Artificial Intelligence Technologies for Detection and Quantification of Hepatic Steatosis: A Scoping Review (Preprint)
semanticscholar(2021)
摘要
BACKGROUND
Non-alcoholic fatty liver disease linked to an increased risk of morbidity and mortality in afflicted individuals. Non-invasive liver imaging technologies such ultrasound (US) is the preferable method for screening hepatic steatosis because to its non-invasiveness, cheap cost, and widespread availability.
Artificial intelligence (AI), in particular deep learning algorithms, has garnered widespread notice for its superior performance on image identification and quantification. They are capable of quantitatively assessing complicated medical image properties automatically and achieving better diagnostic accuracy while increasing efficiency. AI is frequently utilized and gaining popularity in the field of liver medical imaging, which includes radiology, nuclear medicine and ultrasound. AI can assist physicians in making more accurate and reproducible imaging diagnoses while also reducing the workload of physicians.
OBJECTIVE
The primary purpose of this work was to conduct a scoping review of artificial intelligence technologies for hepatic steatosis detection and quantification. It also aimed to explore the role of AI technologies on Ultrasonography for the detection and quantification of hepatic steatosis.
METHODS
The researcher searched several electronic bibliographies including Google Scholar, PubMed, EMBASE, CINAHL, PsychINFO, ACM Digital, IEEEXplore, and Scopus. The references retrieved were investigated for additional relevant studies through the backward and forward reference list checking strategy. One reviewer carried out the studies selection and data extraction.
RESULTS
A total of 718 publications were retrieved; 268 duplicates were removed. After scanning the titles and abstracts, 234 were excluded. After scanning the full texts, many articles were excluded for the following reasons: studies focusing on diseases other than liver parenchymal diseases (n = 39), studies that did not confirm the specified outcomes or confirmation study population (n = 58), studies that were not original research, i.e., reviews, editorials (n = 106), and studies not written in English (n = 8). The qualitative analysis comprised a total of 25 publications.
CONCLUSIONS
This review illustrates the potential for AI systems to aid in the diagnosis and stage of liver fibrosis and non-alcoholic fatty liver disease. By incorporating AI into established noninvasive methods, effective diagnostic tools with an ideal mix of sensitivity and specificity are created. Before adopting these AI-assisted systems in clinical practice, it is necessary to validate these models in additional independent cohorts.
更多查看译文
AI 理解论文
溯源树
样例
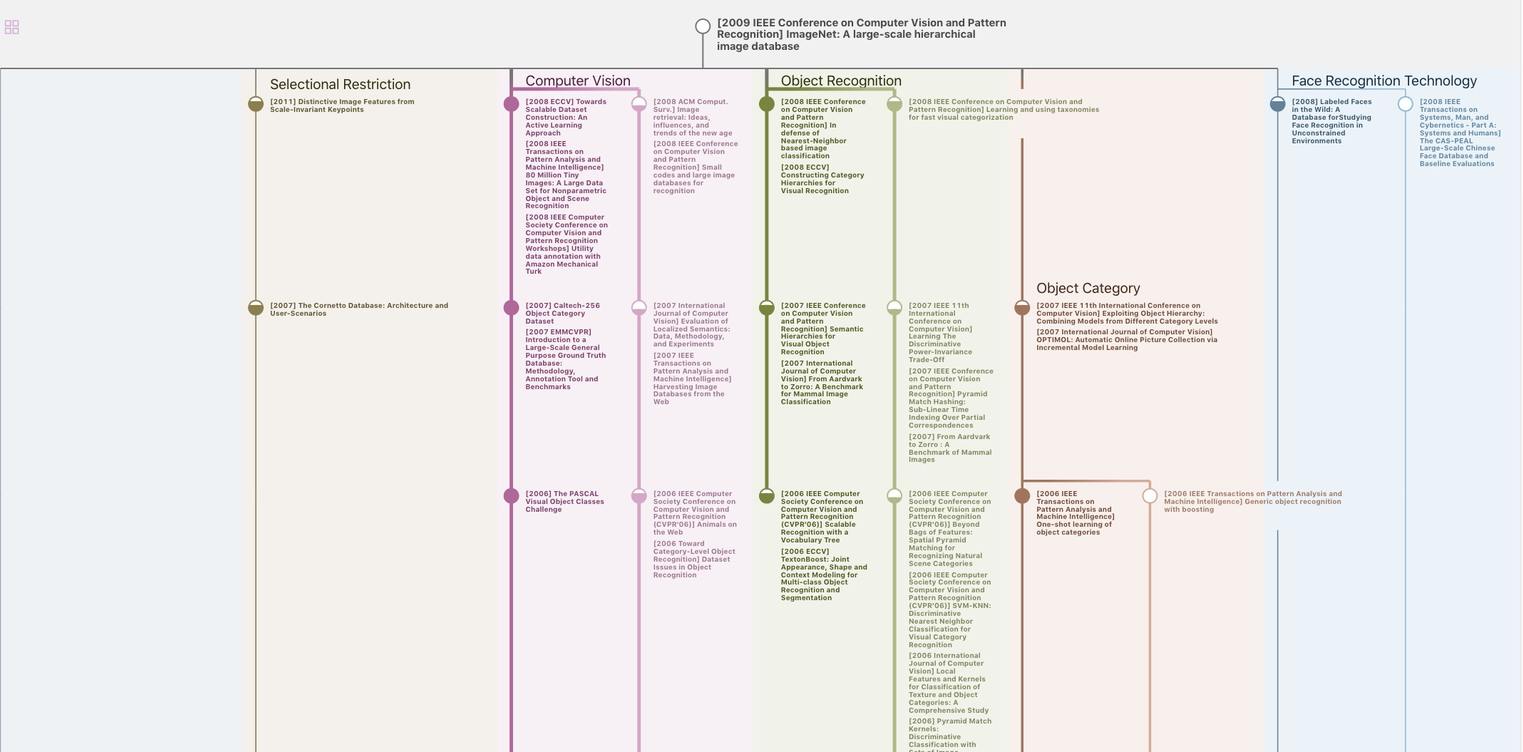
生成溯源树,研究论文发展脉络
Chat Paper
正在生成论文摘要