Decoupled R-CNN: Sensitivity-Specific Detector for Higher Accurate Localization
IEEE Transactions on Circuits and Systems for Video Technology(2022)
摘要
Object detection, as a fundamental problem in computer vision, has been widely used in many industrial applications, such as intelligent manufacturing and intelligent video surveillance. In this work, we find that classification and regression have different sensitivities to the object translation, from the investigation about the availability of highly overlapping proposals. More specifically, the regressor head has intrinsic characteristics of higher sensitivity to translation than the classifier. Based on it, we propose a decoupled sampling strategy for a deep detector, named Decoupled R-CNN, to decouple the proposals sampling for the two tasks, which induces two sensitivity-specific heads. Furthermore, we adopt the cascaded structure for the single regressor head of Decoupled R-CNN, which is an extremely simple but highly effective way of improving the performance of object detection. Extensive empirical analyses using real-world datasets demonstrate the value of the proposed method when compared with the state-of-the-art models. The reproducing code is available at
https://github.com/shouwangzhe134/Decoupled-R-CNN
.
更多查看译文
关键词
Object detection,R-CNN,two-stage detection,decoupled sampling strategy,decoupled R-CNN
AI 理解论文
溯源树
样例
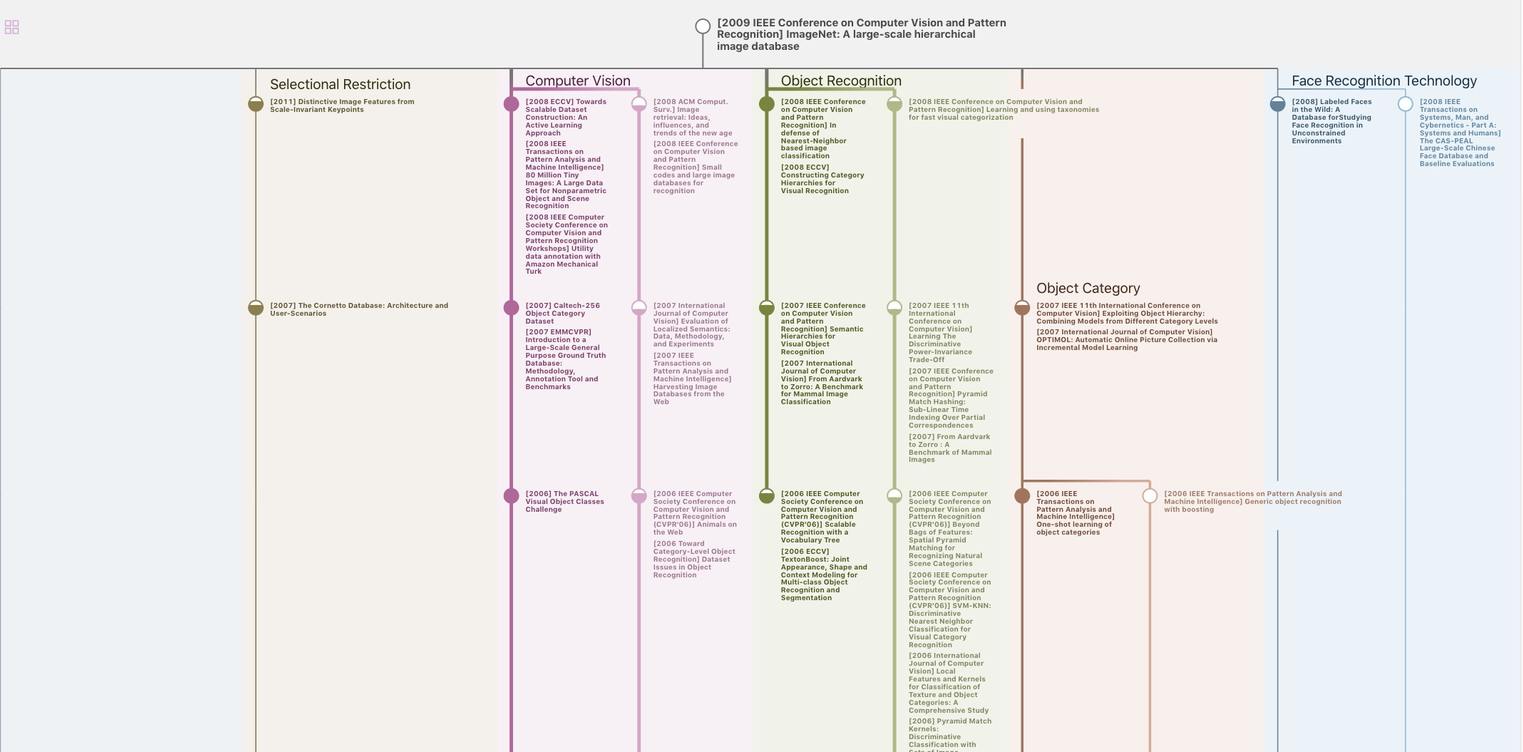
生成溯源树,研究论文发展脉络
Chat Paper
正在生成论文摘要