A Rectal CT Tumor Segmentation Method Based on Improved U-Net
INTERNATIONAL JOURNAL OF PATTERN RECOGNITION AND ARTIFICIAL INTELLIGENCE(2022)
摘要
Automatic and accurate segmentation of tumor area from rectal CT image plays an extremely key role in the treatment and diagnosis of rectal cancer. This paper proposes the MR-U-Net network model. The improvement is that a pair of encoder and decoder is added longitudinally to the U-shaped structure, which is the network structure of the fifth layer, and a residual module is added horizontally to the encoder and decoder of each layer. This model is used to conduct targeted research on the automatic segmentation method of rectal cancer. [H. Gao et al., Rectal tumor segmentation method based on U-Net improved model, J. Comput. Appl. 40 (8) (2020) 2392-2397] also improved U-Net and used the same dataset as this paper, but the Dice coefficient of all targets was only 83.15%, and the Dice coefficient of small targets was only 87.17%. This paper evaluates the improved MR-U-Net network model with the three indicators of precision, recall and Dice coefficient, and finds that in comparison to Ref. 4 the precision is 95.13%, 2.29% higher than the former work, recall is 94.28%, higher than the former work by 0.34%, Dice coefficient of all targets is 88.45%, increased by 5.3% compared with the former work, and the small targets Dice coefficient is increased by 1.28%, which is the best optimization state of this paper. Experiments show that for datasets with extremely skewed positive and negative samples, the MR-U-Net network structure after improving the hyperparameters in the optimizer can more accurately segment the rectal CT tumor lesion area.
更多查看译文
关键词
Rectal cancer,medical image segmentation,U-Net,residual module,Dice coefficient
AI 理解论文
溯源树
样例
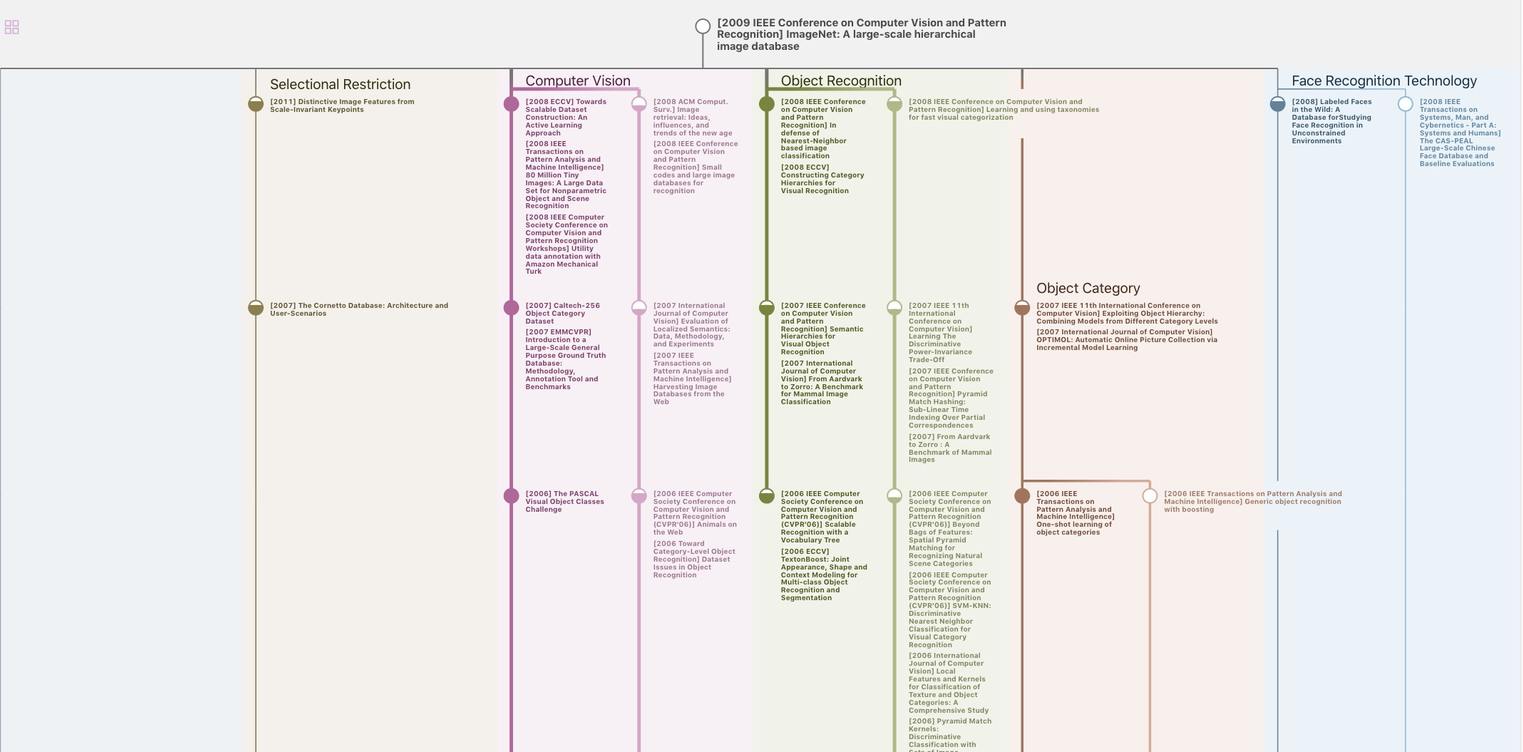
生成溯源树,研究论文发展脉络
Chat Paper
正在生成论文摘要