Improving Surface Melt Estimation over Antarctica
semanticscholar(2021)
摘要
Accurately estimating surface melt volume of the Antarctic Ice Sheet is challenging, and has hitherto relied on climate modelling, or on observations from satellite remote sensing. Each of these methods has its limitations, especially in regions with high surface melt. This study aims to demonstrate the potential of improving surface melt simulations ::: with :: a ::::::: regional :::::: climate :::::: model by deploying a deep learning model. A deep-learning-based framework has been developed to correct surface melt from the regional atmospheric climate model version 2.3p2 (RACMO2), using meteorological observations 5 from automatic weather stations (AWSs), and surface albedo from satellite imagery. The framework includes three steps: (1) training a deep multilayer perceptron (MLP) model using AWS observations; (2) correcting moderate resolution imaging spectroradiometer (MODIS) albedo observations, and (3) using these two to correct the RACMO2 surface melt simulations. Using observations from three AWSs at the Larsen B and C Ice Shelves, Antarctica, cross-validation shows a high accuracy (root mean square error = 0.95 mm w.e.per day, mean absolute error = 0.42 mm w.e.per day, and coefficient of determination 10 = 0.95). Moreover, the deep MLP model outperforms conventional machine learning models (e.g., random forest regression, XGBoost) and a shallow MLP model. When applying the trained deep MLP model over the entire Larsen Ice Shelf, the resulting , corrected RACMO2 surface melt shows a better correlation with the AWS observations for two out of three AWSs. However, for one location (AWS 18) the deep MLP model does not show improved agreement with AWS observations, likely due to the heterogeneous drivers of melt :::::: because :::::: surface :::: melt :: is :::::: driven :: to : a ::::: large ::::: extent :: by ::::: other :::::: factors ::::: (e.g., :: air ::::::::::: temperature, 15 ::::::::: topography, :::::::: katabatic :::::: wind) :::: than :::::: albedo ::::: alone : within the corresponding coarse resolution model pixels. Our study demonstrates the opportunity to improve surface melt simulations using deep learning combined with satellite albedo observations. On the other hand, more work is required to refine the method, especially for complicated and heterogeneous terrains.
更多查看译文
AI 理解论文
溯源树
样例
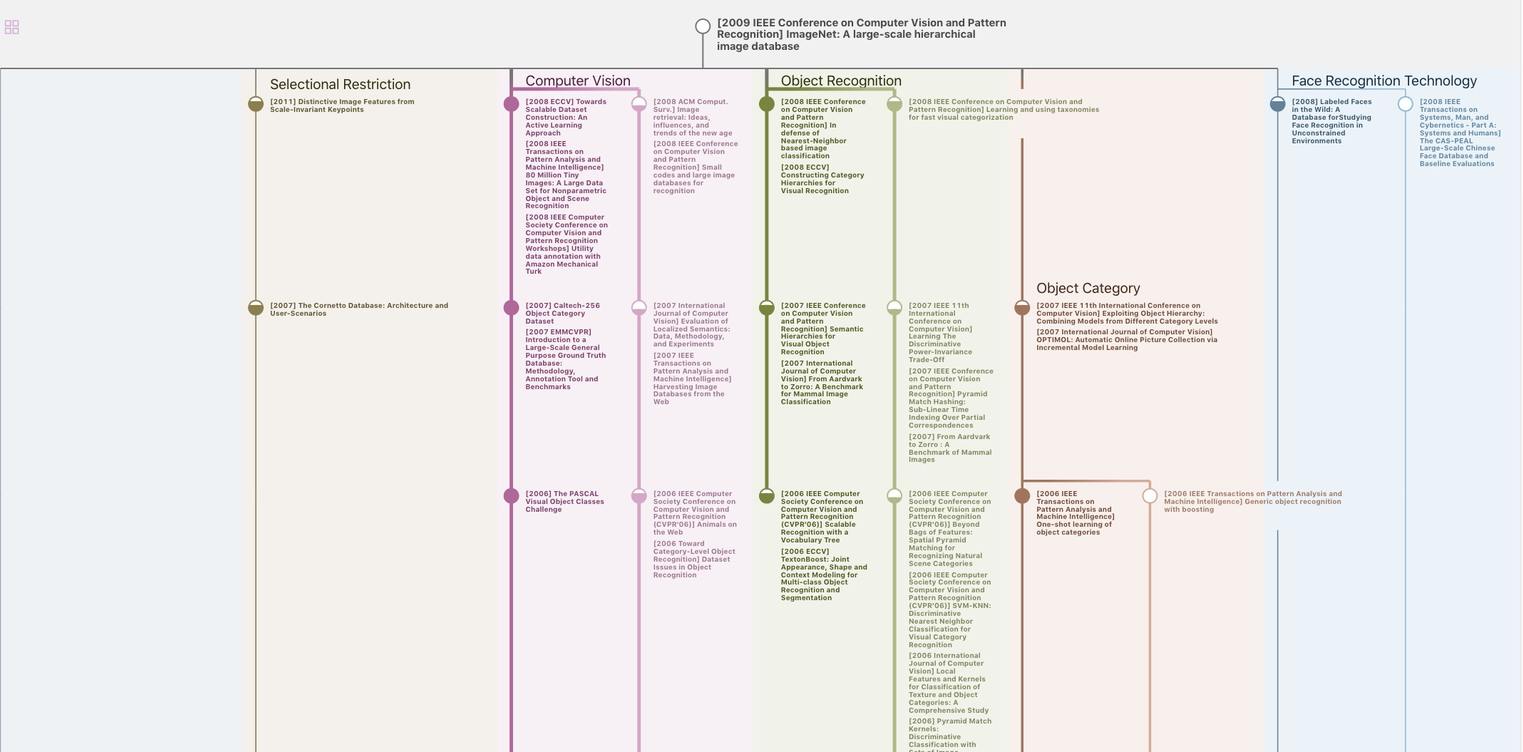
生成溯源树,研究论文发展脉络
Chat Paper
正在生成论文摘要