Is GPT-3 Text Indistinguishable from Human Text? Scarecrow: A Framework for Scrutinizing Machine Text
semanticscholar(2021)
摘要
Modern neural language models can produce remarkably fluent and grammatical text. So much, in fact, that recent work by Clark et al. (2021) has reported that conventional crowdsourcing can no longer reliably distinguish between machine-authored (GPT-3) and humanauthored writing. As errors in machine generations become ever subtler and harder to spot, it poses a new challenge to the research community for robust machine text evaluation. We propose a new framework called SCARECROW for scrutinizing machine text via crowd annotation. To support the broad range of real machine errors that can be identified by laypeople, the ten error categories of SCARECROW— such as redundancy , commonsense errors , and incoherence —are identified through several rounds of crowd annotation experiments without a predefined ontology. We then use SCARECROW to collect over 41k error spans in human-written and machinegenerated paragraphs of English language news text. We isolate factors for detailed analysis, including parameter count, training data, and various decoding-time configurations. Our approach successfully quantifies measurable gaps between human authored text and generations from models of several sizes, including fourteen configurations of GPT-3. In addition, our analysis unveils new insights, with detailed rationales provided by laypeople, e.g., that the commonsense capabilities have been improving with larger models while math capabilities have not, and that the choices of simple decoding hyperparameters can make remarkable differences on the perceived quality of machine text. We release our training material, annotation toolkit and dataset at https: //yao-dou.github.io/scarecrow/.
更多查看译文
AI 理解论文
溯源树
样例
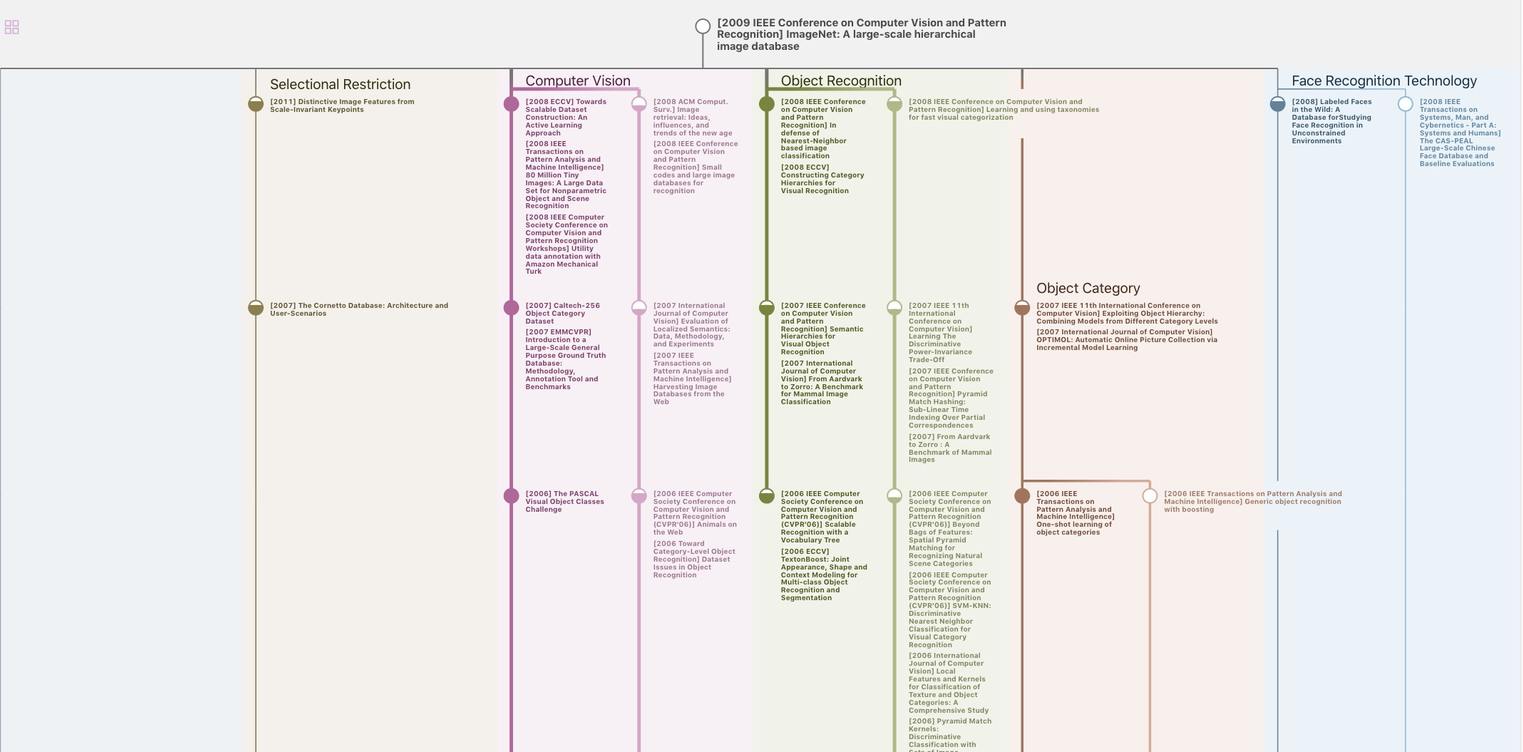
生成溯源树,研究论文发展脉络
Chat Paper
正在生成论文摘要