Energy trading strategy for storage-based renewable power plants
ENERGY(2022)
摘要
Despite the continuous growth and the widespread support of renewable energy sources, solar and wind power plants pose new challenges for Transmission System Operators and Distribution System Opera-tors. Their uncontrollability limits their applicability; therefore, to encourage their further growth, fundamental modifications are needed. The research presented in this paper focuses on the predictive control of storage-based renewable power plants, and suggests a new model for profit optimization. Profit optimization is based on electricity price prediction and effective trading strategies that match the projected electricity prices. For the electricity price prediction, a recurrent Long Short-Term Memory neural network was developed and fine-tuned. For the optimization of the electricity trading, two trading strategies, namely an adaptive gradient-descent method and a differential evolution method were developed. Both optimization techniques were tested on mathematical models of most commer-cially available hybrid inverter systems and one year of historical data of electricity prices. As a result, a novel model predictive control workflow and sizing guide is proposed, which may significantly increase the profit generated by the system.(c) 2022 Elsevier Ltd. All rights reserved.
更多查看译文
关键词
Electricity price prediction,LSTM network,Energy trading,Gradient descent optimization,Differential evolution optimization
AI 理解论文
溯源树
样例
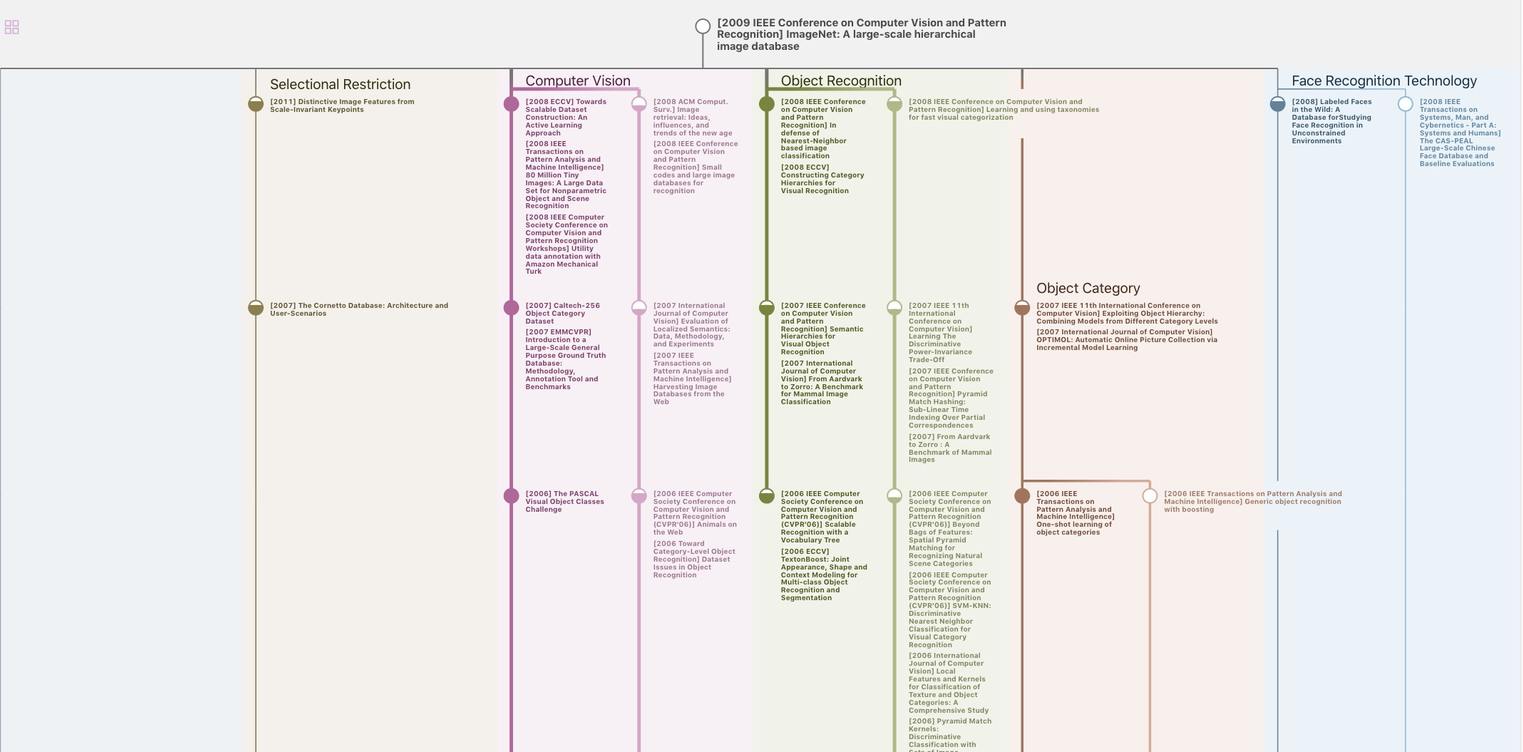
生成溯源树,研究论文发展脉络
Chat Paper
正在生成论文摘要