Integrated Clinical and Genomic Models to Predict Optimal Cytoreduction in High Grade Serous Ovarian Cancer
CANCERS(2022)
摘要
Background: Advanced high grade serous (HGSC) ovarian cancer is treated with either primary surgery followed by chemotherapy or neoadjuvant chemotherapy followed by interval surgery. The decision to proceed with surgery either primarily or after chemotherapy is based on a surgeon’s clinical assessment and prediction of an optimal outcome. Optimal surgery is correlated with improved overall survival. This clinical assessment results in an optimal surgery approximately 70% of the time. We hypothesize that this prediction can be improved by using biological tumor data to predict optimal cytoreduction.Methods: With access to a large biobank of ovarian cancer tumors, we obtained genomic data on 83 patients encompassing gene expression, exon expression, long non-coding RNA, micro RNA, single nucleotide variants, copy number variation, DNA methylation, and fusion transcripts. We then used machine learning to incorporate this data with pre-operative clinical information to create predictive models which successfully predicted whether or not a patient’s cytoreductive surgery would have an optimal outcome. These models were then validated within The Cancer Genome Atlas (TCGA) HGSC database. Results: Of the 124 models created and validated, 21 performed at least equal if not better than our historical clinical rate of optimal debulking in advanced-stage HGSC as a control, 78%. Conclusions: This is the first time tumor genomic data has been used to predict surgical outcome in ovarian cancer. Prospective validation of these models could result in improving our ability to objectively predict which patients will undergo optimal cytoreduction and, therefore, improve our ovarian cancer outcomes.
更多查看译文
关键词
high-grade serous carcinoma, ovarian cancer, surgical cytoreduction, prediction modeling
AI 理解论文
溯源树
样例
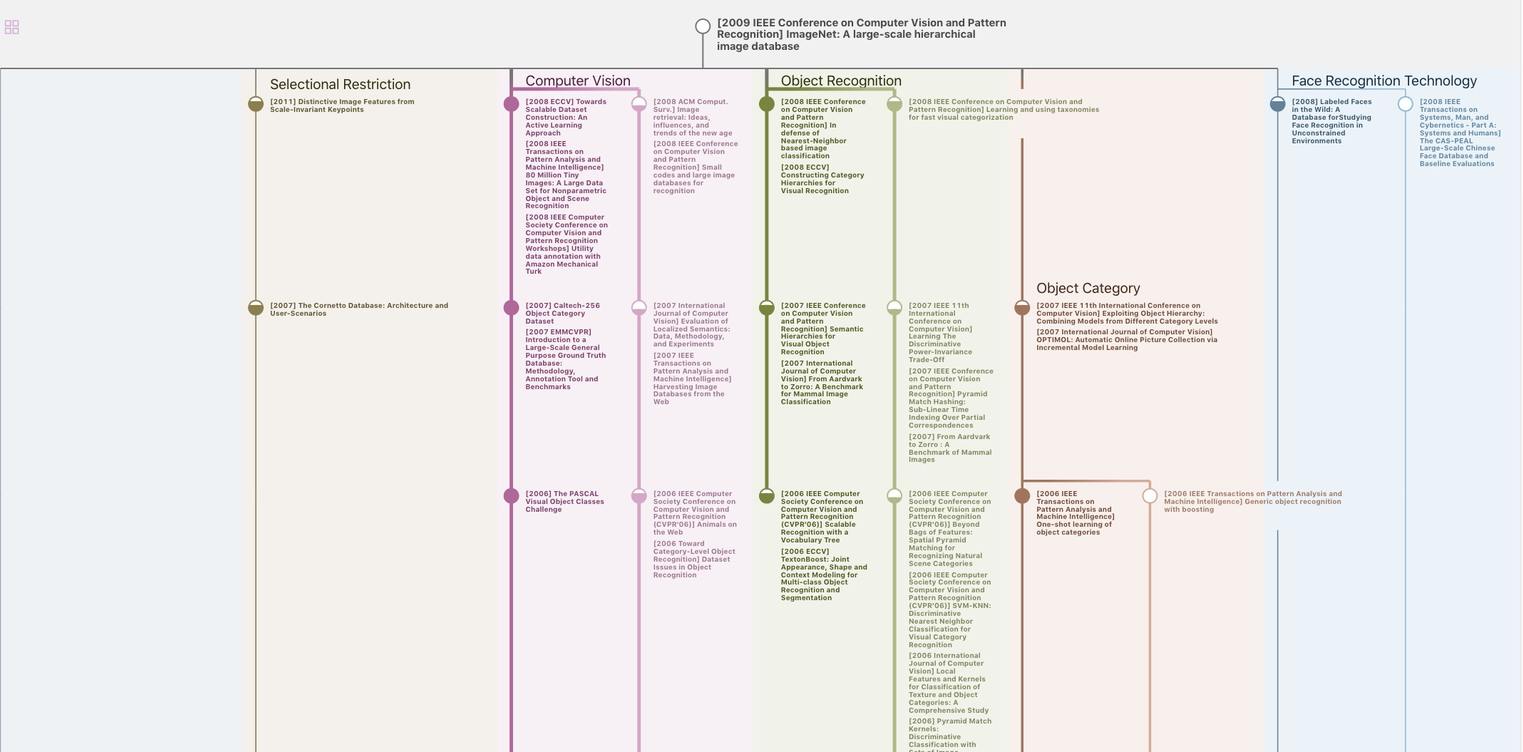
生成溯源树,研究论文发展脉络
Chat Paper
正在生成论文摘要