On Integrating the Number of Synthetic Data Sets m into the a priori Synthesis Approach
PRIVACY IN STATISTICAL DATABASES, PSD 2022(2022)
摘要
The synthesis mechanism given in [4] uses saturated models, along with overdispersed count distributions, to generate synthetic categorical data. The mechanism is controlled by tuning parameters, which can be tuned according to a specific risk or utility metric. Thus expected properties of synthetic data sets can be determined analytically a priori, that is, before they are generated. While [4] considered the case of generating m = 1 data set, this paper considers generating m > 1 data sets. In effect, m becomes a tuning parameter and the role of m in relation to the risk-utility trade-off can be shown analytically. The paper introduces a pair of risk metrics, tau(3)(k, d) and tau(4)(k, d), that are suited to m > 1 data sets; and also considers the more general issue of how best to analyse m > 1 categorical data sets: average the data sets pre-analysis or average results post-analysis. Finally, the methods are demonstrated empirically with the synthesis of a constructed data set which is used to represent the English School Census.
更多查看译文
关键词
Synthetic data, Privacy, Categorical data, Risk metrics, Contingency tables
AI 理解论文
溯源树
样例
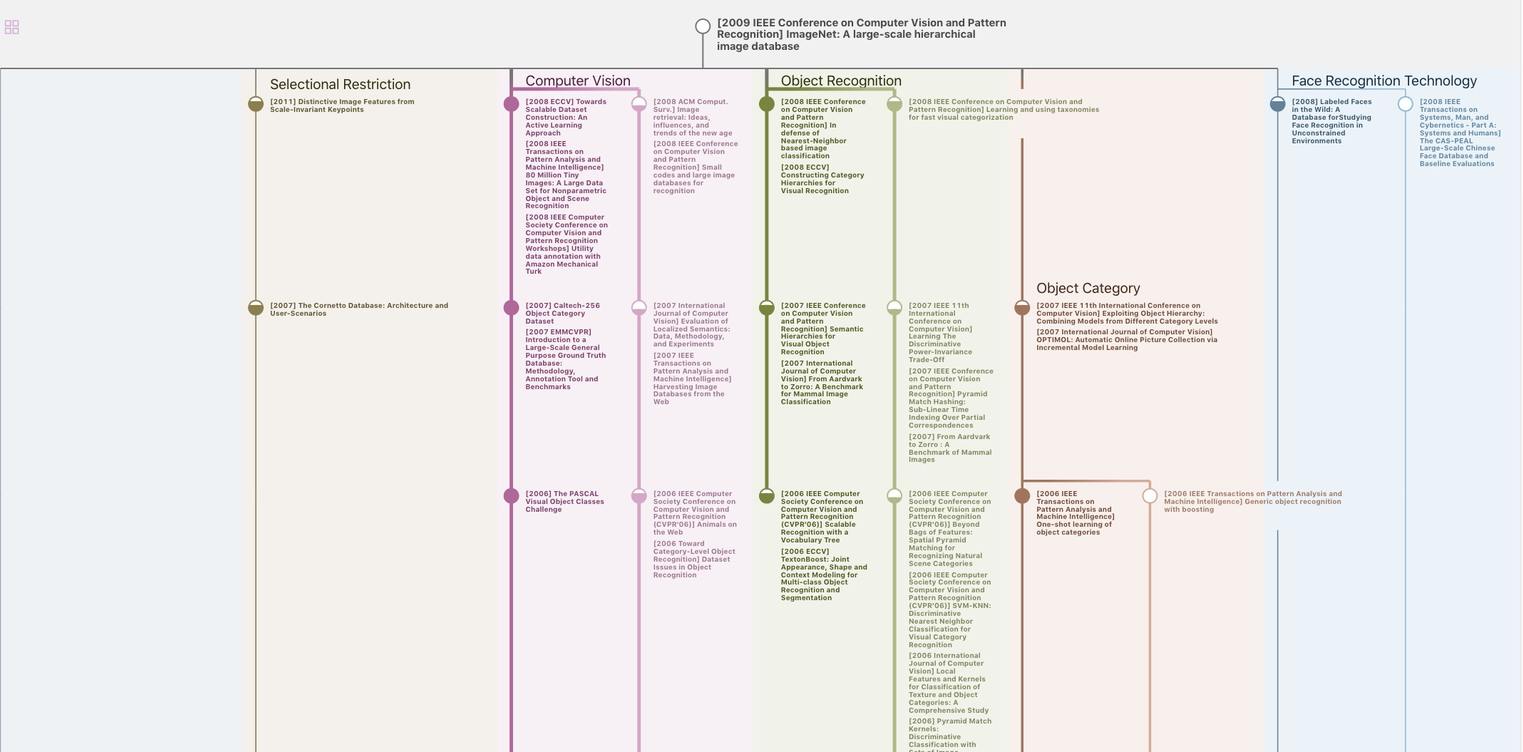
生成溯源树,研究论文发展脉络
Chat Paper
正在生成论文摘要