Collaborative Learning to Improve the Non-uniqueness of NMF
INTERNATIONAL JOURNAL OF COMPUTATIONAL INTELLIGENCE AND APPLICATIONS(2022)
摘要
Non-negative matrix factorization (NMF) is an unsupervised algorithm for clustering where a non-negative data matrix is factorized into (usually) two matrices with the property that all the matrices have no negative elements. This factorization raises the problem of instability, which means whenever we run NMF for the same dataset, we get different factorization. In order to solve the problem of non-uniqueness and to have a more stable solution, we propose a new approach that consists on collaborating different NMF models followed by a consensus. The proposed approach was validated on several datasets and the experimental results showed the effectiveness of our approach which is based on the reducing of standard reconstruction error in NMF model.
更多查看译文
关键词
Unsupervised learning,representations learning,collaborative clustering,non-negative matrix factorization,non-uniqueness of NMF,instability of NMF
AI 理解论文
溯源树
样例
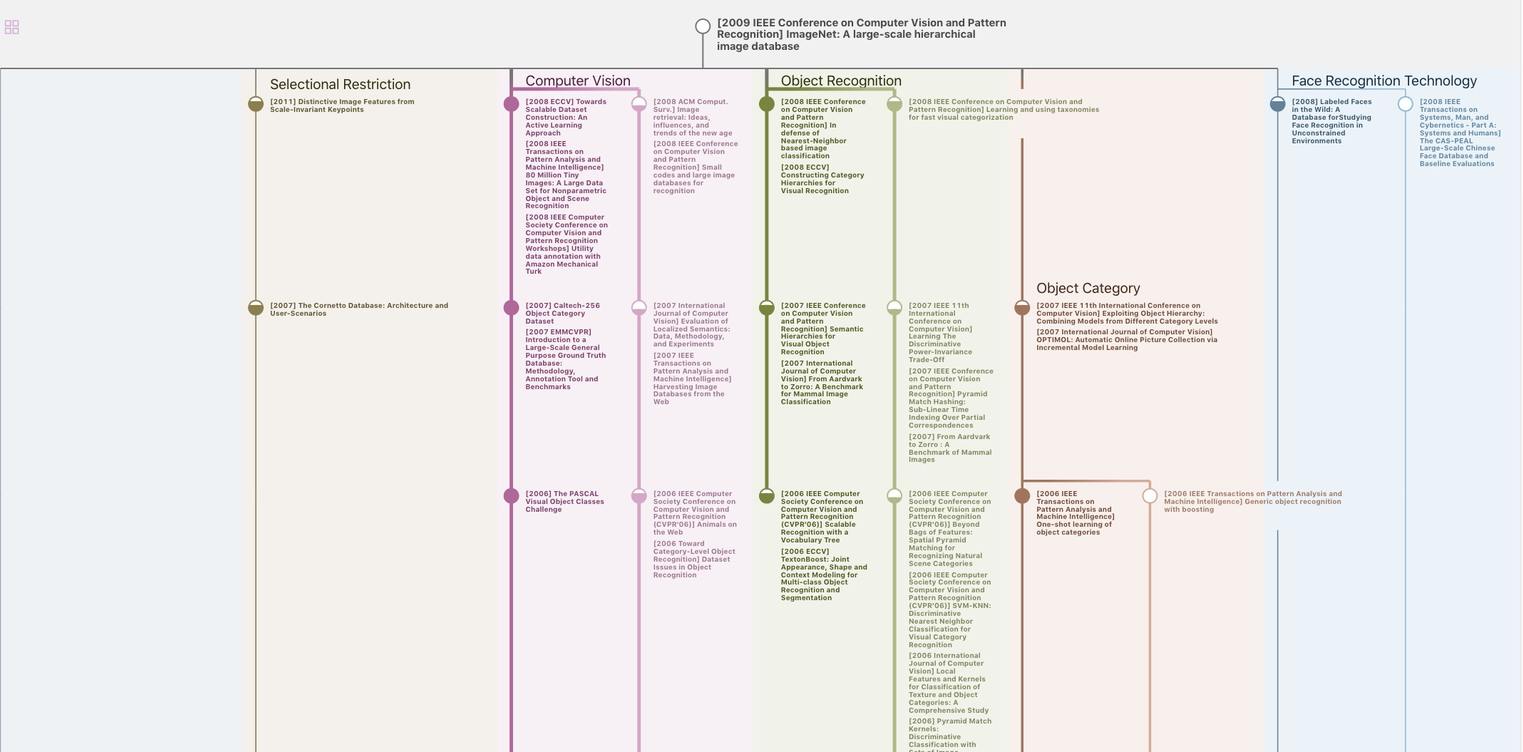
生成溯源树,研究论文发展脉络
Chat Paper
正在生成论文摘要