LTrust: An Adaptive Trust Model Based on LSTM for Underwater Acoustic Sensor Networks
IEEE Transactions on Wireless Communications(2022)
摘要
As an effective security mechanism, trust models have been proposed to estimate the reliability of the individual nodes in Underwater Acoustic Sensor Networks (UASNs) during adverse attacks. However, existing trust models neglect the relative importance of the different nodes within the network topology. Further, few trust models study the effects of defective recommendation trust filtering. In this work, we propose an adaptive trust model based on the Long Short-Term Memory (LSTM) network model for UASNs, which we term LTrust. The LTrust is composed of two stages: trust data collection and trust evaluation. In the first stage, the characteristics of the network topology are leveraged towards evaluating direct trust evidence, by aggregating the communication trust and environment trust metrics; a defective recommendation filtering method is designed for broadcasting accurate trust recommendations among the nodes. In the second stage, an adaptive trust model is designed based on the LSTM model, to identify anomalous nodes by evaluating their trust value. The LTrust model has been tested under both hybrid attack and single-mode attack scenarios. Simulation results demonstrate that the LTrust achieves effective performance, as compared to other approaches proposed in the literature, in terms of trust value, accuracy and error rate.
更多查看译文
关键词
Anomaly detection,long short-term memory network,recommendation filtering,trust evaluation,underwater acoustic sensor networks
AI 理解论文
溯源树
样例
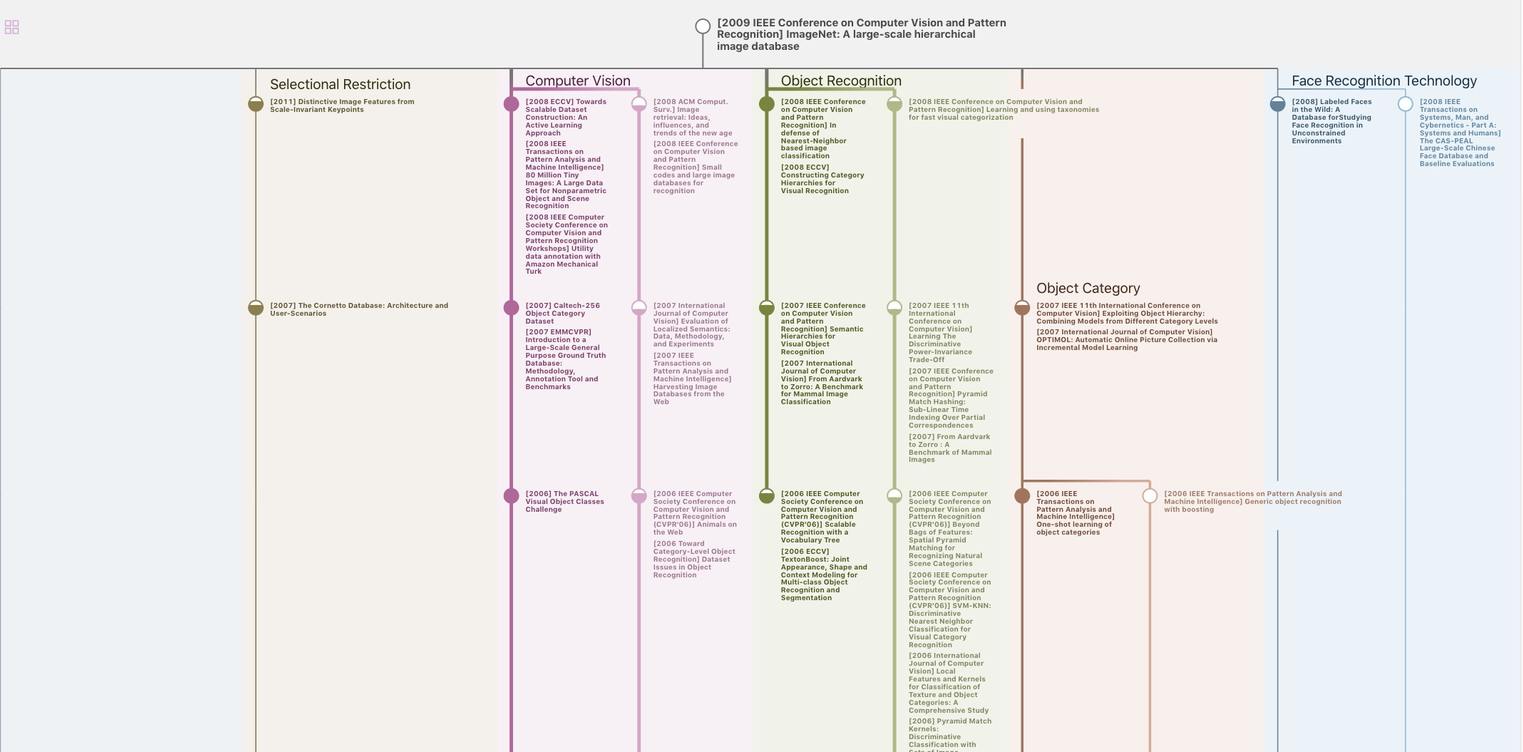
生成溯源树,研究论文发展脉络
Chat Paper
正在生成论文摘要