Fully Automatic Quantitative Measurement of F-18-FDG PET/CT in Thymic Epithelial Tumors Using a Convolutional Neural Network
Clinical nuclear medicine(2022)
摘要
Objectives The aim of this study was to develop a deep learning (DL)-based segmentation algorithm for automatic measurement of metabolic parameters of F-18-FDG PET/CT in thymic epithelial tumors (TETs), comparable performance to manual volumes of interest. Patients and Methods A total of 186 consecutive patients with resectable TETs and preoperative F-18-FDG PET/CT were retrospectively enrolled (145 thymomas, 41 thymic carcinomas). A quasi-3D U-net architecture was trained to resemble ground-truth volumes of interest. Segmentation performance was assessed using the Dice similarity coefficient. Agreements between manual and DL-based automated extraction of SUVmax, metabolic tumor volume (MTV), total lesion glycolysis (TLG), and 63 radiomics features were evaluated via concordance correlation coefficients (CCCs) and linear regression slopes. Diagnostic and prognostic values were compared in terms of area under the receiver operating characteristics curve (AUC) for thymic carcinoma and hazards ratios (HRs) for freedom from recurrence. Results The mean Dice similarity coefficient was 0.83 +/- 0.34. Automatically measured SUVmax (slope, 0.97; CCC, 0.92), MTV (slope, 0.94; CCC, 0.96), and TLG (slope, 0.96; CCC, 0.96) were in good agreement with manual measurements. The mean CCC and slopes were 0.88 +/- 0.06 and 0.89 +/- 0.05, respectively, for the radiomics parameters. Automatically measured SUVmax, MTV, and TLG showed good diagnostic accuracy for thymic carcinoma (AUCs: SUVmax, 0.95; MTV, 0.85; TLG, 0.87) and significant prognostic value (HRs: SUVmax, 1.31 [95% confidence interval, 1.16-1.48]; MTV, 2.11 [1.09-4.06]; TLG, 1.90 [1.12-3.23]). No significant differences in the AUCs or HRs were found between automatic and manual measurements for any of the metabolic parameters. Conclusions Our DL-based model provides comparable segmentation performance and metabolic parameter values to manual measurements in TETs.
更多查看译文
关键词
thymoma, F-18-FDG, PET, deep learning, image processing
AI 理解论文
溯源树
样例
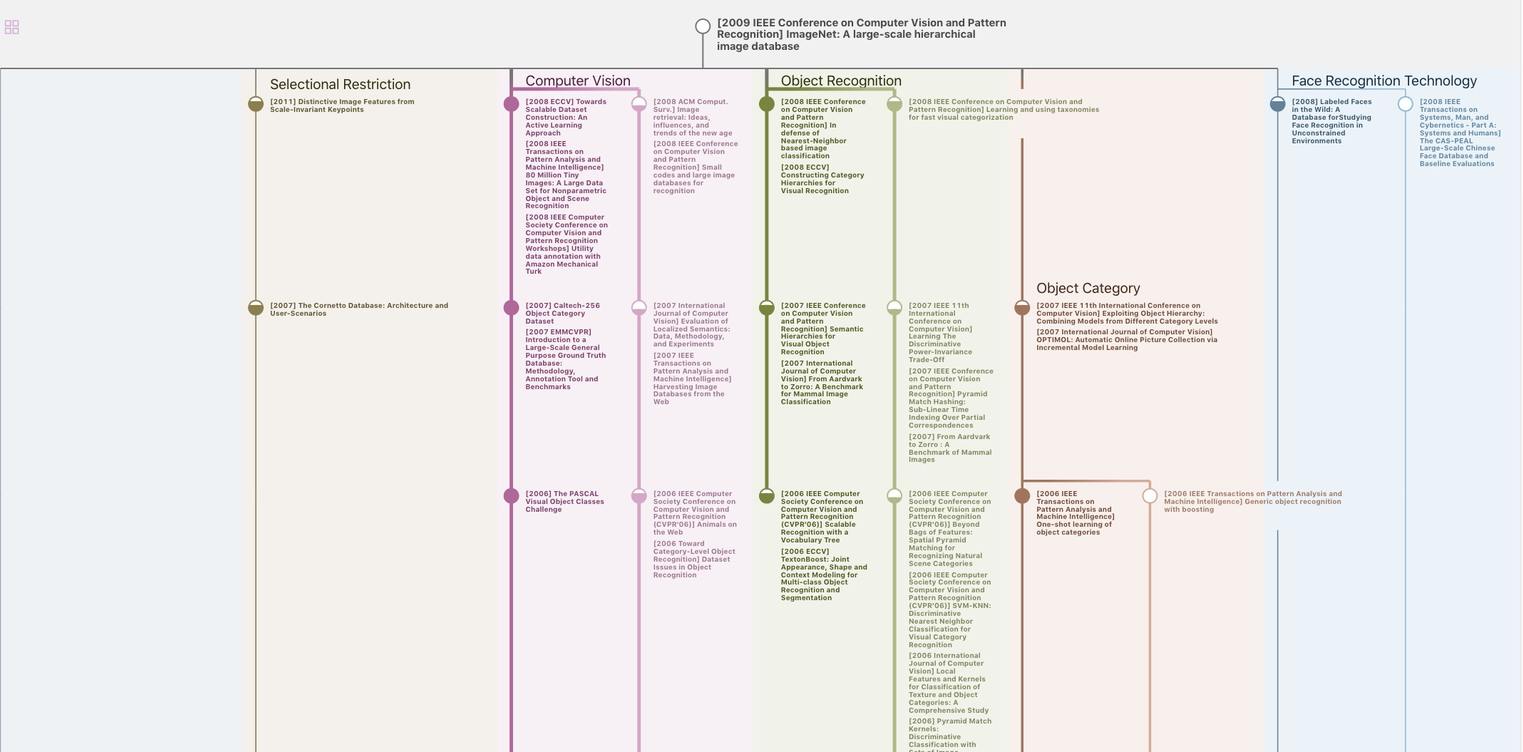
生成溯源树,研究论文发展脉络
Chat Paper
正在生成论文摘要