AlgaeNet: A Deep-Learning Framework to Detect Floating Green Algae From Optical and SAR Imagery
IEEE JOURNAL OF SELECTED TOPICS IN APPLIED EARTH OBSERVATIONS AND REMOTE SENSING(2022)
摘要
This article developed a scalable deep-learning model, the AlgaeNet model, for floating Ulva prolifera (U. prolifera) detection in moderate resolution imaging spectroradiometer (MODIS) and synthetic aperture radar (SAR) images. We labeled 1055/4071 pairs of samples, among which 70%/30% were used for training/validation. As a result, the model reached an accuracy of 97.03%/99.83% and a mean intersection over union of 48.57%/88.43% for the MODIS/SAR images. The model was designed based on the classic U-Net model with two tailored modifications. First, the physics information input was a multichannel multisource remote sensing data. Second, a new loss function was developed to resolve the class-unbalanced samples (algae and seawater) and improve model performance. In addition, this model is expandable to process images from optical sensors (e.g., MODIS/GOCI/Landsat) and SAR (e.g., Sentinel-1/GF-3/Radarsat-1 or 2), reducing the potential biases due to the selection of extraction thresholds during the traditional threshold-based segmentation. We process satellite images containing U. prolifera in the Yellow Sea and draw two conclusions. First, adding the 10-m high-resolution SAR imagery shows a 63.66% increase in algae detection based on the 250-m resolution MODIS image alone. Second, we define a floating and submerged ratio number (FS ratio) based on the floating and submerged parts of U. prolifera detected by SAR and MODIS. A research vessel measurement confirms the FS ratio to be a good indicator for representing different life phases of U. prolifera.
更多查看译文
关键词
Algae,MODIS,Synthetic aperture radar,Optical sensors,Optical imaging,Marine vehicles,Spatial resolution,Deep learning (DL),green algae detection,satellite remote sensing
AI 理解论文
溯源树
样例
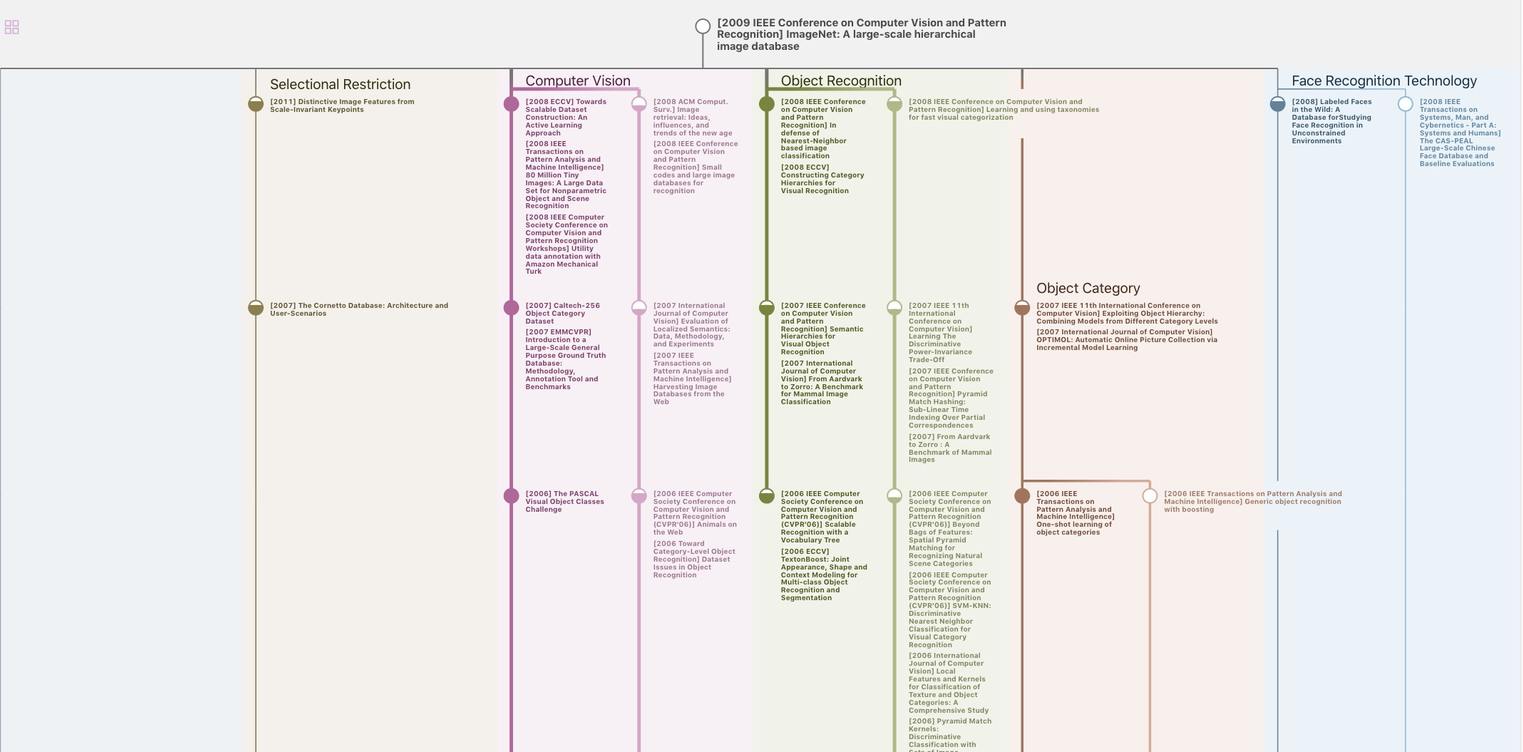
生成溯源树,研究论文发展脉络
Chat Paper
正在生成论文摘要