A Structured Latent Space for Human Body Motion Generation
2022 International Conference on 3D Vision (3DV)(2022)
摘要
We propose a framework to learn a structured latent space to represent 4D human body motion, where each latent vector encodes a full motion of the whole 3D human shape. On one hand several data-driven skeletal animation models exist proposing motion spaces of temporally dense motion signals, but based on geometrically sparse kinematic representations. On the other hand many methods exist to build shape spaces of dense 3D geometry, but for static frames. We bring together both concepts, proposing a motion space that is dense both temporally and geometrically. Once trained, our model generates a multi-frame sequence of dense 3D meshes based on a single point in a low-dimensional latent space. This latent space is built to be structured, such that similar motions form clusters. It also embeds variations of duration in the latent vector, allowing semantically close sequences that differ only by temporal unfolding to share similar latent vectors. We demonstrate experimentally the structural properties of our latent space, and show it can be used to generate plausible interpolations between different actions. We also apply our model to 4D human motion completion, showing its promising abilities to learn spatiotemporal features of human motion. Code is available at https://github.com/mmarsot/A_structured_latent_space.
更多查看译文
关键词
motion,latent,human,Spatio temporal,prior
AI 理解论文
溯源树
样例
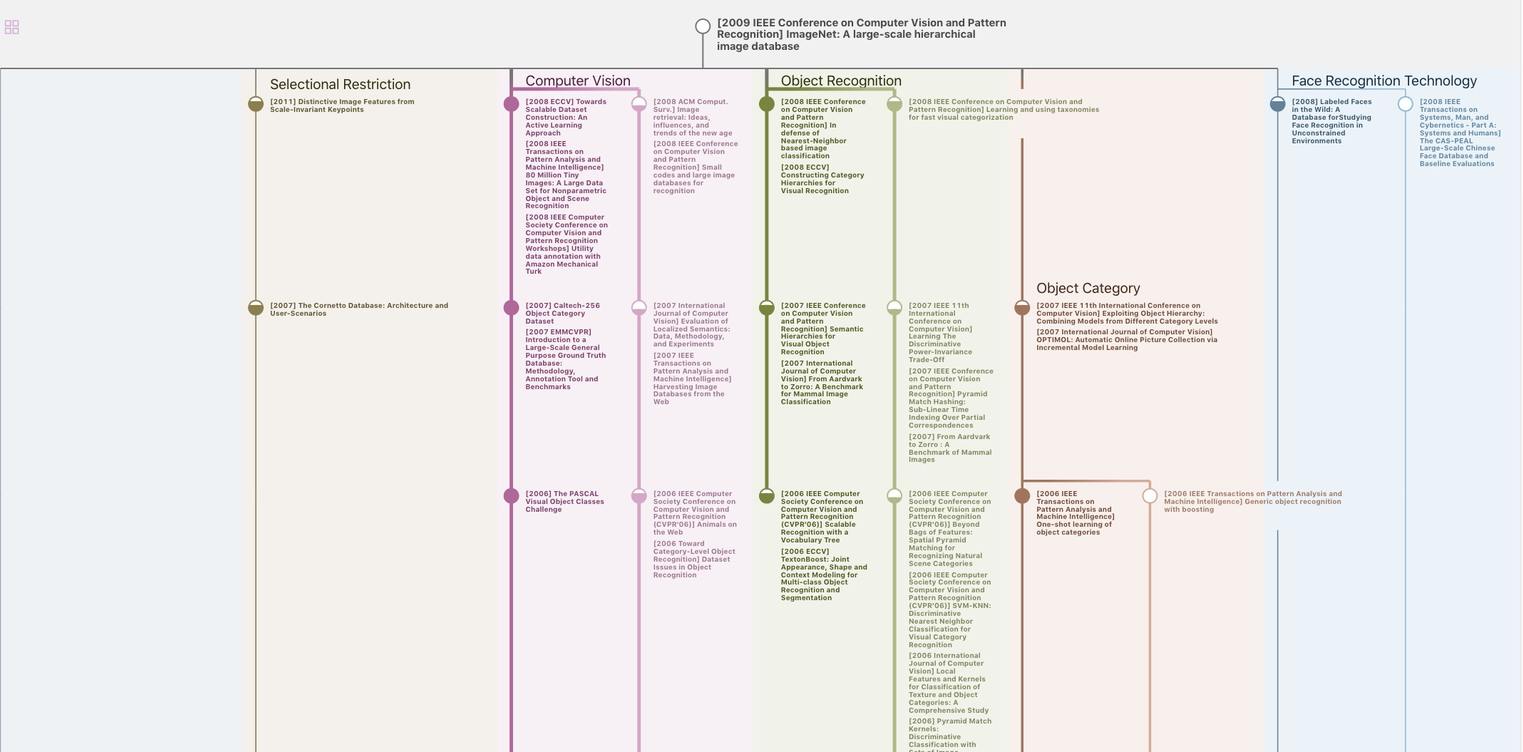
生成溯源树,研究论文发展脉络
Chat Paper
正在生成论文摘要