Who needs explanation and when? Juggling explainable AI and user epistemic uncertainty
INTERNATIONAL JOURNAL OF HUMAN-COMPUTER STUDIES(2022)
摘要
In recent years, AI explainability (XAI) has received wide attention. Although XAI is expected to play a positive role in decision-making and advice acceptance, various opposing effects have also been found. The opposing effects of XAI highlight the critical role of context, especially human factors, in understanding XAI's impacts. This study investigates the effects of providing three types of post-hoc explanations (alternative advice, prediction confidence scores, and prediction rationale) on two context-specific user decision-making outcomes (AI advice acceptance and advice adoption). Our field experiment results show that users' epistemic uncertainty matters when understanding XAI's impacts. As users' epistemic uncertainty increases, only providing prediction rationale is beneficial, whereas providing alternative advice and showing prediction confidence scores may hinder users' advice acceptance. Our study contributes to the emerging literature on the human aspects of XAI by clarifying XAI and showing that XAI may not always be desirable. It also contributes by highlighting the importance of considering user profiles when predicting XAI's impacts, designing XAI, and providing professional services with AI.
更多查看译文
关键词
AI explainability,AI advice acceptance,Medical AI,Human-AI interaction,Experiment
AI 理解论文
溯源树
样例
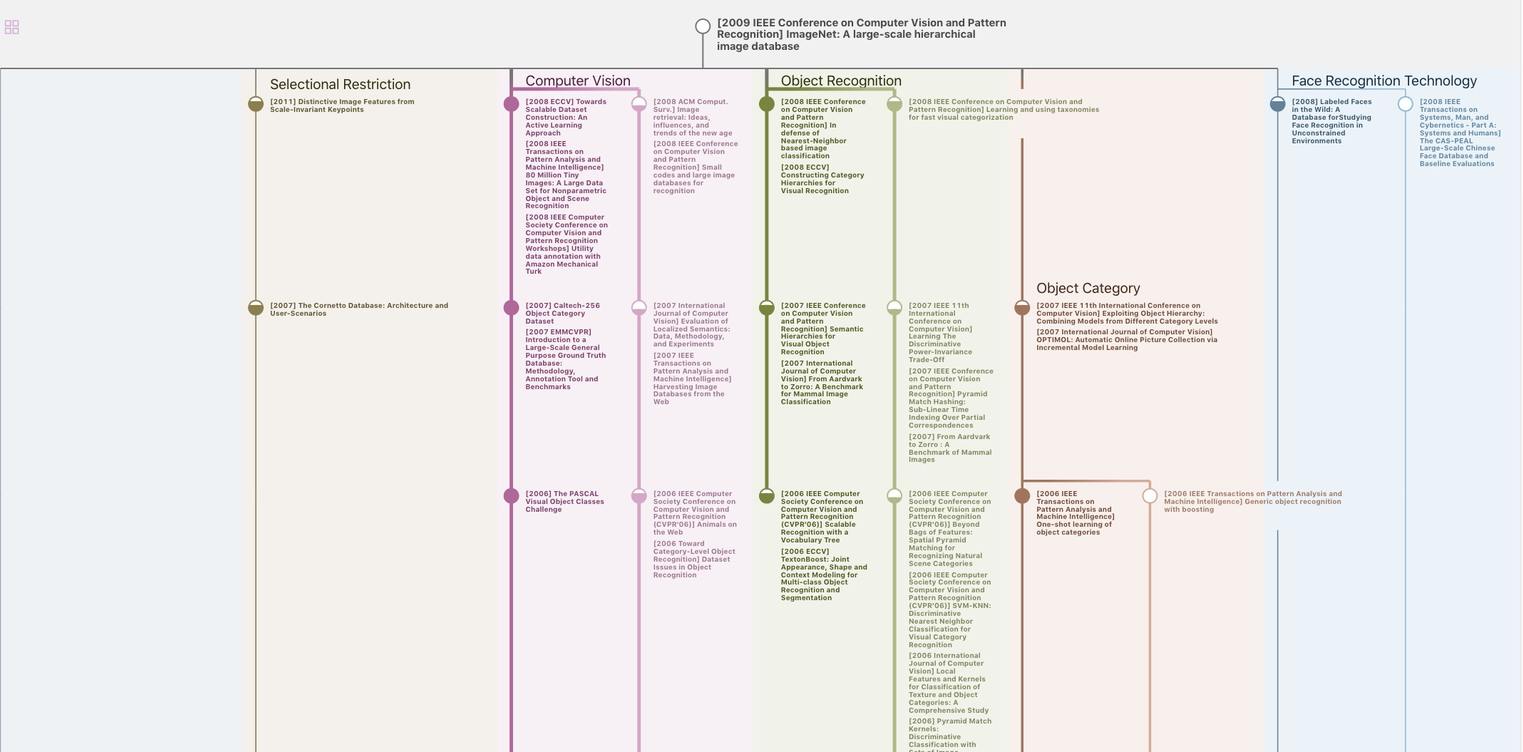
生成溯源树,研究论文发展脉络
Chat Paper
正在生成论文摘要