S3E-GNN: Sparse Spatial Scene Embedding with Graph Neural Networks for Camera Relocalization
arxiv(2022)
摘要
Camera relocalization is the key component of simultaneous localization and mapping (SLAM) systems. This paper proposes a learning-based approach, named Sparse Spatial Scene Embedding with Graph Neural Networks (S3E-GNN), as an end-to-end framework for efficient and robust camera relocalization. S3E-GNN consists of two modules. In the encoding module, a trained S3E network encodes RGB images into embedding codes to implicitly represent spatial and semantic embedding code. With embedding codes and the associated poses obtained from a SLAM system, each image is represented as a graph node in a pose graph. In the GNN query module, the pose graph is transformed to form a embedding-aggregated reference graph for camera relocalization. We collect various scene datasets in the challenging environments to perform experiments. Our results demonstrate that S3E-GNN method outperforms the traditional Bag-of-words (BoW) for camera relocalization due to learning-based embedding and GNN powered scene matching mechanism.
更多查看译文
关键词
camera relocalization,sparse spatial scene,graph neural networks,e-gnn
AI 理解论文
溯源树
样例
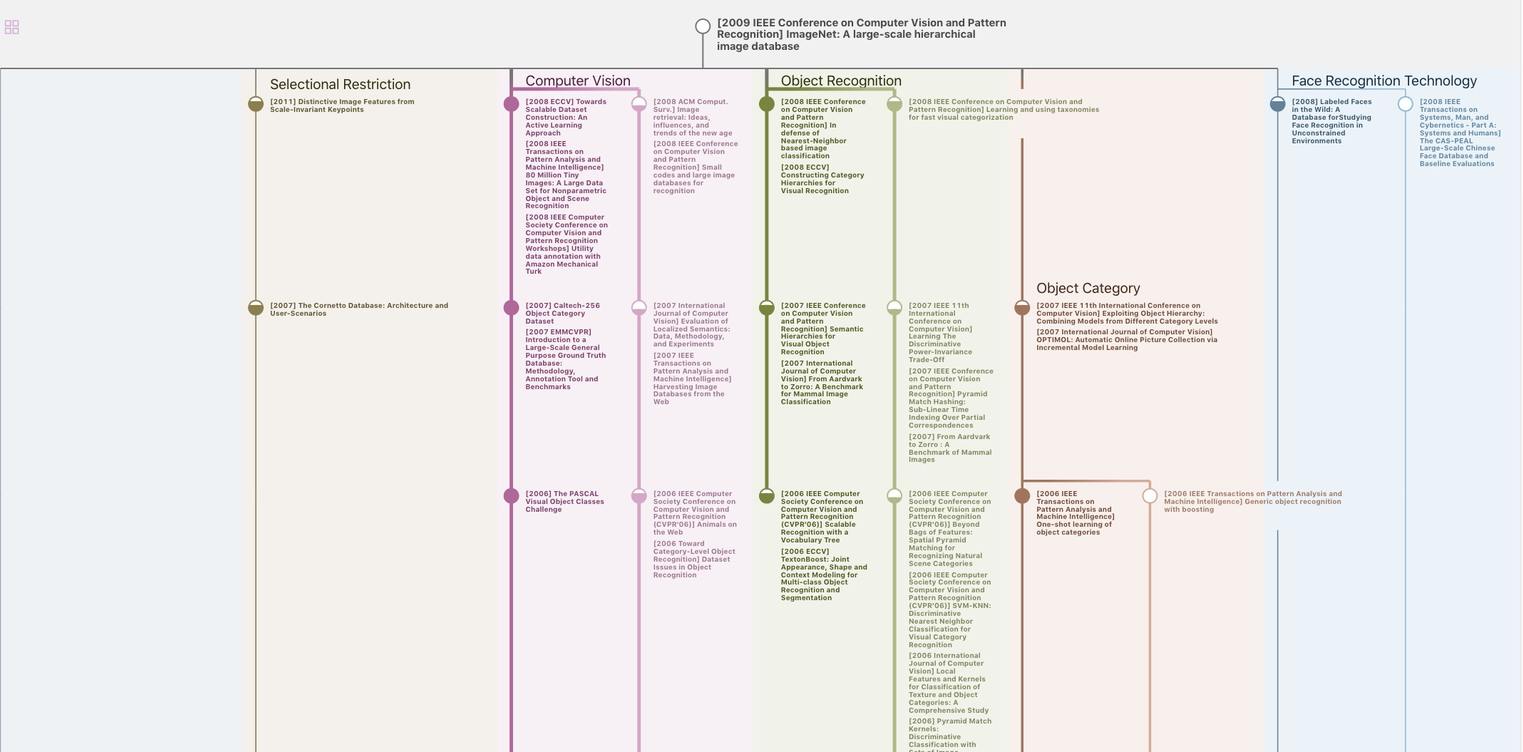
生成溯源树,研究论文发展脉络
Chat Paper
正在生成论文摘要