Electroencephalography robust statistical linear modelling using a single weight per trial
Aperture Neuro(2022)
摘要
Being able to remove or weigh down the influence of outlier data is desirable for any statistical model. While magnetic and electroencephalographic (MEEG) data are often averaged across trials per condition, it is becoming common practice to use information from all trials to build statistical linear models. Individual trials can, however, have considerable weight and thus bias inferential results (effect sizes as well as thresholded t/F/p maps). Here, rather than looking for univariate outliers, defined independently at each measurement point, we apply the principal component projection (PCP) method at each channel, deriving a single weight per trial at each channel independently. Using both synthetic data and open electroencephalographic (EEG) data, we show (1) that PCP is efficient at detecting a large variety of outlying trials; (2) how PCP-based weights can be implemented in the context of the general linear model (GLM) with accurate control of type 1 family-wise error rate; and (3) that our PCP-based weighted least squares (WLS) approach increases the statistical power of group analyses as well as a much slower iterative reweighted least squares (IRLS) approach, although the weighting scheme is markedly different. Together, our results show that WLS based on PCP weights derived from whole trial profiles is an efficient method to weigh down the influence of outlier EEG data in linear models.
更多查看译文
关键词
robust statistical linear
AI 理解论文
溯源树
样例
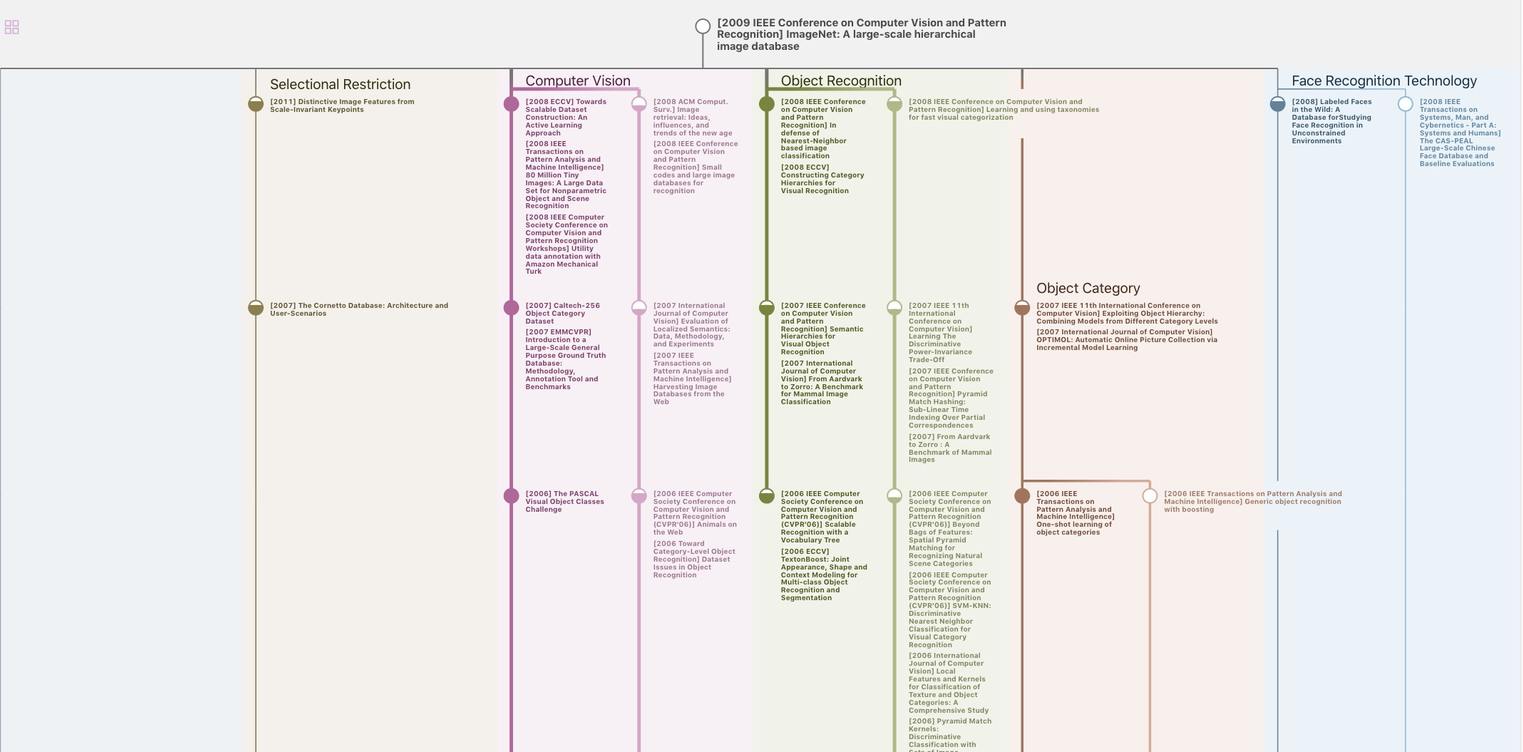
生成溯源树,研究论文发展脉络
Chat Paper
正在生成论文摘要