Self-Supervised Auxiliary Task Learning for Estimating Satellite Orientation
semanticscholar(2021)
摘要
Understanding the orientation or pose of a satellite is critical for space domain awareness. Recent advancements in direct pose estimation using convolutional neural networks (CNNs) have motivated us to examine a data-driven, end-to-end solution for estimating satellite pose. In this work, we use a CNN to directly estimate the pointing angle of a resolved LEO object. To improve the generalization of this task to varying objects, we perform classification as an auxiliary task for regularization. Both supervised and self-supervised learning methods are explored. We show on a large synthetic dataset, that multi-task self-supervision can improve the primary task of pointing angle estimation and improves generalization to held-out objects.
更多查看译文
AI 理解论文
溯源树
样例
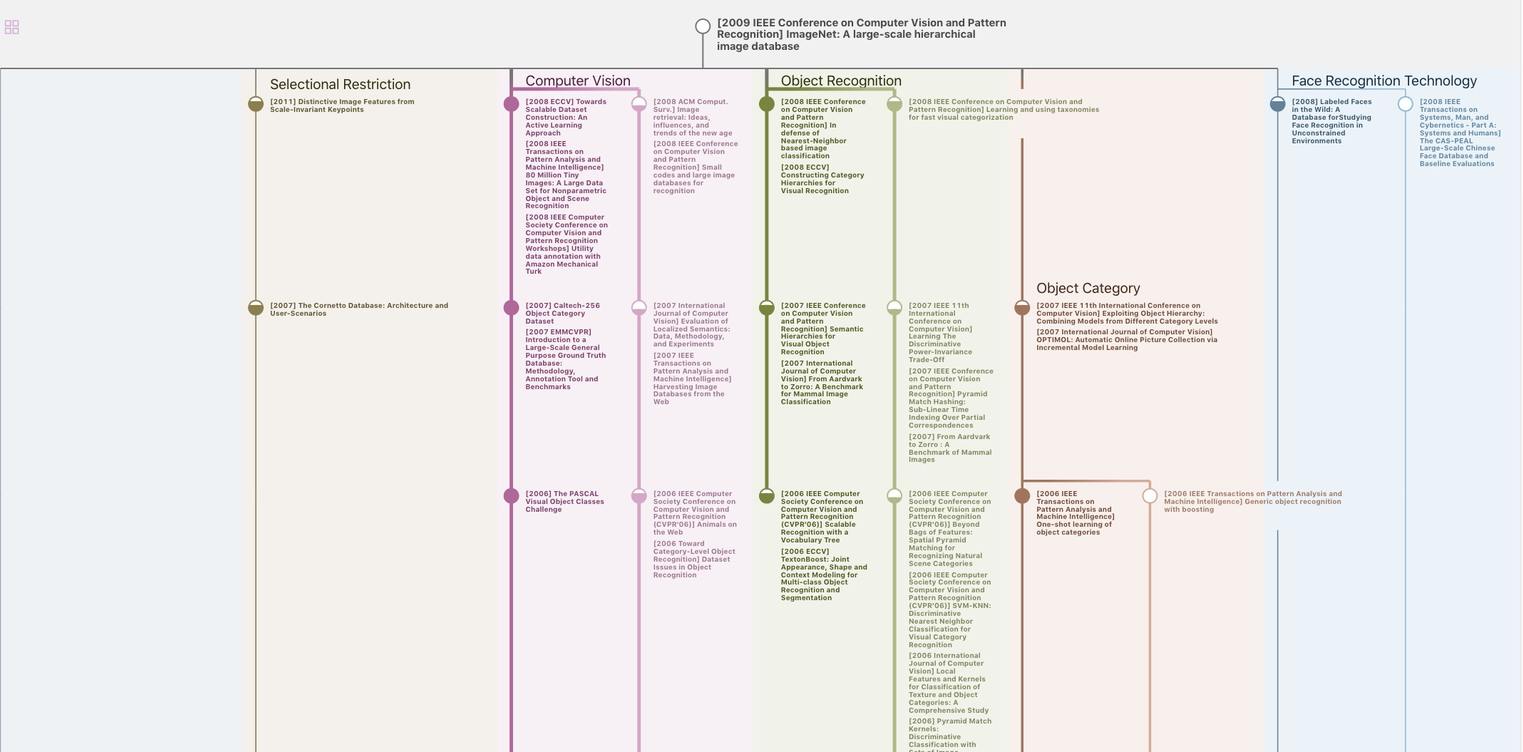
生成溯源树,研究论文发展脉络
Chat Paper
正在生成论文摘要