VQA-BC: Robust Visual Question Answering Via Bidirectional Chaining
ICASSP 2022 - 2022 IEEE International Conference on Acoustics, Speech and Signal Processing (ICASSP)(2022)
摘要
Current VQA models are suffering from the problem of overdependence on language bias, which severely reduces their robustness in real-world scenarios. In this paper, we analyze VQA models from the view of forward/backward chaining in the inference engine, and propose to enhance their robustness via a novel Bidirectional Chaining (VQA-BC) framework. Specifically, we introduce a backward chaining with hardnegative contrastive learning to reason from the consequence (answers) to generate crucial known facts (question-related visual region features). Furthermore, to alleviate the overconfident problem in answer prediction (forward chaining), we present a novel introspective regularization to connect forward and backward chaining with label smoothing. Extensive experiments verify that VQA-BC not only effectively overcomes language bias on out-of-distribution dataset, but also alleviates the over-correct problem caused by ensemble-based method on in-distribution dataset. Compared with competitive debiasing strategies, our method achieves state-of-the-art performance to reduce language bias on VQA-CP v2 dataset.
更多查看译文
关键词
Visual question answering,language bias,forward/backward chaining,label smoothing
AI 理解论文
溯源树
样例
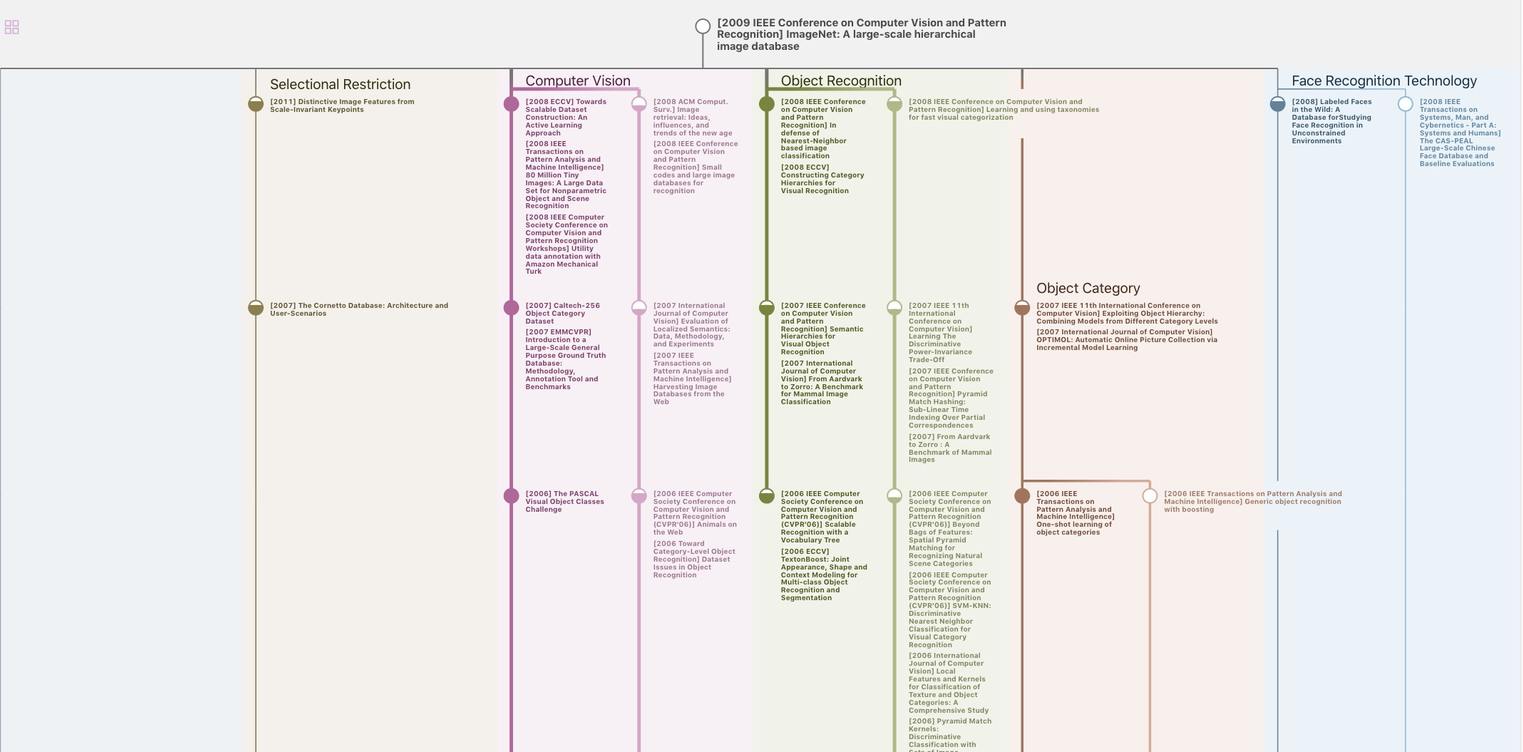
生成溯源树,研究论文发展脉络
Chat Paper
正在生成论文摘要