Local Structure Matters Most: Perturbation Study in NLU
Annual Meeting of the Association for Computational Linguistics(2022)
摘要
Recent research analyzing the sensitivity of natural language understanding models to wordorder perturbations has shown that neural models are surprisingly insensitive to the order of words. In this paper, we investigate this phenomenon by developing order-altering perturbations on the order of words, subwords, and characters to analyze their effect on neural models' performance on language understanding tasks. We experiment with measuring the impact of perturbations to the local neighborhood of characters and global position of characters in the perturbed texts and observe that perturbation functions found in prior literature only affect the global ordering while the local ordering remains relatively unperturbed. We empirically show that neural models, invariant of their inductive biases, pretraining scheme, or the choice of tokenization, mostly rely on the local structure of text to build understanding and make limited use of the global structure.
更多查看译文
关键词
local structure,perturbation study
AI 理解论文
溯源树
样例
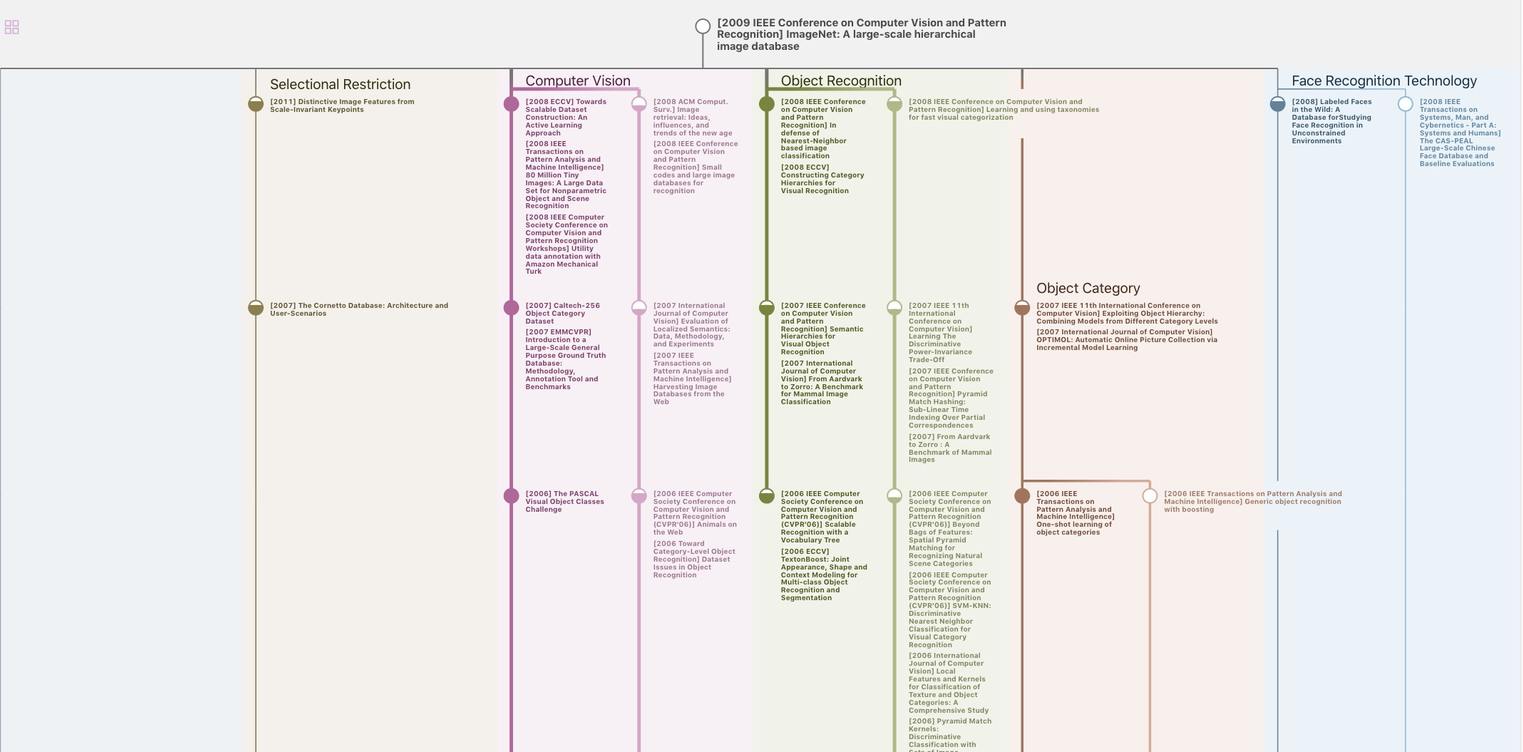
生成溯源树,研究论文发展脉络
Chat Paper
正在生成论文摘要