The Role of Pretrained Representations for the OOD Generalization of Reinforcement Learning Agents
arXiv (Cornell University)(2021)
摘要
Building sample-efficient agents that generalize out-of-distribution (OOD) in real-world settings remains a fundamental unsolved problem on the path towards achieving higher-level cognition. One particularly promising approach is to begin with low-dimensional, pretrained representations of our world, which should facilitate efficient downstream learning and generalization. By training 240 representations and over 10,000 reinforcement learning (RL) policies on a simulated robotic setup, we evaluate to what extent different properties of pretrained VAE-based representations affect the OOD generalization of downstream agents. We observe that many agents are surprisingly robust to realistic distribution shifts, including the challenging sim-to-real case. In addition, we find that the generalization performance of a simple downstream proxy task reliably predicts the generalization performance of our RL agents under a wide range of OOD settings. Such proxy tasks can thus be used to select pretrained representations that will lead to agents that generalize.
更多查看译文
关键词
pretrained representations,ood generalization,learning,agents
AI 理解论文
溯源树
样例
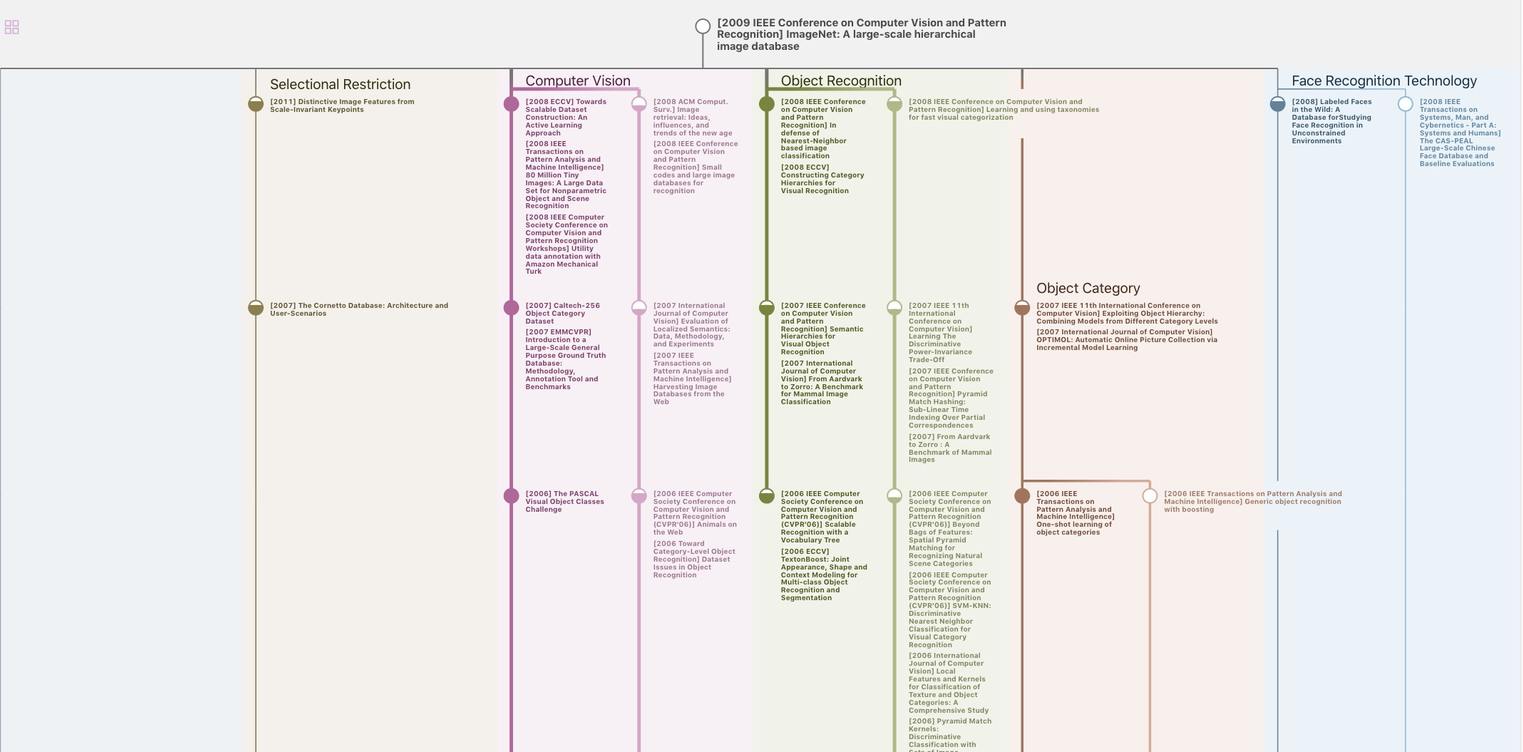
生成溯源树,研究论文发展脉络
Chat Paper
正在生成论文摘要