Structure-aware Protein Self-supervised Learning.
CoRR(2022)
摘要
Protein representation learning methods have shown great potential to yield useful representation for many downstream tasks, especially on protein classification. Moreover, a few recent studies have shown great promise in addressing in-sufficient labels of proteins with self-supervised learning methods. However, existing protein language models are usually pretrained on protein sequences without considering the important protein structural information. To this end, we propose a novel structure-aware protein self-supervised learning method to effectively capture structural information of proteins. In particular, a well-designed graph neural network (GNN) model is pretrained to preserve the protein structural information with self-supervised tasks from a pairwise residue distance perspective and a dihedral angle perspective, respectively. Furthermore, we propose to leverage the available protein language model pretrained on protein sequences to enhance the self-supervised learning. Specifically, we identify the relation between the sequential information in the protein language model and the structural information in the specially designed GNN model via a novel pseudo bi-level optimization scheme. Experiments on several supervised downstream tasks verify the effectiveness of our proposed method. Our code will be released upon acceptance.
更多查看译文
关键词
protein,learning,structure-aware,self-supervised
AI 理解论文
溯源树
样例
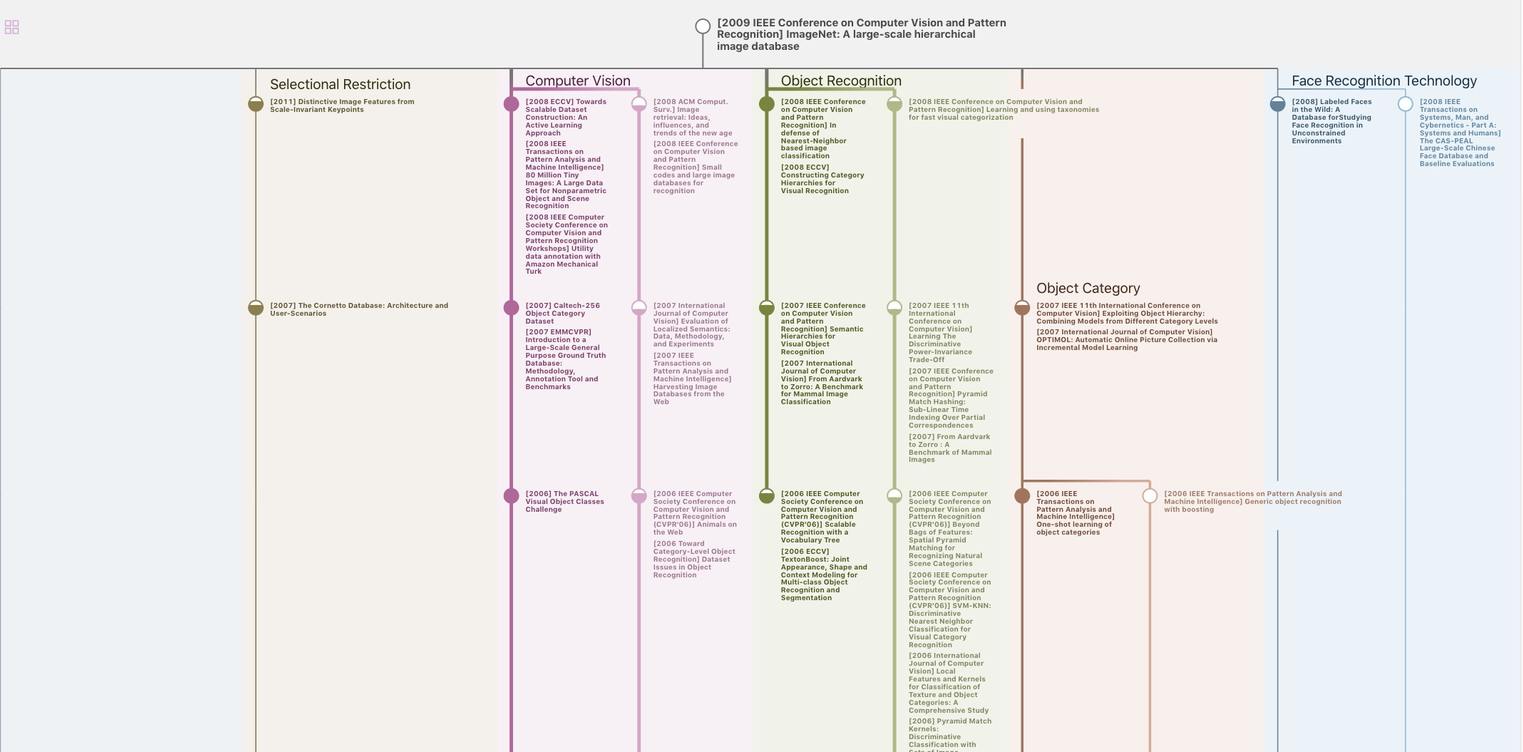
生成溯源树,研究论文发展脉络
Chat Paper
正在生成论文摘要