Learning Explainable Policy For Playing Blackjack Using Deep Reinforcement Learning (Reinforcement Learning)
semanticscholar(2021)
摘要
Our attempt was to learn an optimal Blackjack policy using a Deep Reinforcement Learning model that has full visibility of the state space. We implemented a game simulator and various other models to baseline against. We showed that the Deep Reinforcement Learning model could learn card counting and exceed standard card counting methodologies and achieved a positive average reward (e.g, "beat the house"). We showed the model was explainable and could be used to derive a human-understandable strategy for Blackjack that exceeds current best practices.
更多查看译文
AI 理解论文
溯源树
样例
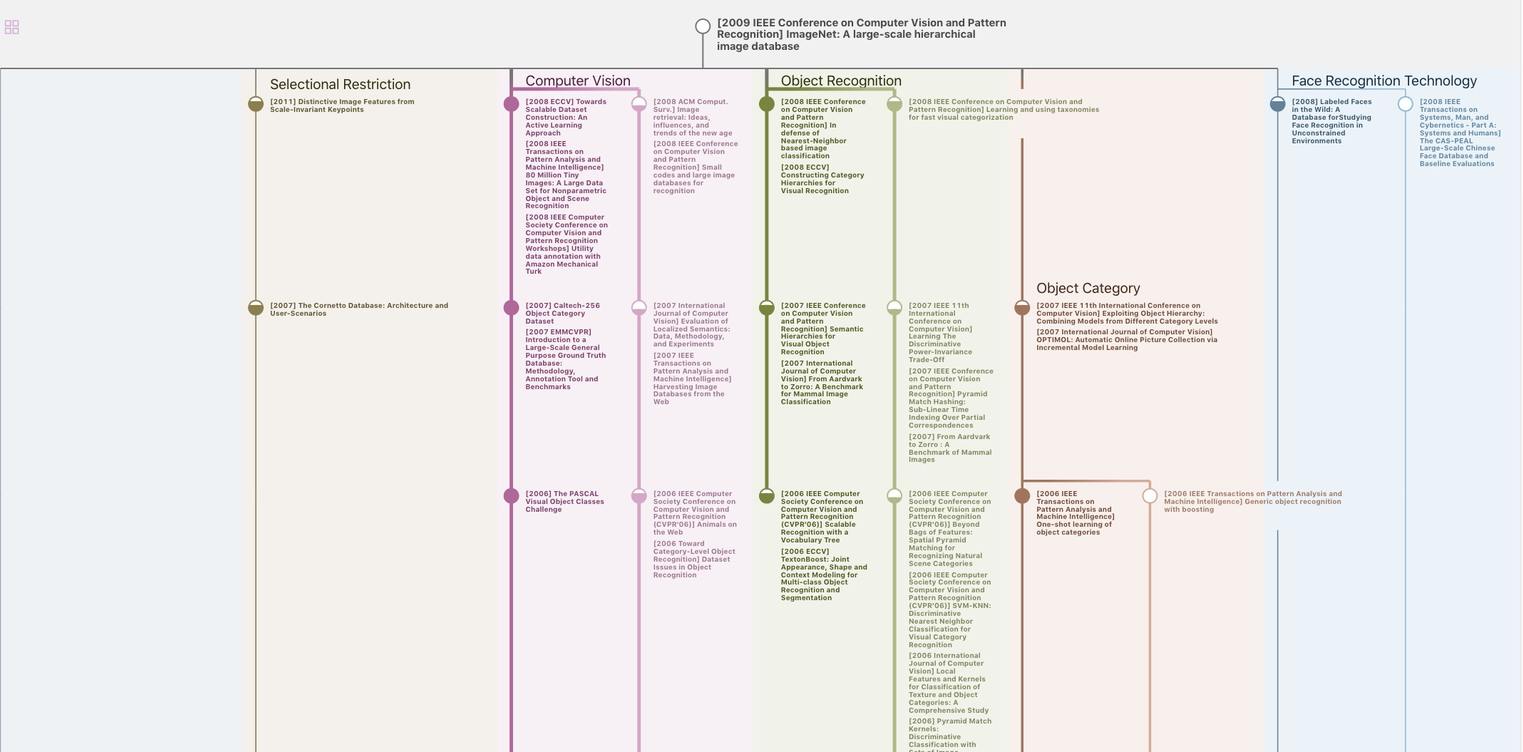
生成溯源树,研究论文发展脉络
Chat Paper
正在生成论文摘要