Identifying the Origins of Extreme Rainfall in South Africa Using Storm Trajectory Analysis and Unsupervised Machine Learning Techniques
semanticscholar(2022)
摘要
Extreme rainfall events can have a detrimental impact on both human life and infrastructure, regularly ranked as the number one risks global risks to infrastructure. Climate change is likely to exacerbate the magnitude of extreme rainfall events and developing an understanding of the underlying causes will be key to improve infrastructure resilience and the prediction of such events in the future. This study has utilised National Oceanic and Atmospheric Administration (NOAA) NCEP/NCAR Reanalysis 1 project meteorological data and the HYSPLIT model to extract the air parcel trajectories for selected historical extreme rainfall events in South Africa. The k -means unsupervised machine learning algorithm has been used to cluster the resulting trajectories and from this the spatial origin of moisture for each of the rainfall events has been determined. It has been demonstrated that rainfall events on the east coast with moisture originating from the Indian Ocean have distinctly larger average maximum daily rainfall magnitudes (279mm) compared to those that occur on the west coast with Atlantic Ocean influences (149mm) and those events occurring in the central plateau (150mm) where moisture has been continentally recirculated. Further to this, this study has suggested new metrics by which the HYSPLIT trajectories may be assessed and demonstrated the applicability of trajectory clustering in a region not previously studied. This insight may in future facilitate improved early warning systems based on monitoring of atmospheric systems and an understanding of rainfall magnitudes and origins can be used to improve the prediction of design floods for infrastructure design.
更多查看译文
AI 理解论文
溯源树
样例
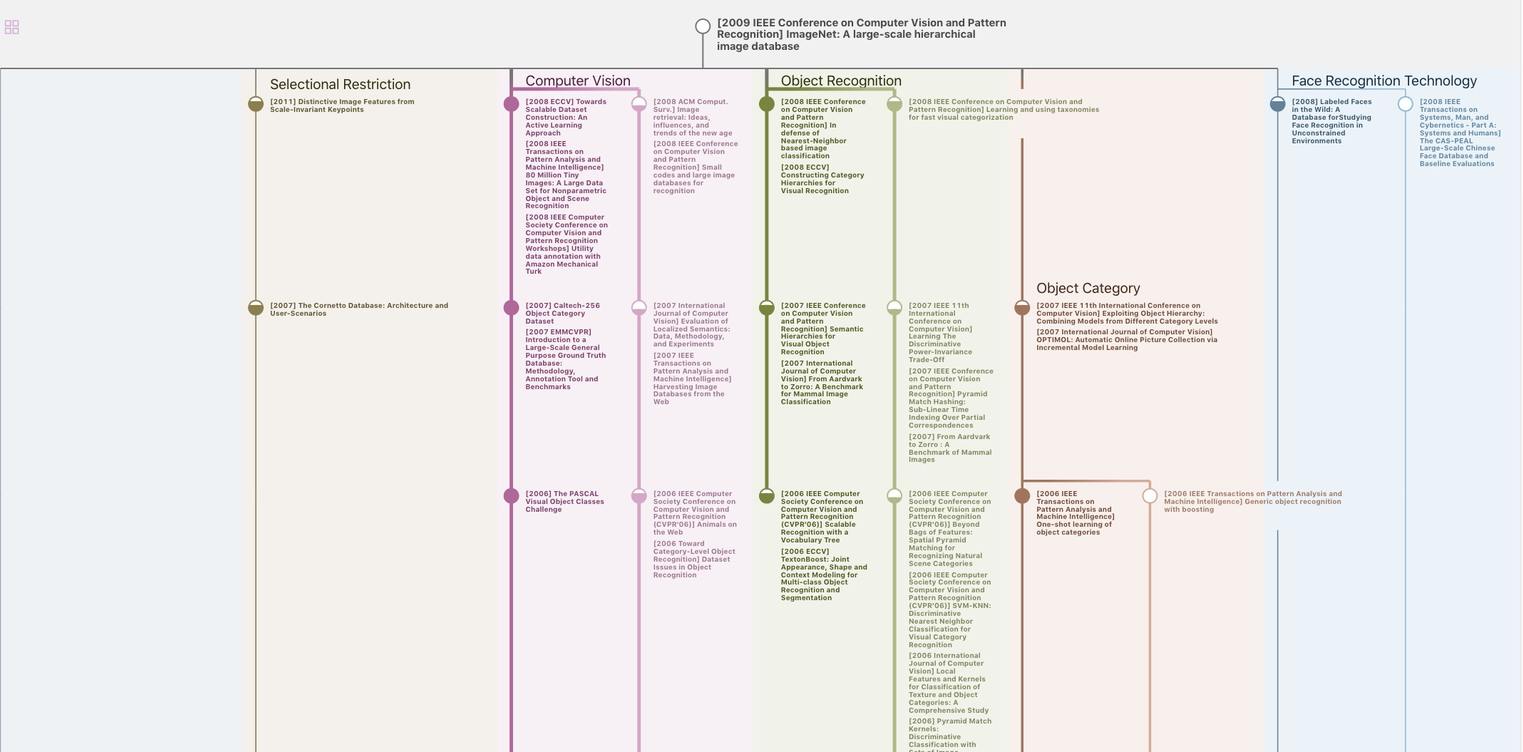
生成溯源树,研究论文发展脉络
Chat Paper
正在生成论文摘要