Making Progress Based on False Discoveries
ArXiv(2022)
摘要
We consider the question of adaptive data analysis within the framework of convex optimization. We ask how many samples are needed in order to compute ε-accurate estimates of O(1/ε) gradients queried by gradient descent, and we provide two intermediate answers to this question. First, we show that for a general analyst (not necessarily gradient descent) Ω(1/ε) samples are required. This rules out the possibility of a foolproof mechanism. Our construction builds upon a new lower bound (that may be of interest of its own right) for an analyst that may ask several non adaptive questions in a batch of fixed and known T rounds of adaptivity and requires a fraction of true discoveries. We show that for such an analyst Ω( √ T/ε) samples are necessary. Second, we show that, under certain assumptions on the oracle, in an interaction with gradient descent Ω̃(1/ε) samples are necessary. Our assumptions are that the oracle has only first order access and is post-hoc generalizing. First order access means that it can only compute the gradients of the sampled function at points queried by the algorithm. Our assumption of post-hoc generalization follows from existing lower bounds for statistical queries. More generally then, we provide a generic reduction from the standard setting of statistical queries to the problem of estimating gradients queried by gradient descent. These results are in contrast with classical bounds that show that with O(1/ε) samples one can optimize the population risk to accuracy of O(ε) but, as it turns out, with spurious gradients.
更多查看译文
AI 理解论文
溯源树
样例
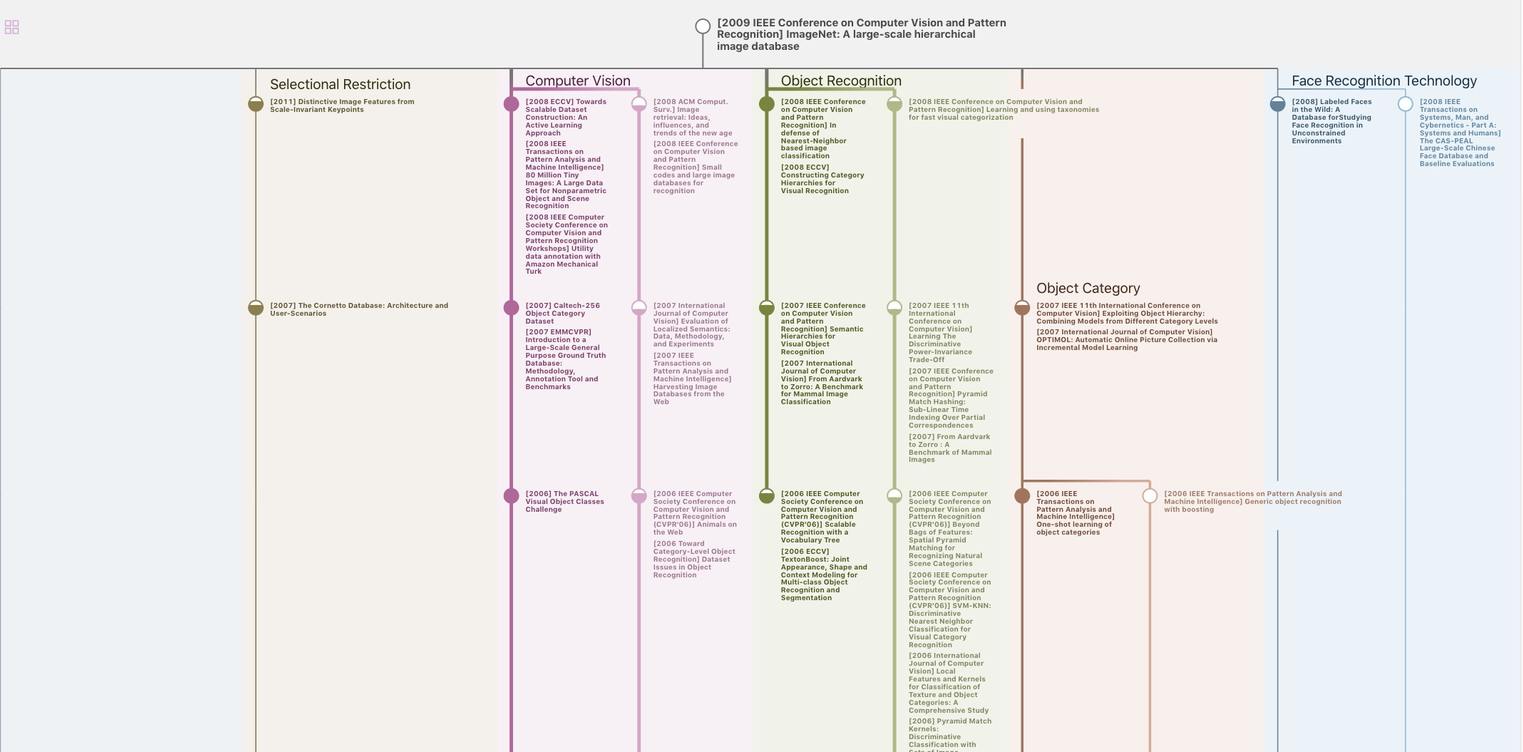
生成溯源树,研究论文发展脉络
Chat Paper
正在生成论文摘要