Streamed Punctuation Annotation using Transformers
semanticscholar(2021)
摘要
To improve readability, punctuation prediction is typically performed on text output by an Automatic Speech Recognition (ASR) model. We introduce a Transformer-based model to predict punctuation marks on unpunctuated text suitable for text streamed word-for-word, as is often the case for ASR models. We propose a decoding strategy that delays punctuation marks’ insertion in case of uncertainty until a specific threshold is reached. Leveraging existing pre-trained language models in conjunction with a special token for acoustic pause features, we achieve state-of-the-art performance for punctuation prediction on the MGB dataset and results that compare favourably to the state-of-the-art on the IWSLT11 dataset while using comparatively less computing power than previous work by using downsampling. To make the model viable for real-time use in combination with an ASR system and on low-resource devices, we evaluate input truncation and weight quantization. We show these techniques lead to faster-than-real-time inference speeds and a significant reduction in model size.
更多查看译文
AI 理解论文
溯源树
样例
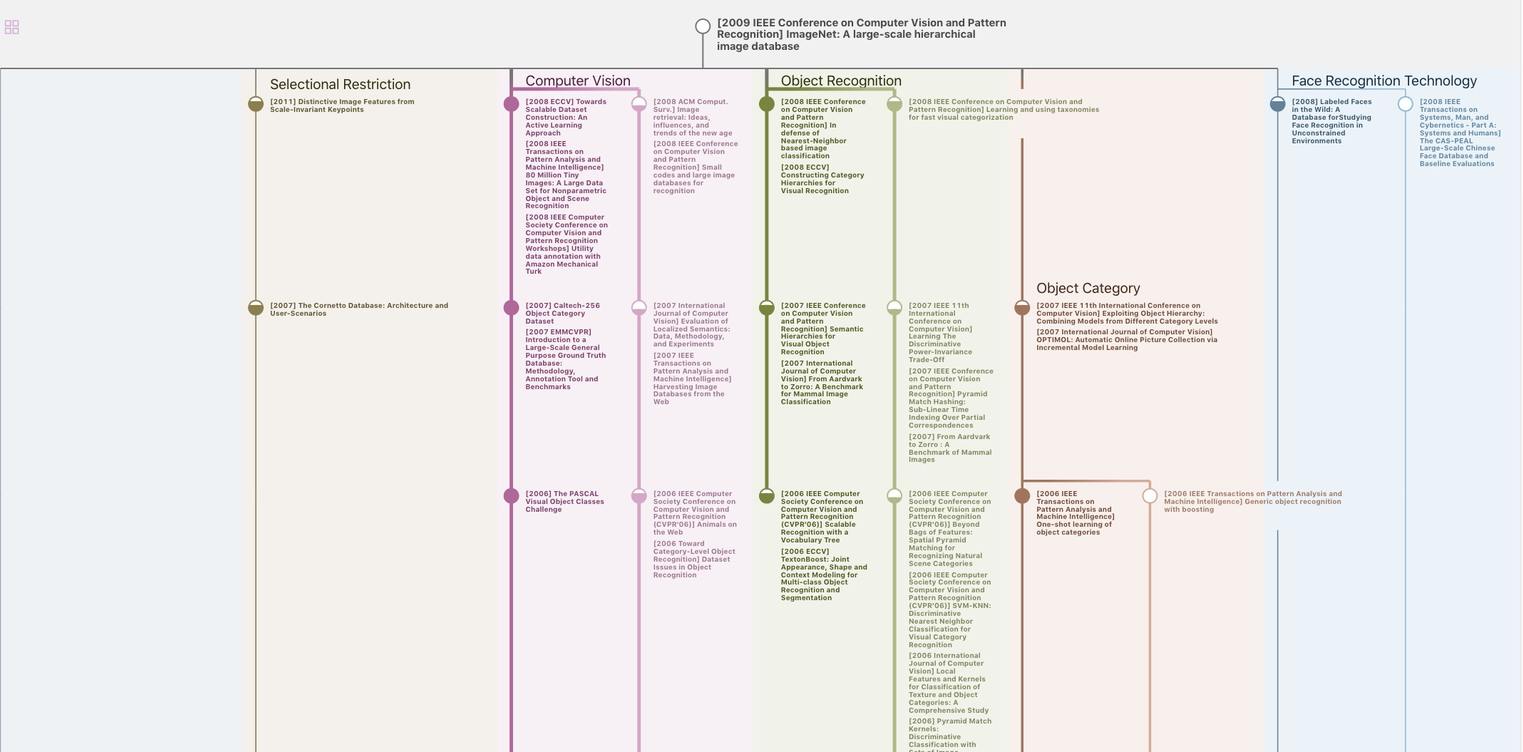
生成溯源树,研究论文发展脉络
Chat Paper
正在生成论文摘要