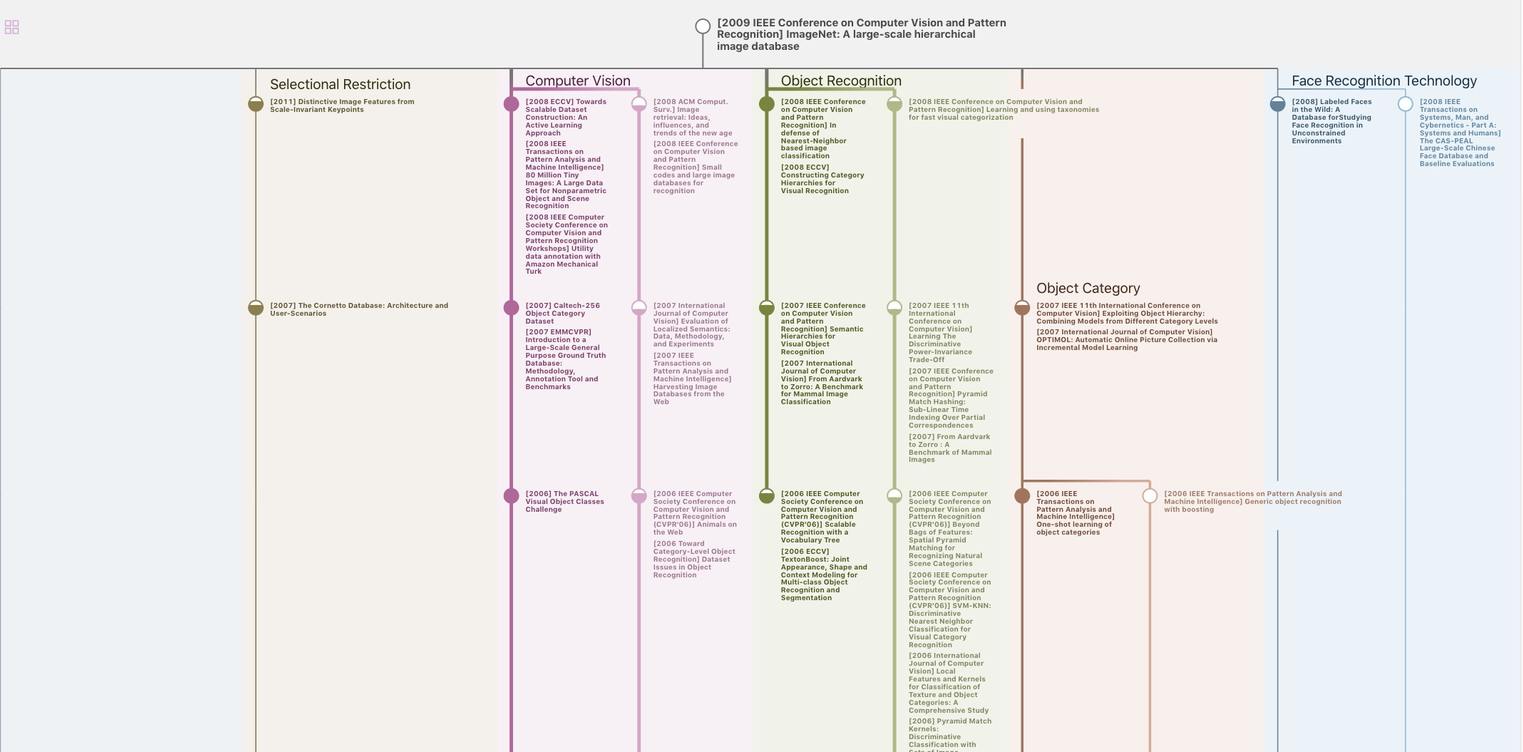
生成溯源树,研究论文发展脉络
Chat Paper
正在生成论文摘要