Addressing Network Bottlenecks with Divide-and-Shuffle Synchronization for Distributed DNN Training
IEEE Conference on Computer Communications (INFOCOM)(2022)
摘要
Bulk synchronous parallel (BSP) is the de-facto paradigm for distributed DNN training in today's production clusters. However, due to the global synchronization nature, its performance can be significantly influenced by network bottlenecks caused by either static topology heterogeneity or dynamic bandwidth contentions. Existing solutions, either system-level optimizations strengthening BSP (e.g., Ring or Hierarchical All-reduce) or algorithmic optimizations replacing BSP (e.g., ASP or SSP, which relax the global barriers), do not completely solve the problem, as they may still suffer from communication inefficiency or risk convergence inaccuracy. In this paper, we present a novel divide-and-shuffle synchronization (DS-Sync) to realize communication efficiency without sacrificing convergence accuracy for distributed DNN training. At its heart, by taking into account the network bottlenecks, DS-Sync improves communication efficiency by dividing workers into non-overlap groups to synchronize independently in a bottleneck-free manner. Meanwhile, it maintains convergence accuracy by iteratively shuffling workers among different groups to ensure a global consensus. We theoretically prove that DS-Sync converges properly in non-convex and smooth conditions like DNN. We further implement DS-Sync and integrate it with PyTorch, and our testbed experiments show that DS-Sync can achieve up to 94% improvements on the end-to-end training time with existing solutions while maintaining the same accuracy.
更多查看译文
关键词
distributed DNN training, synchronization, communication efficiency, convergence accuracy
AI 理解论文
溯源树
样例
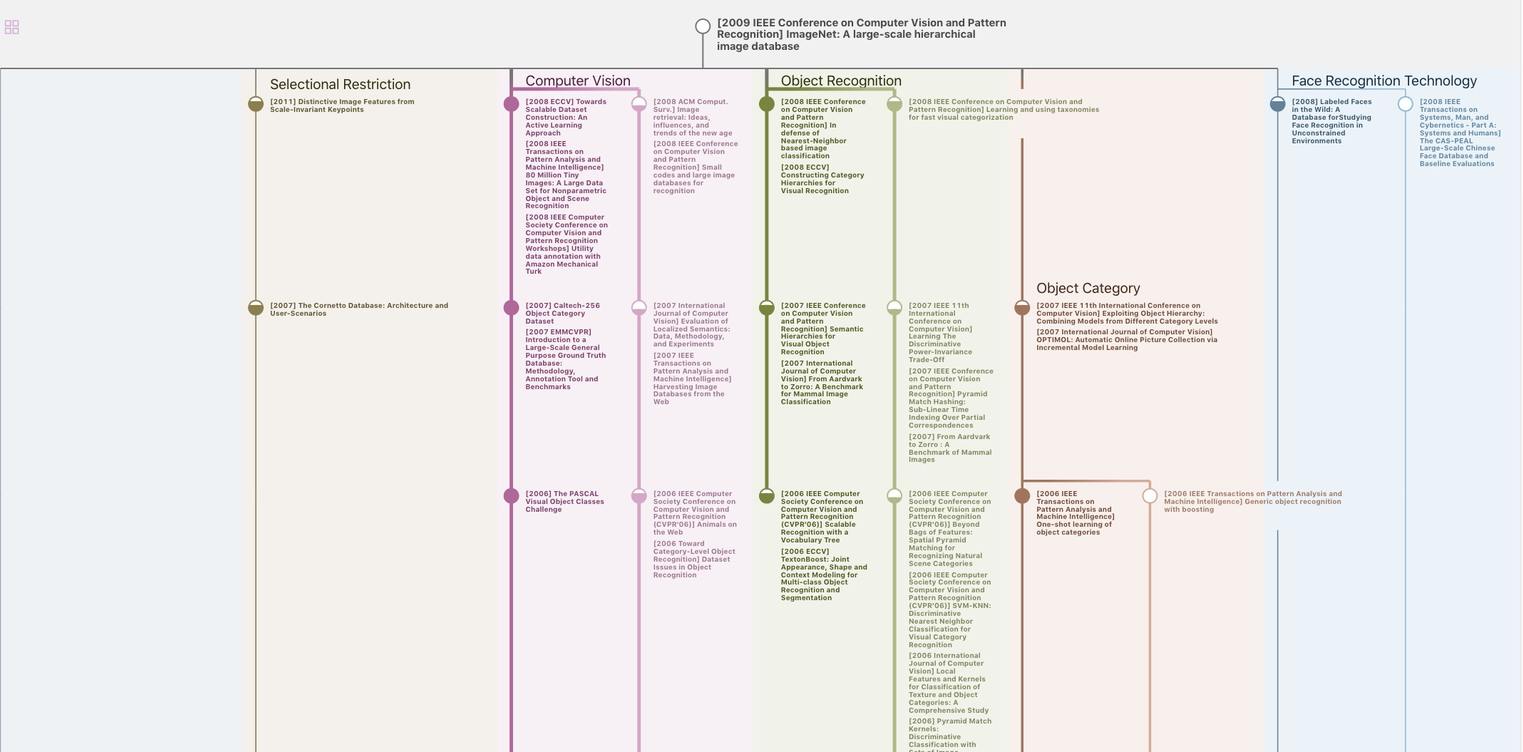
生成溯源树,研究论文发展脉络
Chat Paper
正在生成论文摘要