Water-Body Segmentation for SAR Images: Past, Current, and Future
REMOTE SENSING(2022)
摘要
Synthetic Aperture Radar (SAR), as a microwave sensor that can sense a target all day or night under all-weather conditions, is of great significance for detecting water resources, such as coastlines, lakes and rivers. This paper reviews literature published in the past 30 years in the field of water body extraction in SAR images, and makes some proposals that the community working with SAR image waterbody extraction should consider. Firstly, this review focuses on the main ideas and characteristics of traditional water body extraction on SAR images, mainly focusing on traditional Machine Learning (ML) methods. Secondly, how Deep Learning (DL) methods are applied and optimized in the task of water-body segmentation for SAR images is summarized from the two levels of pixel and image. We also pay more attention to the most popular networks, such as U-Net and its modified models, and novel networks, such as the Cascaded Fully-Convolutional Network (CFCN) and River-Net. In the end, an in-depth discussion is presented, along with conclusions and future trends, on the limitations and challenges of DL for water-body segmentation.
更多查看译文
关键词
synthetic aperture radar,water-body segmentation,Deep Learning,U-Net,River-Net
AI 理解论文
溯源树
样例
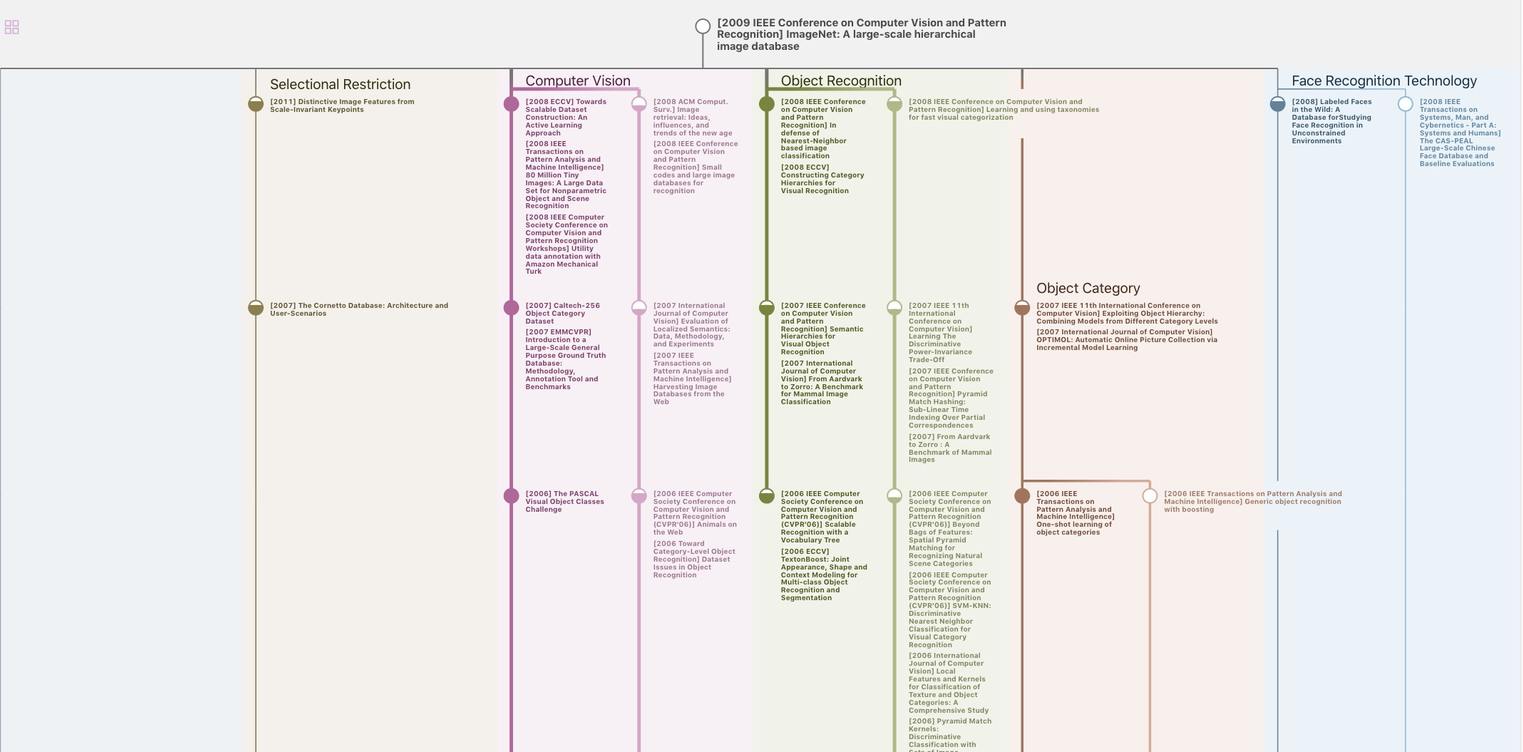
生成溯源树,研究论文发展脉络
Chat Paper
正在生成论文摘要