Streaming Submodular Maximization Under Matroid Constraints
International Colloquium on Automata, Languages and Programming (ICALP)(2022)
摘要
Recent progress in (semi-)streaming algorithms for monotone submodular function maximization has led to tight results for a simple cardinality constraint. However, current techniques fail to give a similar understanding for natural generalizations, including matroid constraints. This paper aims at closing this gap. For a single matroid of rank k (i.e., any solution has cardinality at most k), our main results are: A single-pass streaming algorithm that uses Õ(k) memory and achieves an approximation guarantee of 0.3178. A multi-pass streaming algorithm that uses Õ(k) memory and achieves an approximation guarantee of (1 − 1/e − ε) by taking a constant (depending on ε) number of passes over the stream. This improves on the previously best approximation guarantees of 1/4 and 1/2 for single-pass and multi-pass streaming algorithms, respectively. In fact, our multi-pass streaming algorithm is tight in that any algorithm with a better guarantee than 1/2 must make several passes through the stream and any algorithm that beats our guarantee of 1− 1/e must make linearly many passes (as well as an exponential number of value oracle queries). Moreover, we show how the approach we use for multi-pass streaming can be further strengthened if the elements of the stream arrive in uniformly random order, implying an improved result for p-matchoid constraints. 2012 ACM Subject Classification Mathematics of computing → Submodular optimization and polymatroids; Theory of computation → Streaming models
更多查看译文
AI 理解论文
溯源树
样例
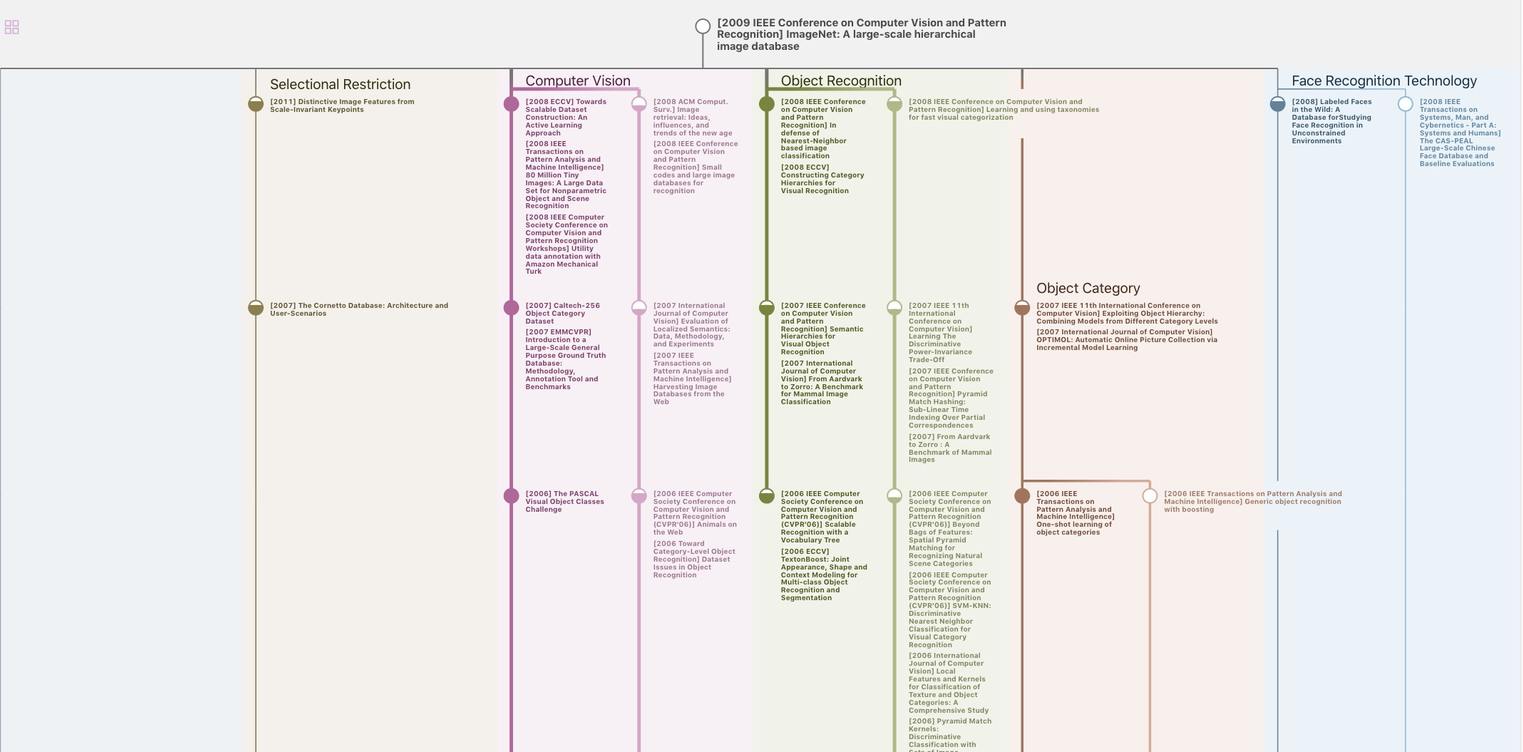
生成溯源树,研究论文发展脉络
Chat Paper
正在生成论文摘要