Deep learning achieves radiologist-level performance at segmenting breast cancers on MRI
semanticscholar(2022)
摘要
Purpose: The purpose was to develop a deep network architecture that achieves fully-automated radiologist-level segmentation of cancers in breast MRI. Materials and Methods: We leveraged 38,229 exams (64,063 individual breast scans) collected retrospectively from women aged 12-94 (mean age 54) who presented between 2002 and 2014 at a single clinical site. For network training, we selected 2,555 breast cancers which were segmented in 2D by radiologists, as well as 60,108 benign breasts, which served as examples of non-cancerous tissue during training. For testing, an additional 250 breast cancers were segmented independently in 2D by four radiologists. We selected among several 3D deep convolutional neural network architectures, input modalities and harmonization methods. The outcome measure was the Dice score for 2D segmentation, and was compared between the network and radiologists using the Wilcoxon signed-rank test and the TOST procedure. Results: The best-performing network on the training set was a 3D U-Net with contrast enhancement dynamic as input and with intensity normalized for each exam. In the test set the median Dice score of this network was 0.77+/-0.26. The performance of the network was equivalent to that of the radiologists (TOST procedure with radiologist performance of 0.69-0.84 as equivalence bounds: p < .001 and p < .001, respectively; N = 250) and compares favorably with published state of the art (0.6-0.77). Conclusion: When trained on a sufficiently large dataset, a carefully designed 3D U-Net performs as well as fellowship-trained radiologists in detailed 2D segmentation of breast cancers in routine clinical MRI.
更多查看译文
AI 理解论文
溯源树
样例
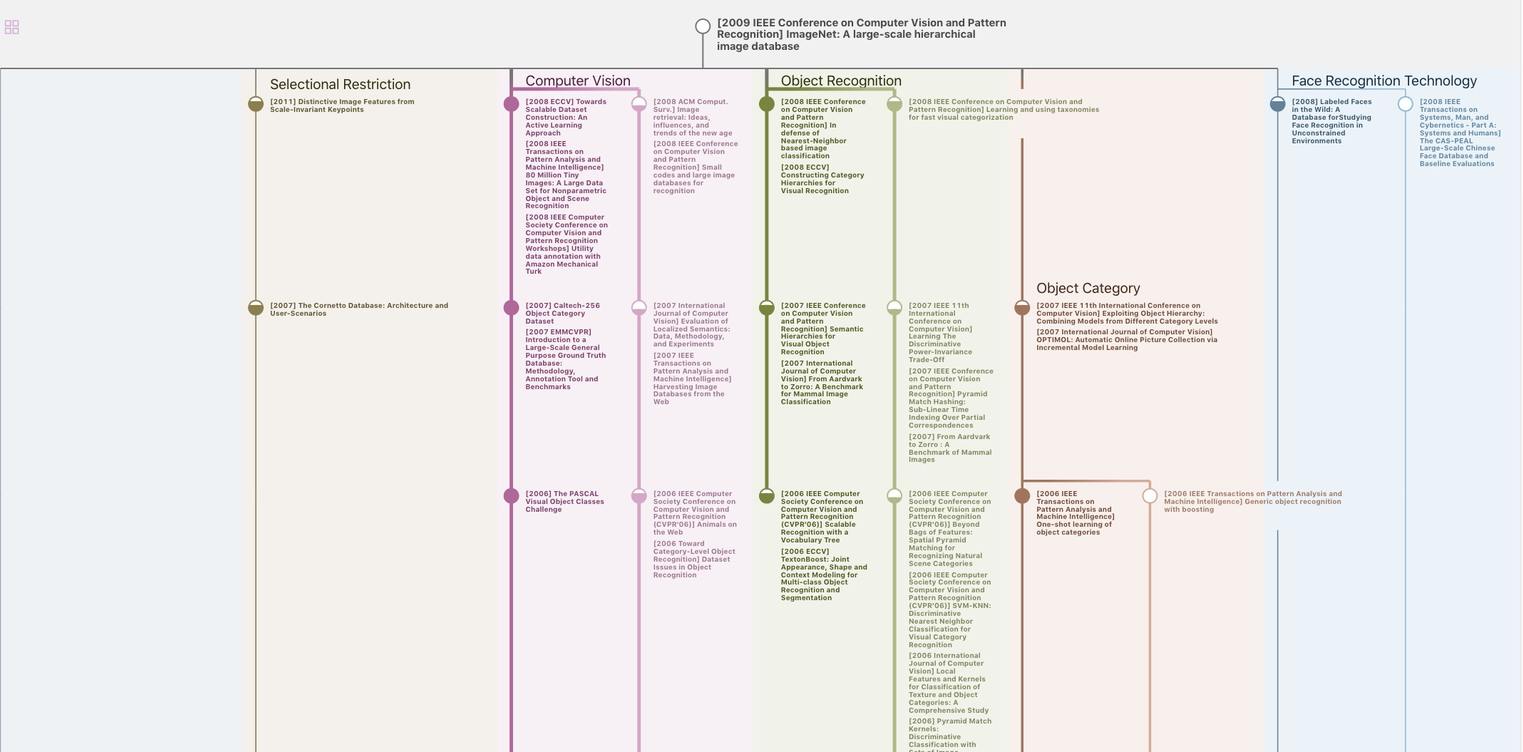
生成溯源树,研究论文发展脉络
Chat Paper
正在生成论文摘要