BenchMetrics Prob: benchmarking of probabilistic error/loss performance evaluation instruments for binary classification problems
INTERNATIONAL JOURNAL OF MACHINE LEARNING AND CYBERNETICS(2023)
摘要
Probabilistic error/loss performance evaluation instruments that are originally used for regression and time series forecasting are also applied in some binary-class or multi-class classifiers, such as artificial neural networks. This study aims to systematically assess probabilistic instruments for binary classification performance evaluation using a proposed two-stage benchmarking method called BenchMetrics Prob. The method employs five criteria and fourteen simulation cases based on hypothetical classifiers on synthetic datasets. The goal is to reveal specific weaknesses of performance instruments and to identify the most robust instrument in binary classification problems. The BenchMetrics Prob method was tested on 31 instrument/instrument variants, and the results have identified four instruments as the most robust in a binary classification context: Sum Squared Error ( SSE ), Mean Squared Error ( MSE ), Root Mean Squared Error ( RMSE , as the variant of MSE ), and Mean Absolute Error ( MAE ). As SSE has lower interpretability due to its [0, ∞) range, MAE in [0, 1] is the most convenient and robust probabilistic metric for generic purposes. In classification problems where large errors are more important than small errors, RMSE may be a better choice. Additionally, the results showed that instrument variants with summarization functions other than mean ( e.g. , median and geometric mean), LogLoss , and the error instruments with relative/percentage/symmetric-percentage subtypes for regression, such as Mean Absolute Percentage Error ( MAPE ), Symmetric MAPE ( sMAPE ), and Mean Relative Absolute Error ( MRAE ), were less robust and should be avoided. These findings suggest that researchers should employ robust probabilistic metrics when measuring and reporting performance in binary classification problems.
更多查看译文
关键词
Performance measures,Probabilistic error,loss,Squared error,Binary classification,Regression,Time series forecasting
AI 理解论文
溯源树
样例
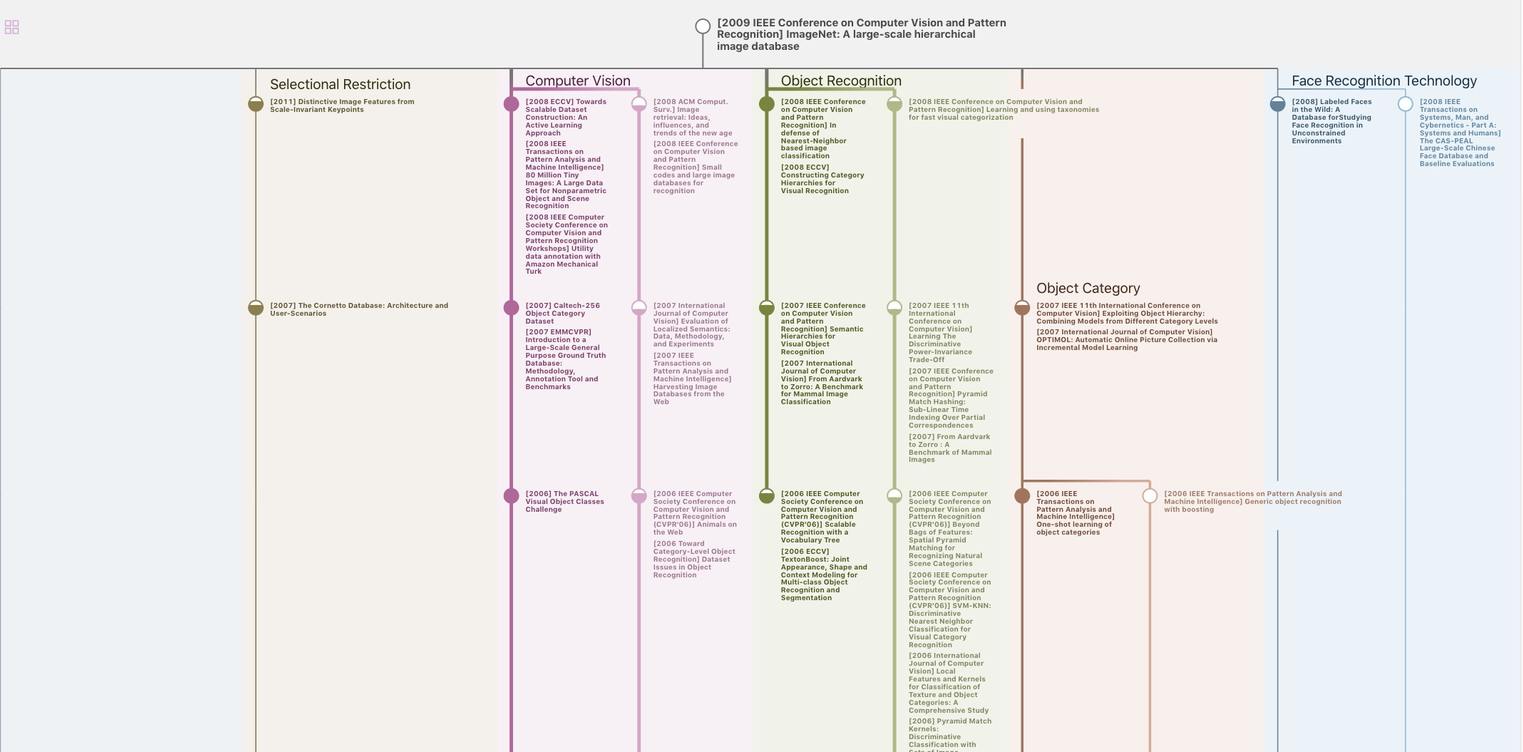
生成溯源树,研究论文发展脉络
Chat Paper
正在生成论文摘要