MOT: a Multi-Omics Transformer for Multiclass Classification Tumour Types Predictions
BIOINFORMATICS(2022)
摘要
Motivation: Breakthroughs in high-throughput technologies and machine learning methods have enabled the shift towards multi-omics modeling as the preferred mean to understand the mechanisms underlying biological processes, and to improve complex disease prognosis in clinical settings. However, most multi-omic studies only use transcriptomics and epigenomics due to their over-representation in databases and their early technical maturity compared to others omics. For complex phenotypes and mechanisms, not leveraging all the omics despite their varying degree of availability can lead to a failure to understand the underlying biological mechanisms. Results: We proposed MOT (Multi-Omic Transformer), a deep learning based model using the transformer architecture, that discriminates complex phenotypes (herein cancers types) based on five omics data type regardless of their availability: transcriptomics (mRNA and miRNA), epigenomics (DNA methylation), copy number variations (CNVs), and proteomics. At its core, MOT uses a data augmentation scheme that allows it to handle missing omics views and its attention layers give a macro level of interpretability for each phenotypes. Indeed, MOT identifies the required omic type for the best prediction for each phenotype and therefore could guide clinical decision making when acquiring data to confirm a diagnostic. It achieves an accuracy score of 96.04% after 5-fold cross-validation among 33 tumour types. The newly introduced model can integrate and analyse five different omics data while handling the missing omics views and can also identify the essential omics data for the tumour multiclass classification tasks. Availability and implementation: MOT source code is available at https://github.com/dizam92/multiomic_predictions.
更多查看译文
关键词
classification,multi-omics
AI 理解论文
溯源树
样例
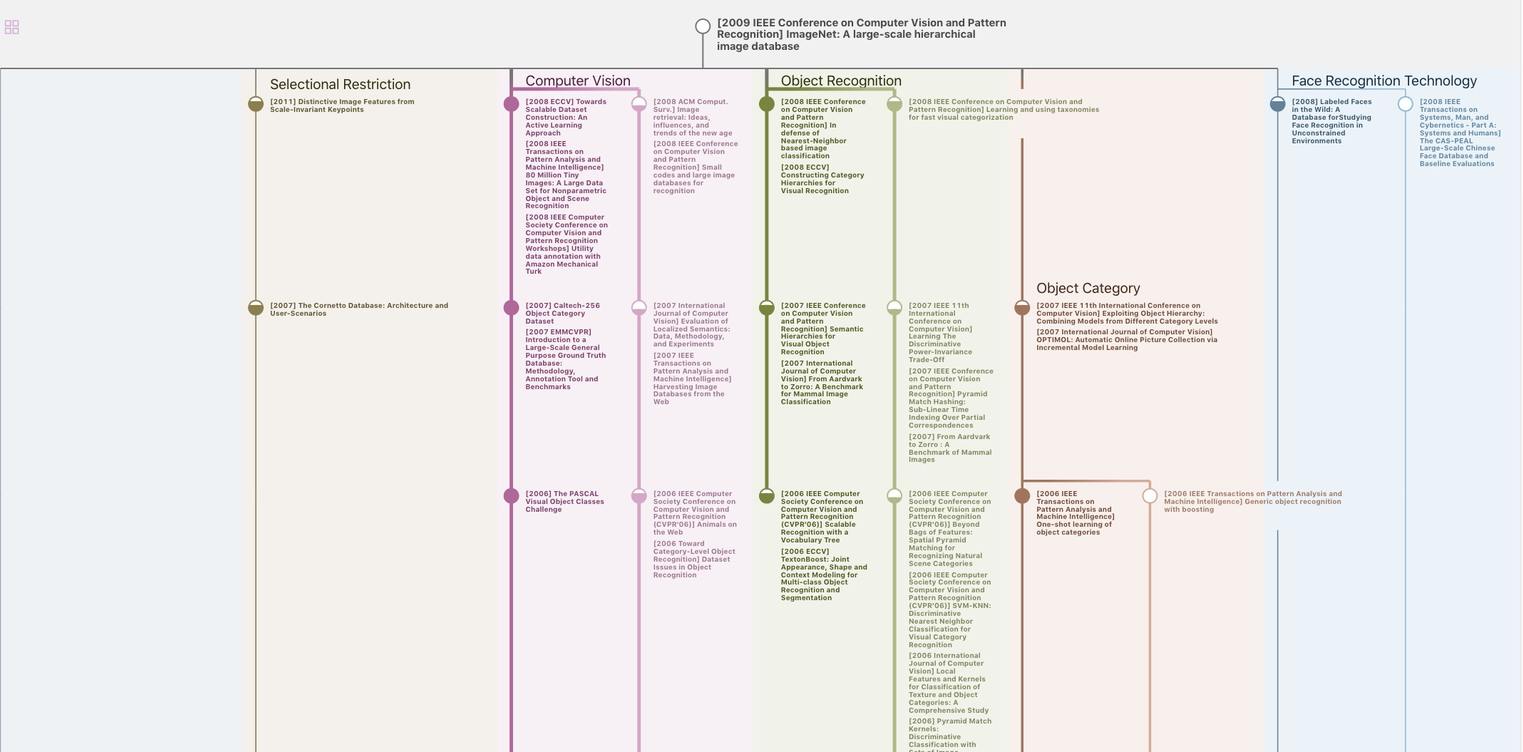
生成溯源树,研究论文发展脉络
Chat Paper
正在生成论文摘要