Fit The Right NP-Hard Problem: End-to-end Learning of Integer Programming Constraints
semanticscholar(2020)
摘要
Bridging logical and algorithmic reasoning with modern machine learning techniques is a fundamental challenge with potentially transformative impact. On the algorithmic side, many NP-Hard problems can be expressed as integer programs, in which the constraints play the role of their “combinatorial specification”. In this work, we aim to integrate integer programming solvers into neural network architectures by providing loss functions for both the objective and the constraints. The resulting end-to-end trainable architectures have the power of jointly extracting features from raw data and of solving a suitable (learned) combinatorial problem with state-of-the-art integer programming solvers. We experimentally validate our approach on artificial datasets created from random constraints, and on solving KNAPSACK instances from their description in natural language.
更多查看译文
AI 理解论文
溯源树
样例
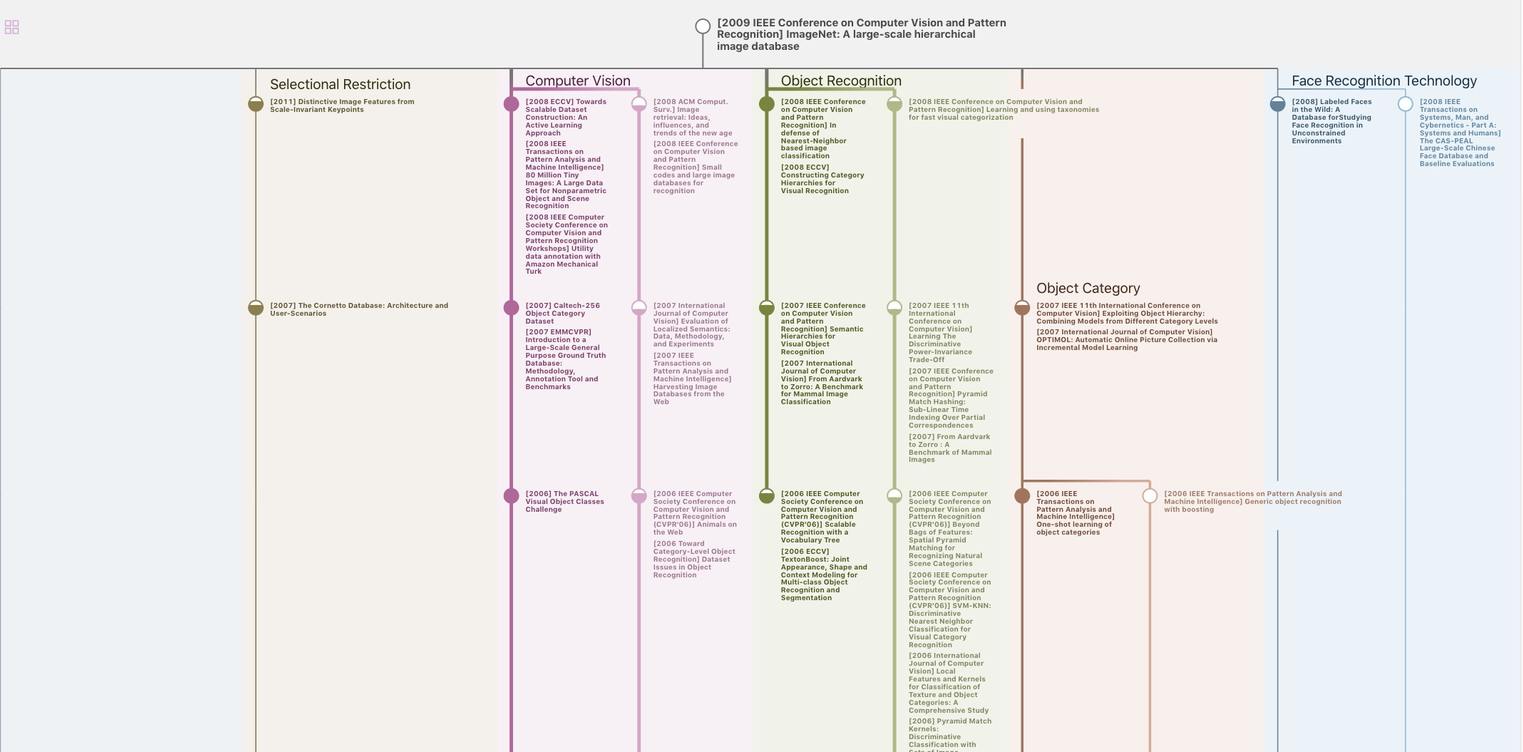
生成溯源树,研究论文发展脉络
Chat Paper
正在生成论文摘要