Predictive Model Using a Machine Learning Approach for Enhancing the Retention Rate of Students At-Risk
INTERNATIONAL JOURNAL ON SEMANTIC WEB AND INFORMATION SYSTEMS(2022)
摘要
Student retention is a widely recognized challenge in the educational community to assist the institutes in the formation of appropriate and effective pedagogical interventions. This study intends to predict the students at risk of low performance during an on-going course, those at risk of graduating late than the tentative timeline. and predicts the capacity of students in a campus. The data constitutes of demographics, learning, academic, and education-related attributes that are suitable to deploy various machine learning algorithms for the prediction of at-risk students. For class balancing, synthetic minority over sampling technique is also applied to eliminate the imbalance in the academic award-gap performances and late/timely graduates. Results reveal the effectiveness of the deployed techniques with long short-term memory (LSTM) outperforming other models for early prediction of at-risk students. The main contribution of this work is a machine learning approach capable of enhancing the academic decision-making related to student performance.
更多查看译文
关键词
Academic Performance Prediction,Balancing Student Data,Classification,Early Student Prediction,LSTM,Machine Learning,Student at Risk
AI 理解论文
溯源树
样例
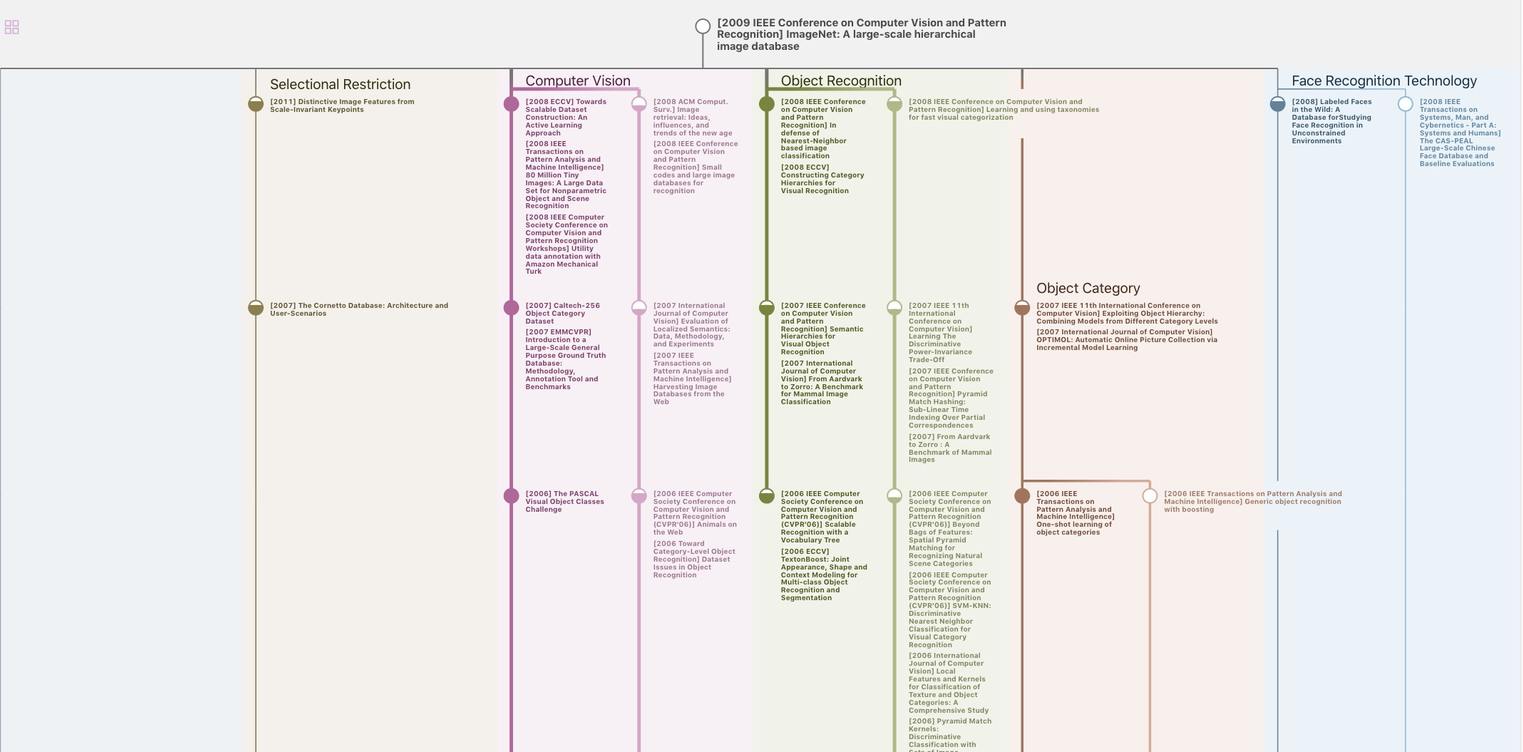
生成溯源树,研究论文发展脉络
Chat Paper
正在生成论文摘要