IFA-EO: An improved firefly algorithm hybridized with extremal optimization for continuous unconstrained optimization problems
SOFT COMPUTING(2022)
摘要
As one of the evolutionary algorithms, firefly algorithm (FA) has been widely used to solve various complex optimization problems. However, FA has significant drawbacks in slow convergence rate and is easily trapped into local optimum. To tackle these defects, this paper proposes an improved FA combined with extremal optimization (EO), named IFA-EO, where three strategies are incorporated. First, to balance the tradeoff between exploration ability and exploitation ability, we adopt a new attraction model for FA operation, which combines the full attraction model and the single attraction model through the probability choice strategy. In the single attraction model, small probability accepts the worse solution to improve the diversity of the offspring. Second, the adaptive step size is proposed based on the number of iterations to dynamically adjust the attention to the exploration model or exploitation model. Third, we combine an EO algorithm with powerful ability in local-search into FA. Experiments are tested on two group popular benchmarks including complex unimodal and multimodal functions. Our experimental results demonstrate that the proposed IFA-EO algorithm can deal with various complex optimization problems and has similar or better performance than the other eight FA variants, three EO-based algorithms, and one advanced differential evolution variant in terms of accuracy and statistical results.
更多查看译文
关键词
Firefly algorithm, extremal optimization, Probability choice strategy, Adaptive step size, Continuous optimization problems
AI 理解论文
溯源树
样例
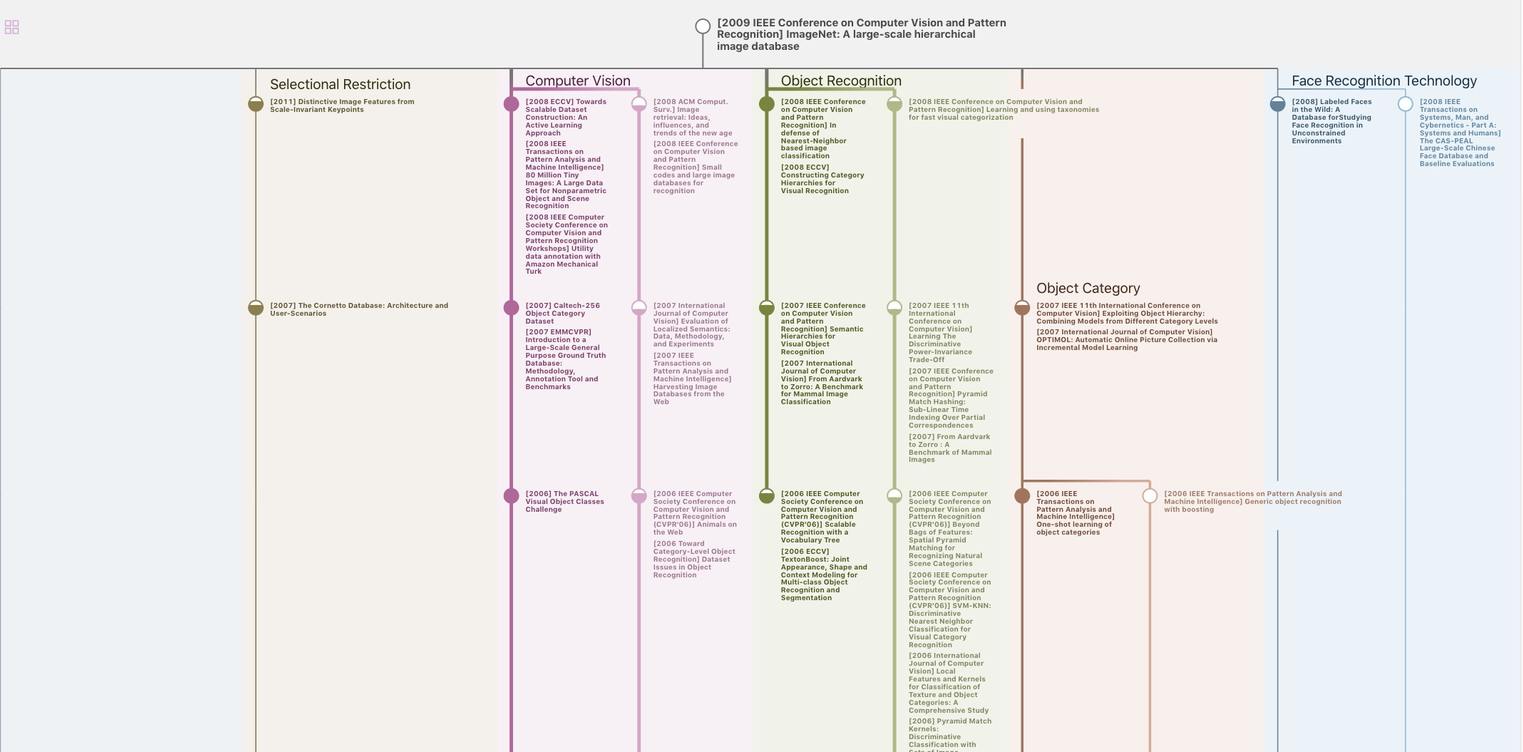
生成溯源树,研究论文发展脉络
Chat Paper
正在生成论文摘要