Forward Compatible Training for Large-Scale Embedding Retrieval Systems.
2022 IEEE/CVF Conference on Computer Vision and Pattern Recognition (CVPR)(2022)
摘要
In visual retrieval systems, updating the embedding model requires recomputing features for every piece of data. This expensive process is referred to as backfilling. Re-cently, the idea of backward compatible training (BCT) was proposed. To avoid the cost of backfilling, BCT modifies training of the new model to make its representations com-patible with those of the old model. However, BCT can sig-nificantly hinder the performance of the new model. In this work, we propose a new learning paradigm for representation learning: forward compatible training (FCT). In Fct, when the old model is trained, we also prepare for a future unknown version of the model. We propose learning side- information, an auxiliary feature for each sample which fa-cilitates future updates of the model. To develop a powerful and flexible frameworkfor model compatibility, we combine side-information with a forward transformation from old to newembeddings. Training of the new model is not modified, hence, its accuracy is not degraded. We demonstrate sig-nificant retrieval accuracy improvement compared to BCT for various datasets: ImageNet-1k (+18.1%), Places-365 (+5.4%), and VGG-Face2 (+8.3%). Fctobtains model compatibility when the new and old models are trained across different datasets, losses, and architectures. 1 1 Code available at https://github.com/apple/ml-fct.
更多查看译文
关键词
forward,large-scale large-scale
AI 理解论文
溯源树
样例
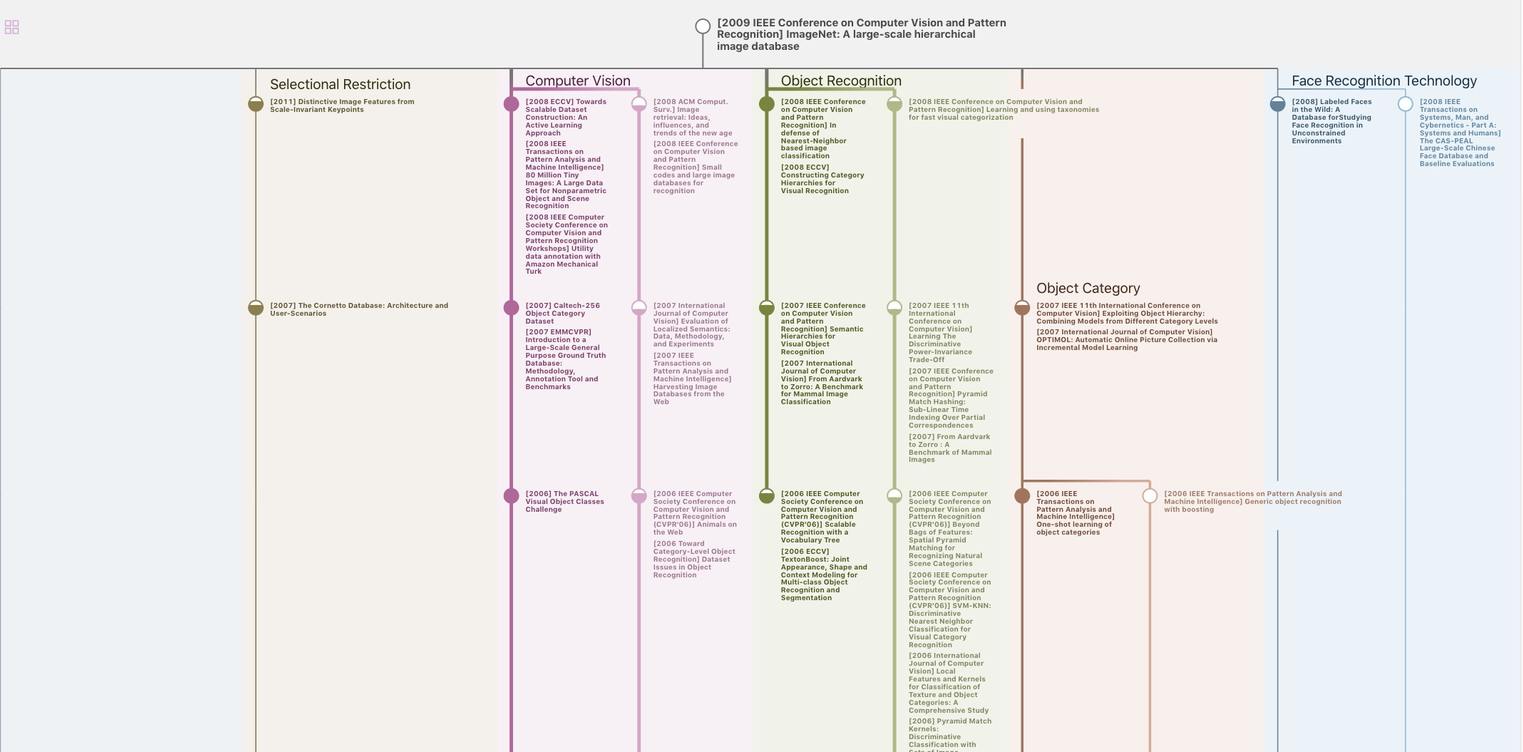
生成溯源树,研究论文发展脉络
Chat Paper
正在生成论文摘要