Tracking normal fluid flow in He II with unsupervised machine learning
semanticscholar(2022)
摘要
Time dependent observations of point-to-point correlations of the velocity vector field (structure functions) are necessary to fully understand and model fluid flow around complex objects. Using thermal gradients, we induced flow of the normal fluid component of liquid He II and observed the flow by recording fluorescence of He*2 excimers produced by neutron capture throughout a ~cm3 volume. We applied an unsupervised machine learning algorithm to identify individual excimer clouds and then track their motion with millimeter and millisecond precision using a purpose-built correlation metric. Owing to the fact excimers are produced over a large region, the data are sparse in comparison to other techniques used to produce excimers. Machine learning is crucially important to track flow represented by sparse data—a situation encountered in other fields of science—and its importance will increase as improvements are made to overcome the sparsity of data.
更多查看译文
关键词
normal fluid flow,unsupervised machine learning
AI 理解论文
溯源树
样例
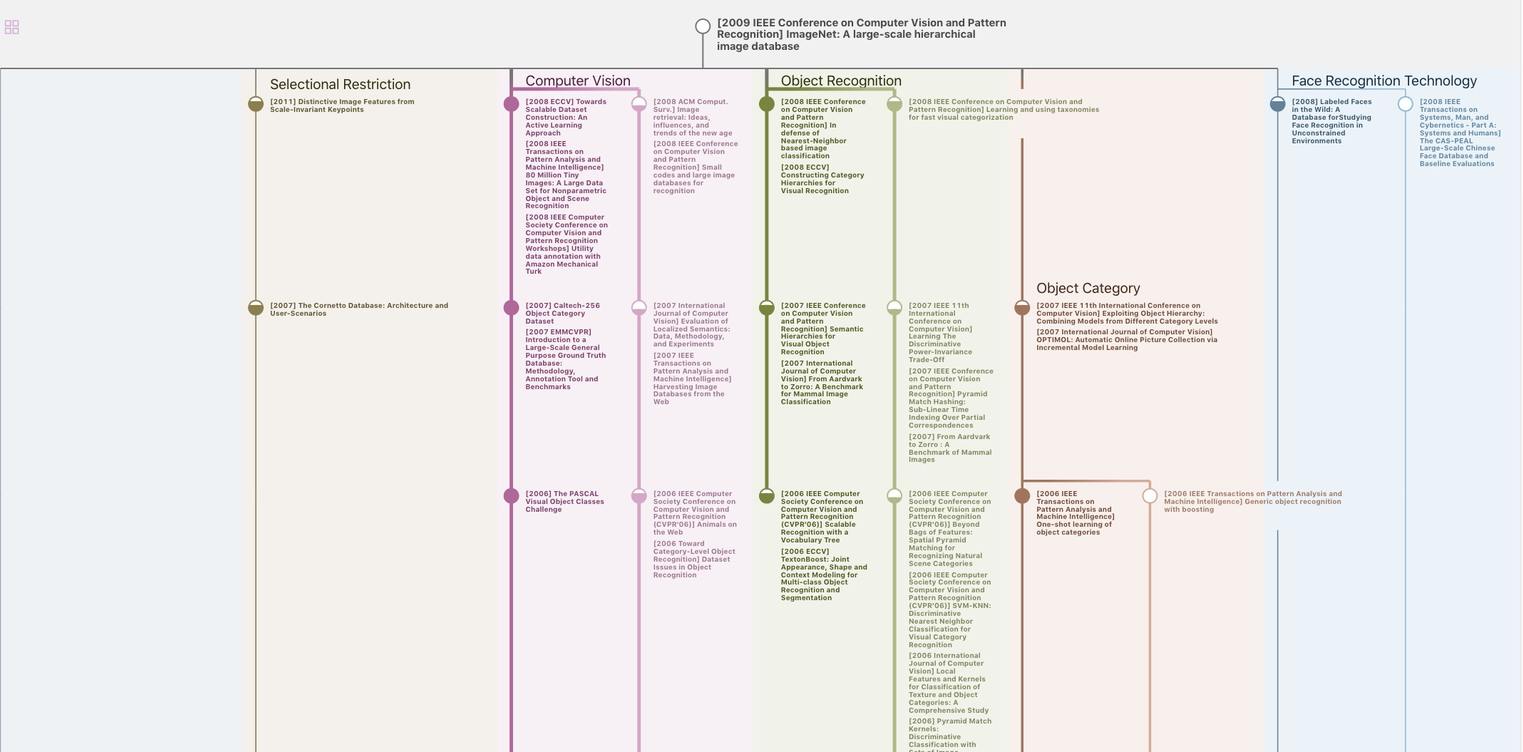
生成溯源树,研究论文发展脉络
Chat Paper
正在生成论文摘要