Multi-Coil MRI Reconstruction Challenge -- Assessing Brain MRI Reconstruction Models and their Generalizability to Varying Coil Configurations
FRONTIERS IN NEUROSCIENCE(2020)
摘要
Deep-learning-based brain magnetic resonance imaging (MRI) reconstruction methods have the potential to accelerate the MRI acquisition process. Nevertheless, the scientific community lacks appropriate benchmarks to assess MRI reconstruction quality of high-resolution brain images, and evaluate how these proposed algorithms will behave in the presence of small, but expected data distribution shifts. The Multi-Coil Magnetic Resonance ImPreprint submitted to arXiv December 23, 2021 ar X iv :2 01 1. 07 95 2v 2 [ ee ss .I V ] 2 1 D ec 2 02 1 age (MC-MRI) Reconstruction Challenge provides a benchmark that aims at addressing these issues, using a large dataset of high-resolution, threedimensional, T1-weighted MRI scans. The challenge has two primary goals: 1) to compare different MRI reconstruction models on this dataset and 2) to assess the generalizability of these models to data acquired with a different number of receiver coils. In this paper, we describe the challenge experimental design, and summarize the results of a set of baseline and state of the art brain MRI reconstruction models. We provide relevant comparative information on the current MRI reconstruction state-of-the-art and highlight the challenges of obtaining generalizable models that are required prior to broader clinical adoption. The MC-MRI benchmark data, evaluation code and current challenge leaderboard are publicly available. They provide an objective performance assessment for future developments in the field of brain MRI reconstruction.
更多查看译文
关键词
machine learning, magnetic resonance imaging (MRI), benchmark, image reconstruction, inverse problems, brain imaging
AI 理解论文
溯源树
样例
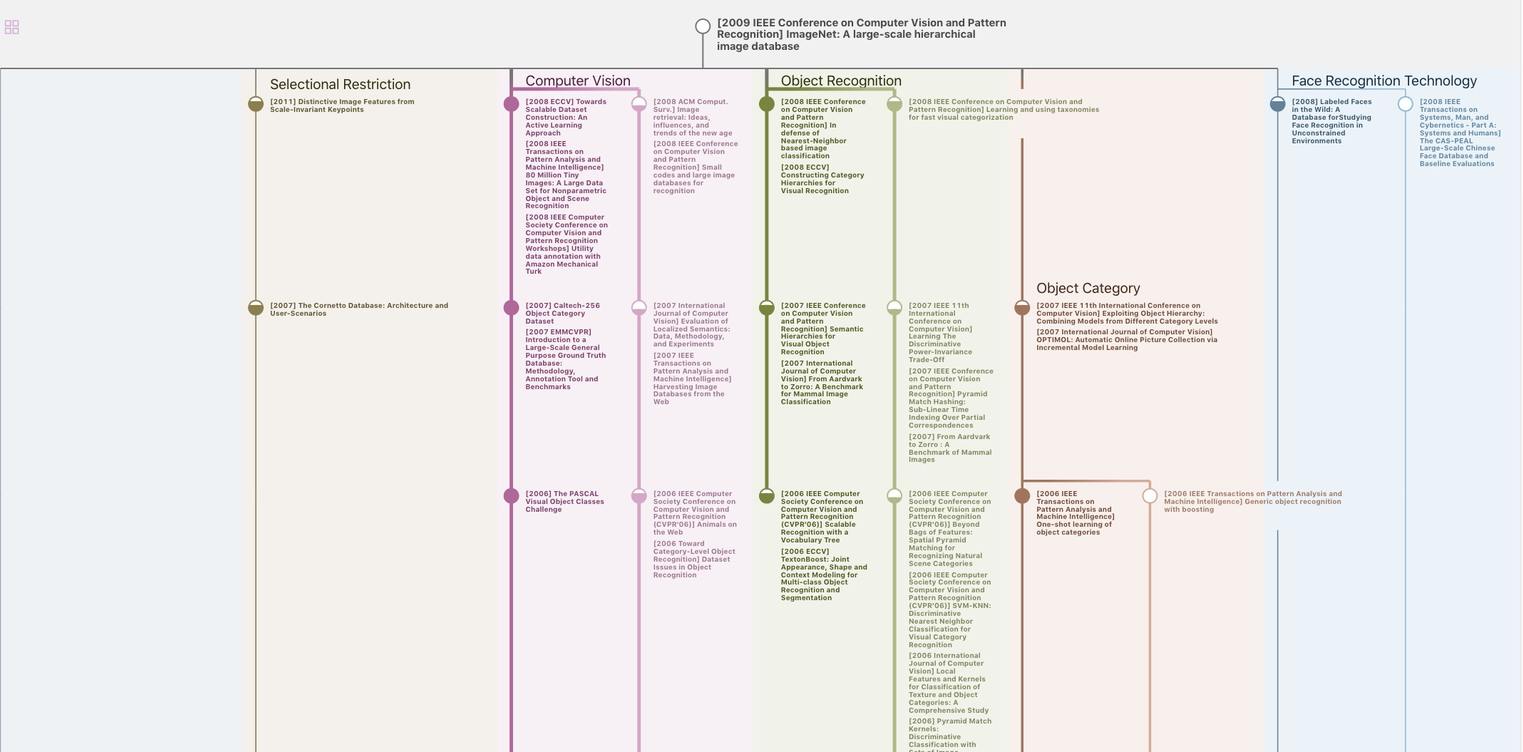
生成溯源树,研究论文发展脉络
Chat Paper
正在生成论文摘要