Attentive Deep Stitching and Quality Assessment for 360◦ Omnidirectional Images
semanticscholar(2019)
摘要
360◦ omnidirectional images are very helpful in creat4 ing immersive multimedia contents, which enables a huge demand 5 in their efficient generation and effective assessment. In this paper, 6 we leverage an attentive idea to meet this demand by addressing two 7 concerns: how to generate a good omnidirectional image in a fast 8 and robust way and what is a good omnidirectional image for hu9 man. To this end, we propose an attentive deep stitching approach 10 to facilitate the efficient generation of omnidirectional images, 11 which is composed of two modules. The low-resolution deformation 12 module aims to learn the deformation rules from dual-fisheye to om13 nidirectional images with joint implicit and explicit attention mech14 anisms, while the high-resolution recurrence module enhances the 15 resolution of stitching results with the high-resolution guidance in a 16 recurrent manner. In this way, the stitching approach can efficiently 17 generate high-resolution omnidirectional images that are highly 18 consistent with human immersive experiences. Beyond the efficient 19 generation, we further present an attention-driven omnidirectional 20 image quality assessment (IQA) method which uses joint evaluation 21 with both global and local metrics. Especially, the local metric 22 mainly focuses on the stitching region and attention region that 23 mostly affect the Mean Opinion Score (MOS), leading to a consis24 tent evaluation of human perception. To verify the effectiveness of 25 our proposed assessment and stitching approaches, we construct a 26 hybrid benchmark evaluation with 7 stitching models and 8 IQA 27 metrics. Qualitative and quantitative experiments show our stitch28 ing approach generate preferable results with the state-of-the-art 29 models at a 6× faster speed and the proposed quality assessment 30 approach surpasses other methods by a large margin and is highly 31 consistent with human subjective evaluations. Q1 32
更多查看译文
AI 理解论文
溯源树
样例
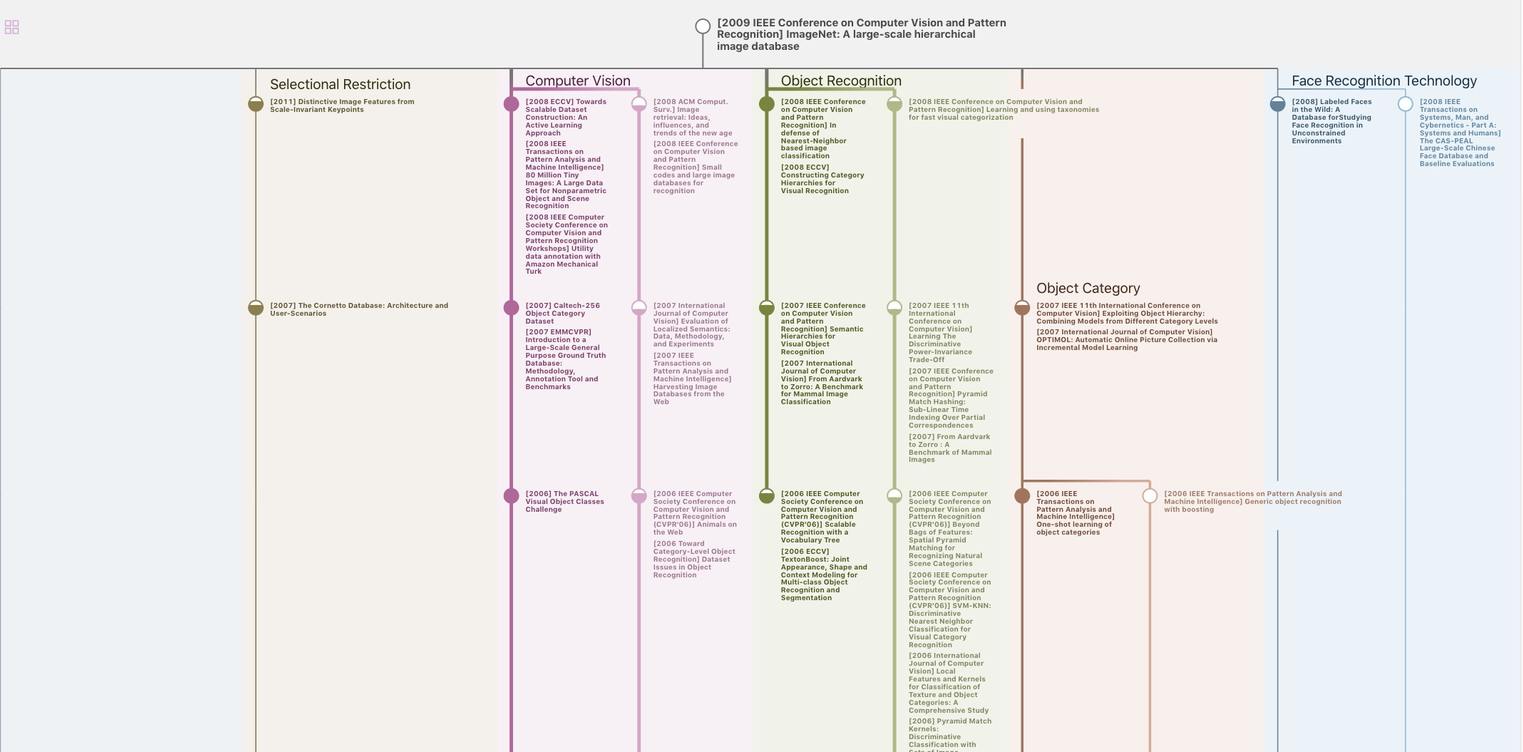
生成溯源树,研究论文发展脉络
Chat Paper
正在生成论文摘要