Parallel Transport Convolution: Deformable Convolutional Networks on Manifold-Structured Data
SIAM JOURNAL ON IMAGING SCIENCES(2022)
摘要
Convolution has played a prominent role in various applications in science and engineering for many years and has become a key operation in many neural networks. There has been a recent growth of interest in generalizing convolutions on three-dimensional surfaces, often represented as compact manifolds. However, existing approaches cannot preserve all the desirable properties of Euclidean convolutions, namely, compactly supported filters, directionality, and transferability across different manifolds. This paper develops a new generalization of the convolution operation, referred to as parallel transport convolution (PTC), on Riemannian manifolds and their discrete counterparts. PTC is designed based on parallel transportation that can translate information along a manifold and intrinsically preserve directionality. Furthermore, PTC allows for the construction of compactly supported filters and is also robust to manifold deformations. This enables us to perform waveletlike operations and to define convolutional neural networks on curved domains.
更多查看译文
关键词
shape analysis,convolution neural networks,parallel transport,manifold learning
AI 理解论文
溯源树
样例
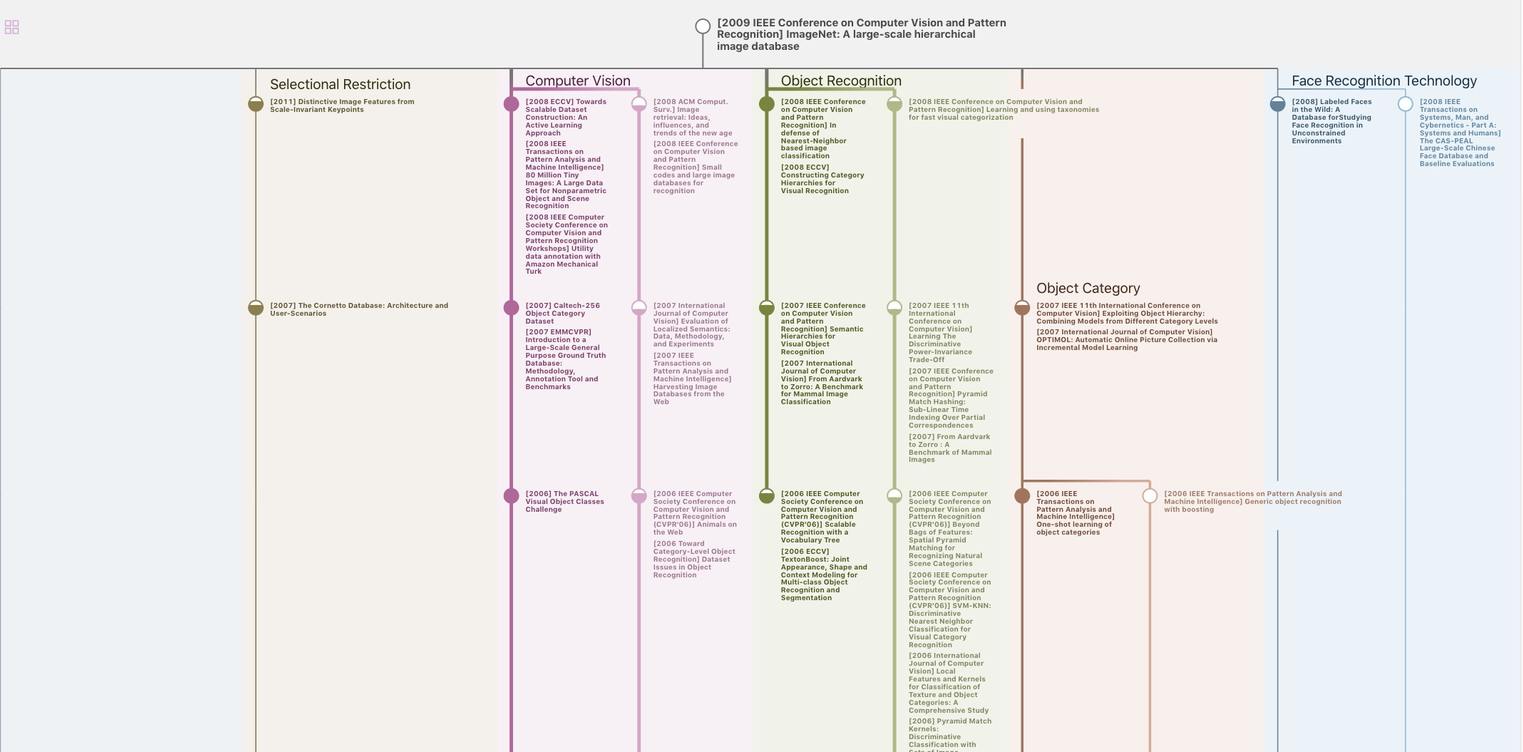
生成溯源树,研究论文发展脉络
Chat Paper
正在生成论文摘要