Do Language Models Learn Commonsense Knowledge?
semanticscholar(2021)
摘要
Language models (LMs) trained on large amounts of data (e.g., Brown et al., 2020; Patwary et al., 2021) have shown impressive performance on many NLP tasks under the zero-shot and few-shot setup. Here we aim to better understand the extent to which such models learn commonsense knowledge — a critical component of many NLP applications. To that end, we conduct a systematic and rigorous zero-shot and few-shot commonsense evaluation of pre-trained LMs, where we: (i) carefully control for the LM’s ability to exploit potential surface cues and annotation artefacts, and (ii) account for variations in model performance that arise from non-commonsense related factors. Our findings highlight the limitations of pre-trained LMs in acquiring commonsense knowledge without task-specific supervision; furthermore, using larger models — or augmenting the LMs with commonsense knowledge bases at test-time — did not substantially improve their performance. More broadly, our findings offer valuable lessons and best practices for conducting more rigorous multiplechoice evaluations of pre-trained LMs.
更多查看译文
AI 理解论文
溯源树
样例
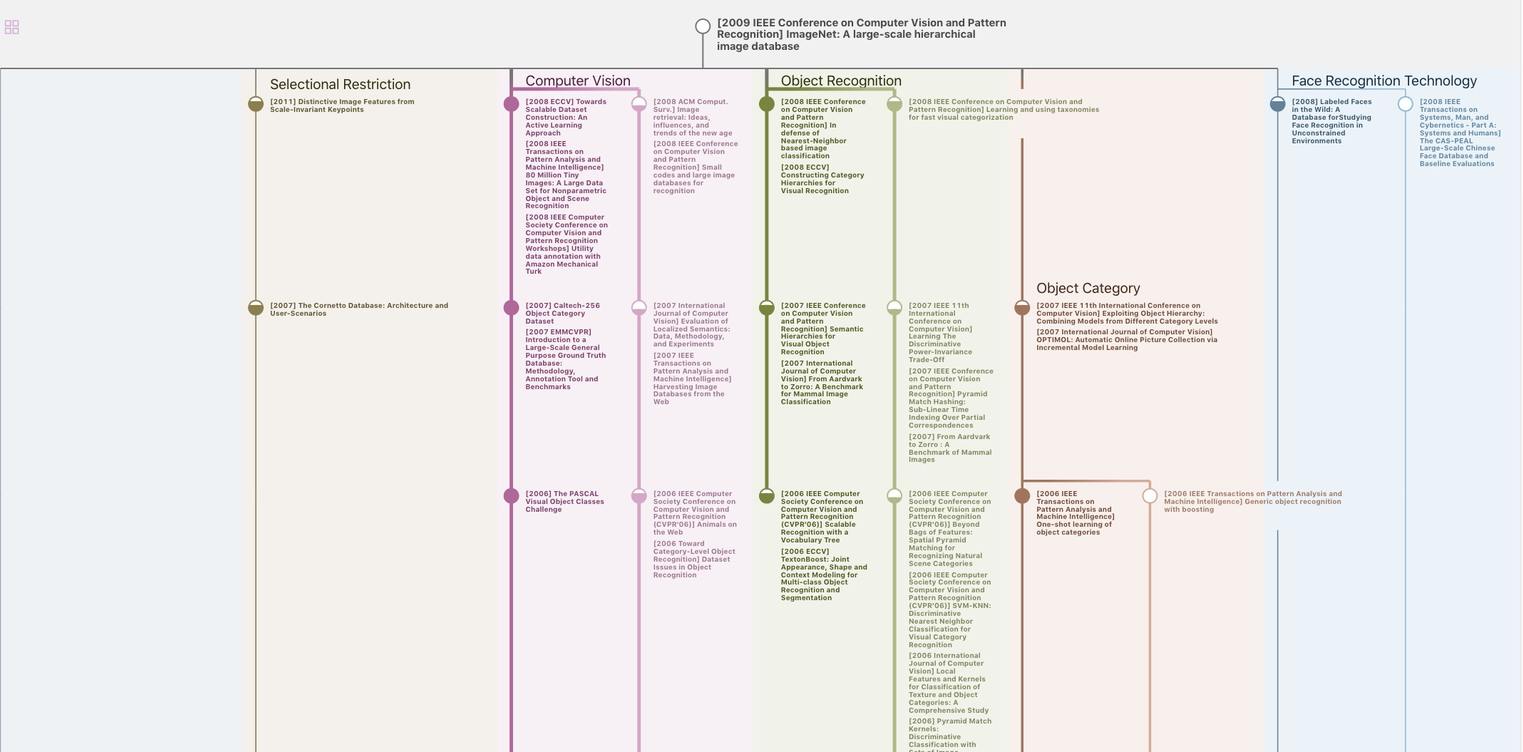
生成溯源树,研究论文发展脉络
Chat Paper
正在生成论文摘要