Out-of-distribution Generalization via Partial Feature Decorrelation
semanticscholar(2020)
摘要
Most deep-learning-based image classification methods assume that all samples are generated under an independent and identically distributed (IID) setting. However, out-ofdistribution (OOD) generalization is more common in practice, which means an agnostic context distribution shift between training and testing environments. To address this problem, we present a novel Partial Feature Decorrelation Learning (PFDL) algorithm, which jointly optimizes a feature decomposition network and the target image classification model. The feature decomposition network decomposes feature embeddings into the independent and the correlated parts such that the correlations between features will be highlighted. Then, the correlated features help learn a stable feature representation by decorrelating the highlighted correlations while optimizing the image classification model. We verify the correlation modeling ability of the feature decomposition network on a synthetic dataset. The experiments on real-world datasets demonstrate that our method can improve the backbone model’s accuracy on OOD image classification datasets.
更多查看译文
关键词
partial feature decorrelation,out-of-distribution
AI 理解论文
溯源树
样例
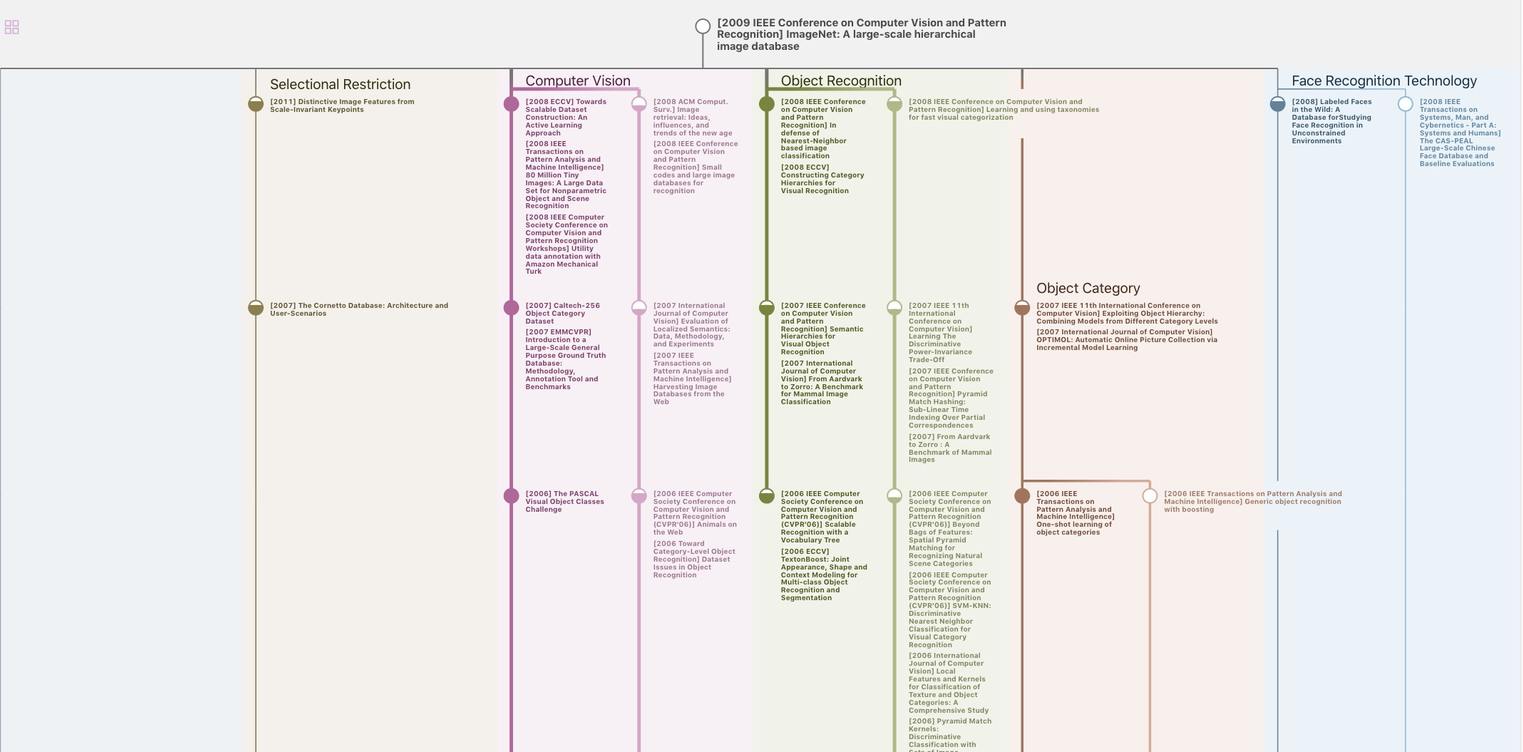
生成溯源树,研究论文发展脉络
Chat Paper
正在生成论文摘要