The effect of chemical representation on active machine learning towards closed-loop optimization
REACTION CHEMISTRY & ENGINEERING(2022)
摘要
Multivariate chemical reaction optimization involving catalytic systems is a non-trivial task due to the high number of tuneable parameters and discrete choices. Active machine learning (ML) represents a powerful strategy for automating reaction optimization. However, the translation of chemical reaction conditions into a machine-readable format requires the identification of highly informative features which accurately capture the factors which determine reaction success. Herein, we compare the efficacy of different calculated chemical descriptors for a high throughput experimentation generated dataset to determine the impact on a supervised ML model when predicting reaction yield. Then, the effect of featurization and size of the initial dataset within a closed-loop reaction optimization was examined. Finally, the balance between descriptor complexity and dataset size was considered. Ultimately, tailored descriptors did not outperform simple generic representations, however, a larger initial dataset accelerated reaction optimization.
更多查看译文
AI 理解论文
溯源树
样例
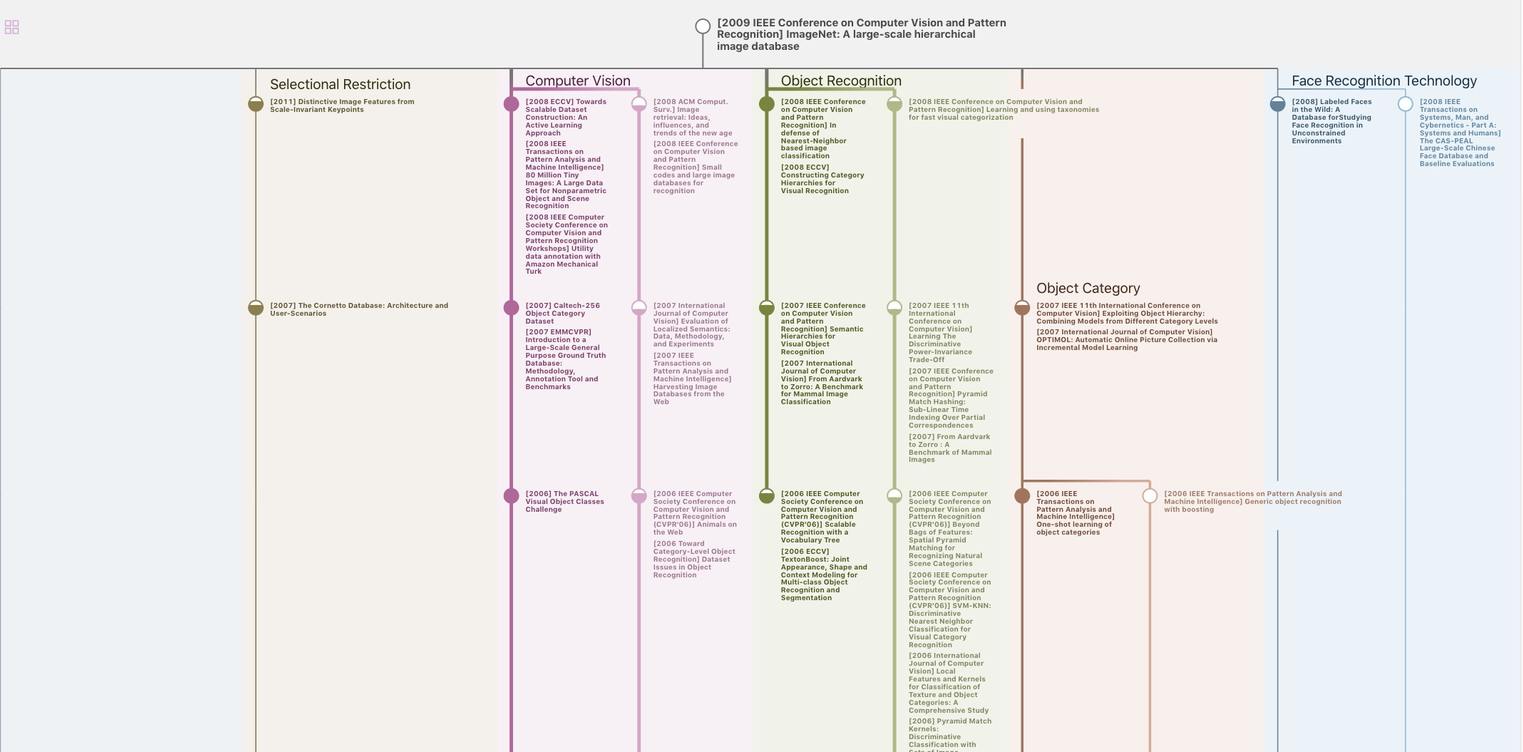
生成溯源树,研究论文发展脉络
Chat Paper
正在生成论文摘要