Deep-learning based end-to-end system for text reading in the wild
Multimedia Tools and Applications(2022)
摘要
Scene text reading includes both scene text detection and recognition tasks. These tasks face several challenges including text pattern variability, background complexity and scene image quality. Efficient state-of-the-art scene text reading systems are deep learning based methods. They have shown substantial performance at the cost of a complex architecture with high computational time. This paper introduces a deep learning based end-to-end system to improve text detection and recognition efficiency under a unified framework with a low computational cost. In comparison with existing systems, the main characteristics of the proposed system are four. First, we propose a refined patch based selective search to localize all text instances in a scene image. Second, we propose a unified trainable framework taking scene text detection and recognition. This framework is built using a single yet much smaller Convolutional Neural Networks (CNN). Third, we propose a character segmentation free based approach with no scale normalization. Fourth, we use a spanning tree-based algorithm for character grouping to enhance the word recognition process. The comparative study performed on standard benchmarks, including ICDAR 2003, ICDAR 2013, ICDAR 2015, and SVT datasets, demonstrates that the proposed scene text reading system achieves very promising results mainly in both reduced and generic lexicon-based recognition.
更多查看译文
关键词
Scene text reading, Convolutional Neural Networks, Selective search, End-to-end system, Characters sequence detection and recognition
AI 理解论文
溯源树
样例
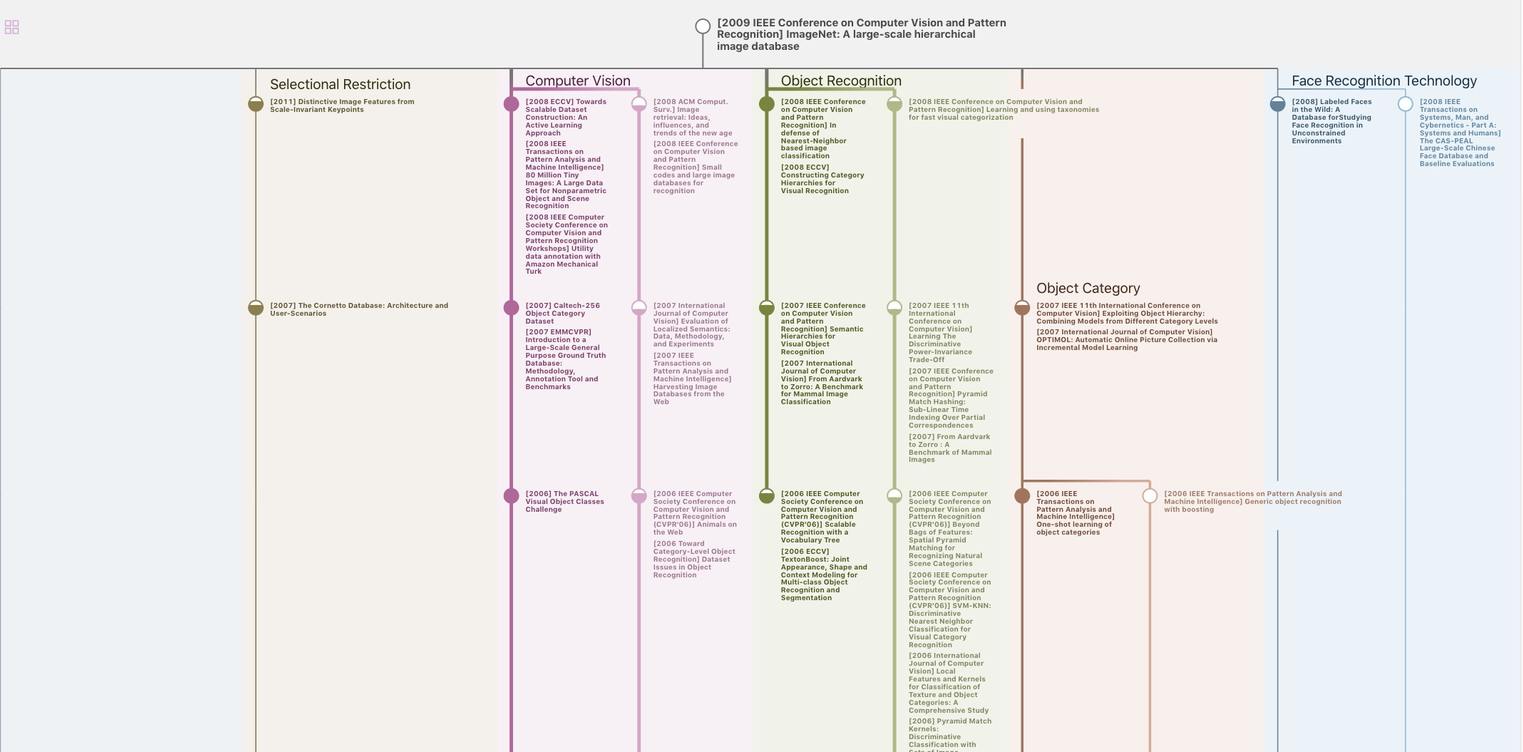
生成溯源树,研究论文发展脉络
Chat Paper
正在生成论文摘要