Partially Autoregressive Machine Learning: Development and Testing of Methods to Predict United States Air Force Retention
SSRN Electronic Journal(2022)
摘要
Establishing effective personnel management policies in the United States Air Force (USAF) requires methods to predict the number of personnel remaining in the USAF for different lengths of time in the future. Defined as the Personnel Retention Problem (PRP), determining this type of aggregate survival rate is a time series regression problem that shares many characteristics with binary classification problems. The limitations of this particular structure are particularly difficult to overcome for problems with limited data like the USAF PRP. We develop and test several machine learning models to produce improved retention predictions compared to the USAF’s current Kaplan Meier model. In addition to traditional random forest models and feedforward neural networks, we propose the inclusion of a partially autoregressive feature to extend the benefits of low-capacity autoregressive techniques to higher-capacity machine learning techniques. We present a Partially Autoregressive Neural Network (PARNet) and a Partially Autoregressive Random Forest (PARFor) and test the performance of each technique across a range of hyperparameter values. We select the superlative model using a validation dataset, compare results to the existing benchmark model, and find a 62.8% reduction in aggregate prediction error for the baseline neural network and 34.8% reduction for the PARNet.
更多查看译文
关键词
Autoregressive,Machine learning,Time series,Personnel retention
AI 理解论文
溯源树
样例
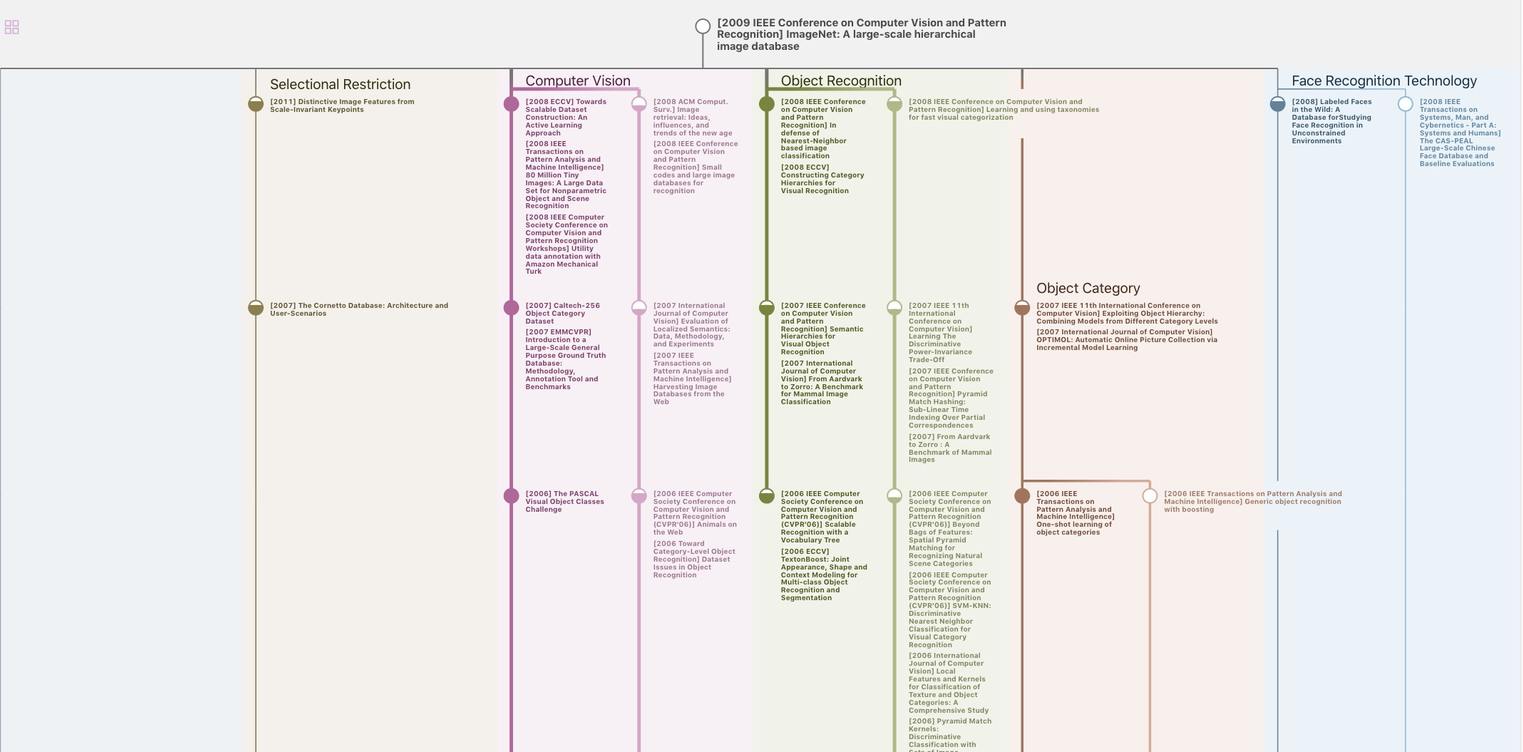
生成溯源树,研究论文发展脉络
Chat Paper
正在生成论文摘要