Machine-Learning Inversion of Forest Vertical Structure Based on 2-D-SGVBVoG Model for P-Band Pol-InSAR
IEEE TRANSACTIONS ON GEOSCIENCE AND REMOTE SENSING(2022)
摘要
The polarimetric interferometric synthetic aperture radar (Pol-InSAR) model under P-band observations exhibits vertical structure diversity. Compared with the exponential-based random volume over ground (RVoG) model, the Gaussian vertical backscatter volume over ground (GVBVoG) model expresses a more complex forest vertical structure via introducing more parameters. On account of the influence of topographic fluctuation on the model, this article establishes the sloped Gaussian vertical backscatter volume over ground (SGVBVoG) model by drawing into the terrain slope. Based upon the SGVBVoG model, this article develops the 2-D SGVBVoG (2-D-SGVBVoG) model by defining the structure factor, which effectively reduces the model complexity from three to two dimensions. In the 2-D-SGVBVoG model inversion, in view of the diversity of forest species, age, shape, density, etc., in the natural scene and the variation of specific radar systems, a structure factor prediction scheme relying on machine learning is proposed. In the machine-learning model training, the radar incidence angle and the PDHigh coherence acquired by coherence optimization with terrain phase removal are utilized as the variables for characterizing the structure factor. Ultimately, in the case of fixing structure factor, a geometric inversion process on the complex plane is put forward to extract the forest height. The BIOSAR 2008 P-band Pol-InSAR data validation shows that the proposed method achieves an RMSE of 3.07 m, which is 24.0% better than the three-baseline SRVoG inversion.
更多查看译文
关键词
Forest vertical structure,Gaussian vertical backscatter volume,machine learning,P-band polarimetric interferometric synthetic aperture radar (Pol-InSAR)
AI 理解论文
溯源树
样例
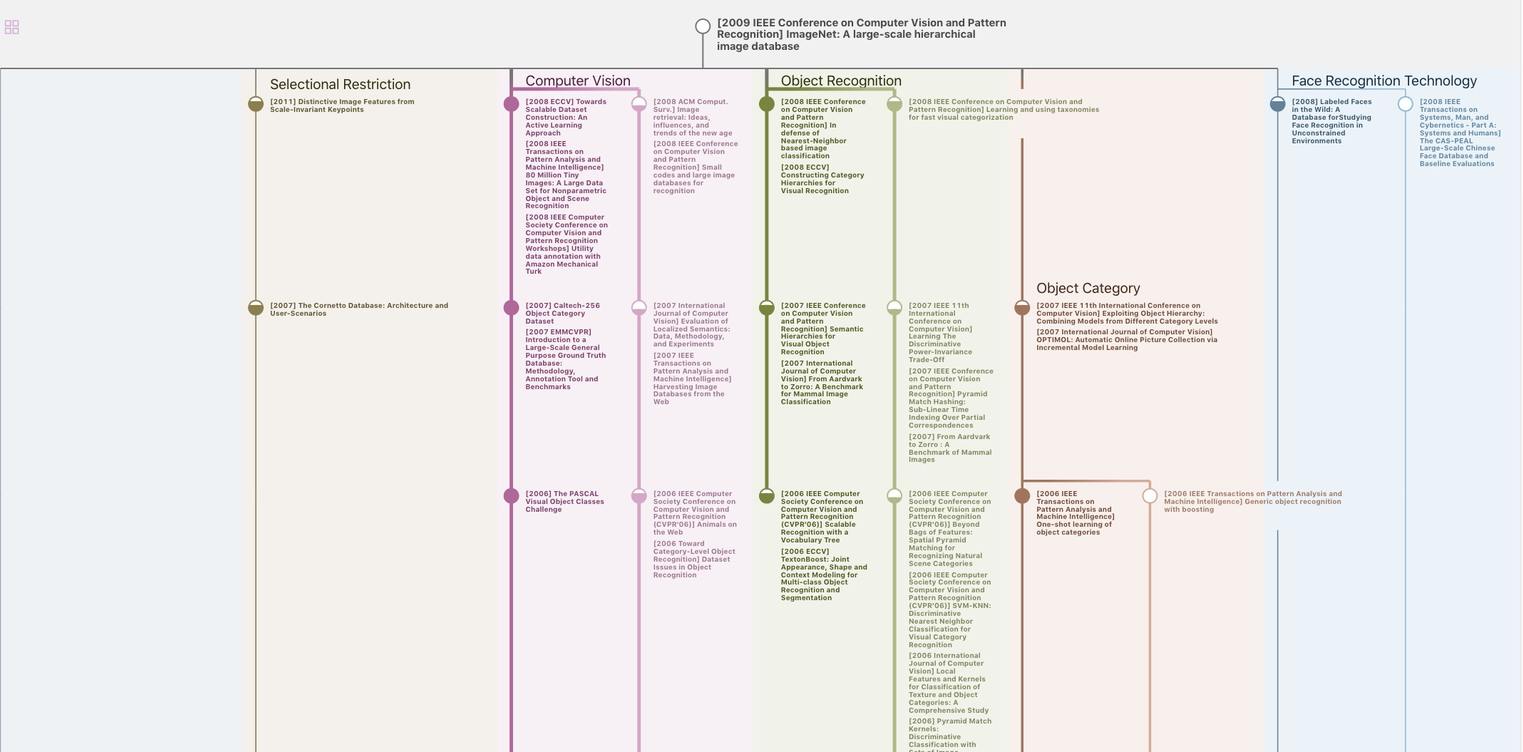
生成溯源树,研究论文发展脉络
Chat Paper
正在生成论文摘要