Two-Stage Cross-Modality Transfer Learning Method for Military-Civilian SAR Ship Recognition
IEEE GEOSCIENCE AND REMOTE SENSING LETTERS(2022)
摘要
Military-civilian attribute recognition of ships in synthetic aperture radar (SAR) imagery plays an important role in marine surveillance. However, high-quality labeled data are hard to obtain for SAR ships, which hinder the development of deep learning models. Considering that models directly transferred from labeled optical images cannot achieve satisfactory performance for SAR applications due to the great discrepancy of different modalities, we propose a two-stage transfer learning method by combining the data-level and feature-level knowledge transfer. First, CycleGAN is adopted in the first stage to transfer the labeled optical image domain to the intermediate SAR-like image domain with the attribute labels. Then, a novel network called Domain Transfer using Adversarial learning and Metric learning (DTAM) is proposed to realize the task of military-civilian ship recognition by the domain adaption of the intermediate domain and the target SAR domain with joint adversarial learning and metric learning. To validate the proposed method, we establish a high-resolution SAR ship recognition dataset (HRSSRD), containing SAR and optical images of military and civilian ships. The experimental results show that the proposed two-stage architecture exhibits promising performance on the problem of SAR military-civilian ship recognition.
更多查看译文
关键词
Optical imaging,Synthetic aperture radar,Marine vehicles,Optical sensors,Image recognition,Task analysis,Radar polarimetry,Domain adaption,military-civilian ship recognition,optical remote sensing images,synthetic aperture radar (SAR) imagery,transfer learning
AI 理解论文
溯源树
样例
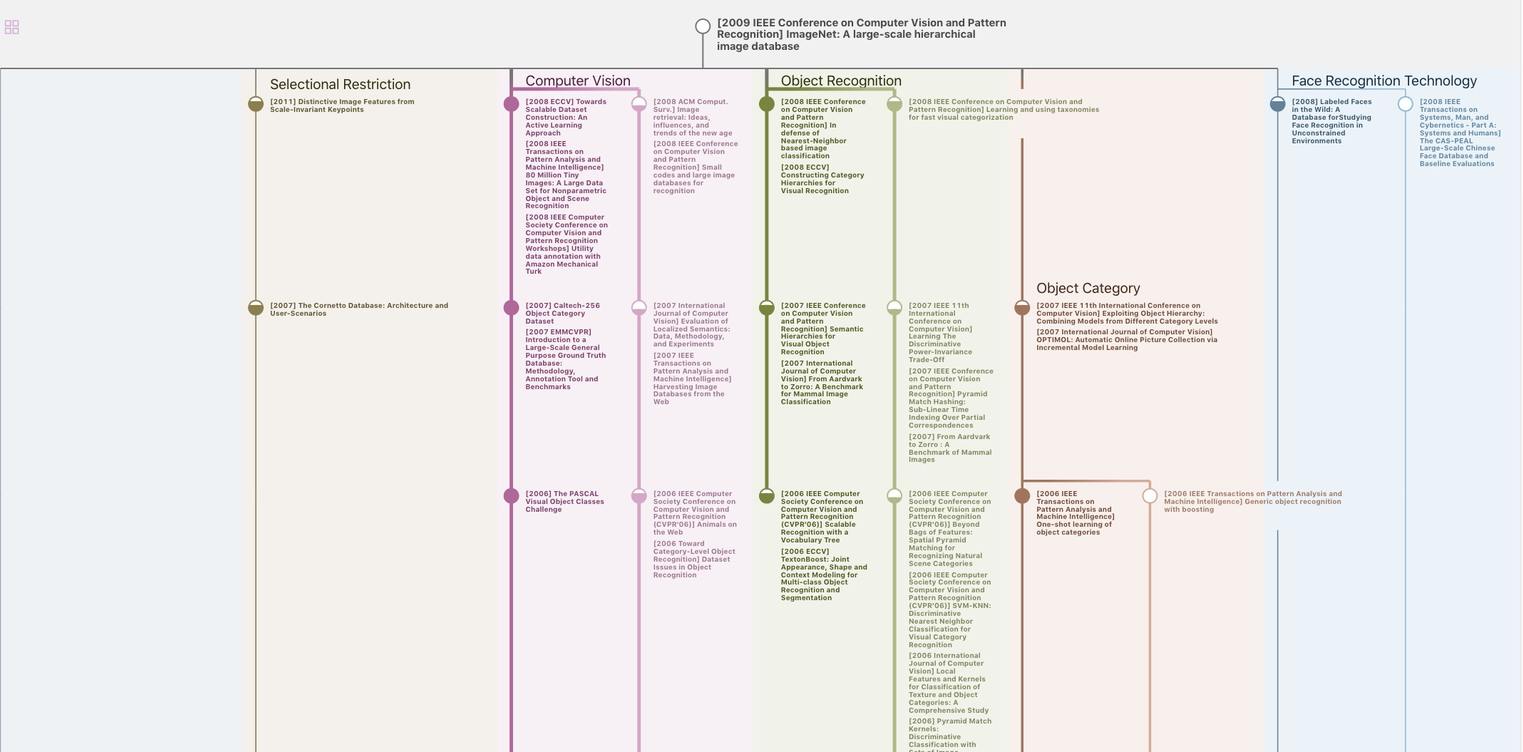
生成溯源树,研究论文发展脉络
Chat Paper
正在生成论文摘要