Pixel-Wise Semisupervised Fabric Defect Detection Method Combined With Multitask Mean Teacher
IEEE TRANSACTIONS ON INSTRUMENTATION AND MEASUREMENT(2022)
摘要
Fabric defect detection is a crucial problem in the production of textile industry. Nowadays, a large number of fabric defect detection algorithms based on deep learning have been proved to have excellent detection results, but the performance of detection often depends on large-scale labeled datasets. However, it is very laborious and costly to label a large number of pixel-level defect samples in industrial production. Although semisupervised detection method can reduce the labeling workload, fabric defect detection is still a challenging task due to the diversity of defects and the low contrast between defects and background. To address these issues, this article proposes a pixel-wise semisupervised fabric defect detection method combined with multitask mean teacher (MT). We focus on establishing a multitask detection model for defect area detection, defect contour detection, and defect distance map detection by leveraging their auxiliary information in better defect segmentation and assign this model as a student network and teacher network. Finally, the multitask supervised loss for labeled images and the multitask consistency loss for unlabeled images to train the network. Experiments on three public fabric defect detection datasets show that our method outperforms the current mainstream pixel-wise segmentation methods with MT, which verifies that the proposed network can effectively detect fabric defects by a small number of labeled samples.
更多查看译文
关键词
Fabrics,Multitasking,Image segmentation,Feature extraction,Production,Frequency-domain analysis,Training,Fabric defect detection,mean teacher (MT) network,multitask learning,pixel-wise segmentation,semisupervised learning
AI 理解论文
溯源树
样例
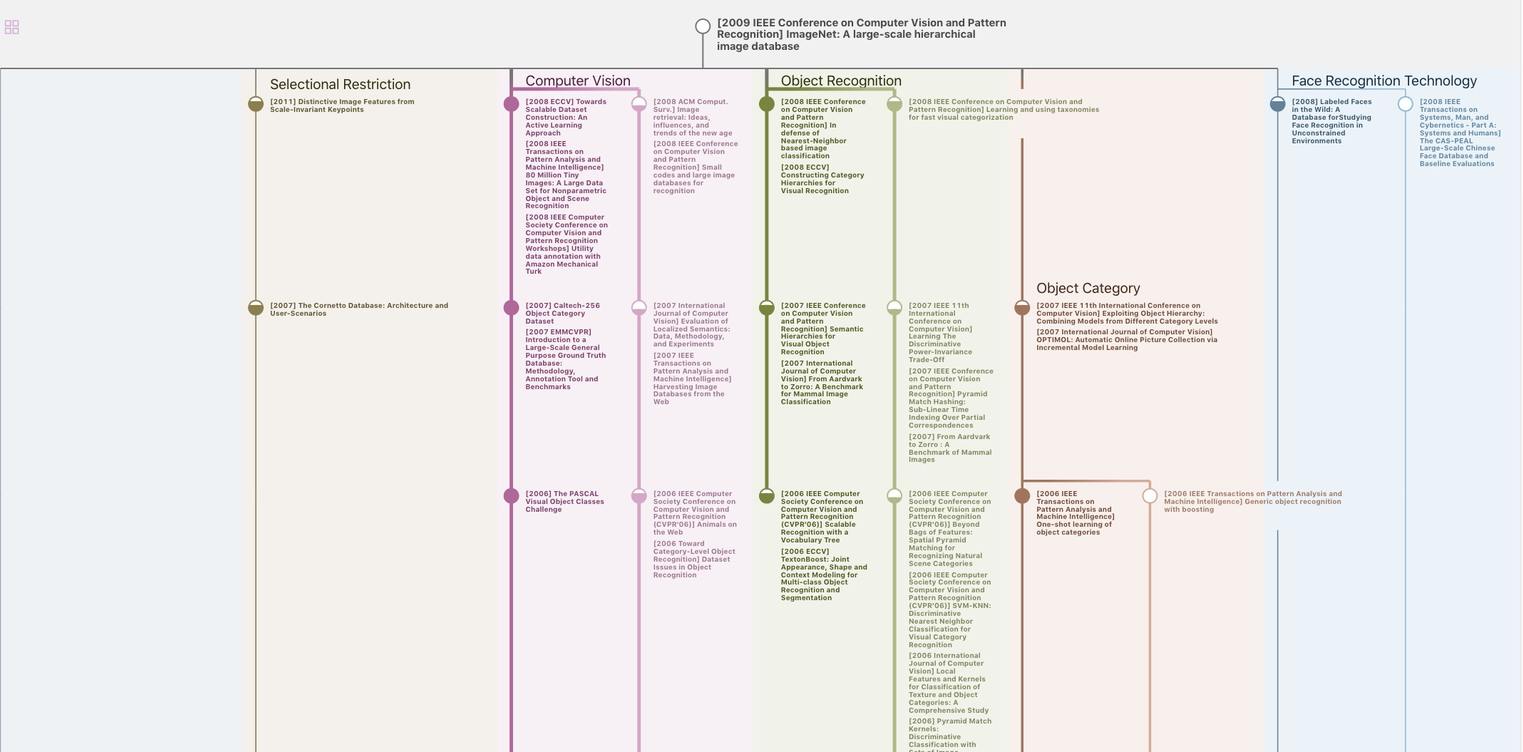
生成溯源树,研究论文发展脉络
Chat Paper
正在生成论文摘要