HELD: Hierarchical entity-label disambiguation in named entity recognition task using deep learning
INTELLIGENT DATA ANALYSIS(2022)
摘要
Named Entity Recognition (NER) is a challenging learning task of identifying and classifying entity mentions in texts into predefined categories. In recent years, deep learning (DL) methods empowered by distributed representations, such as word-and character-level embeddings, have been employed in NER systems. However, for information extraction in Police narrative reports, the performance of a DL-based NER approach is limited due to the presence of fine-grained ambiguous entities. For example, given the narrative report "Anna stole Ada's car", imagine that we intend to identify the VICTIM and the ROBBER, two sub-labels of PERSON. Traditional NER systems have limited performance in categorizing entity labels arranged in a hierarchical structure. Furthermore, it is unfeasible to obtain information from knowledge bases to give a disambiguated meaning between the entity mentions and the actual labels. This information must be extracted directly from the context dependencies. In this paper, we deal with the Hierarchical Entity-Label Disambiguation problem in Police reports without the use of knowledge bases. To tackle such a problem, we present HELD, an ensemble model that combines two components for NER: a BLSTM-CRF architecture and a NER tool. Experiments conducted on a real Police reports dataset show that HELD significantly outperforms baseline approaches.
更多查看译文
关键词
Fine-grained entity labels,hierarchical entity-label disambiguation using context,named entity recognition,deep learning,police reports domain
AI 理解论文
溯源树
样例
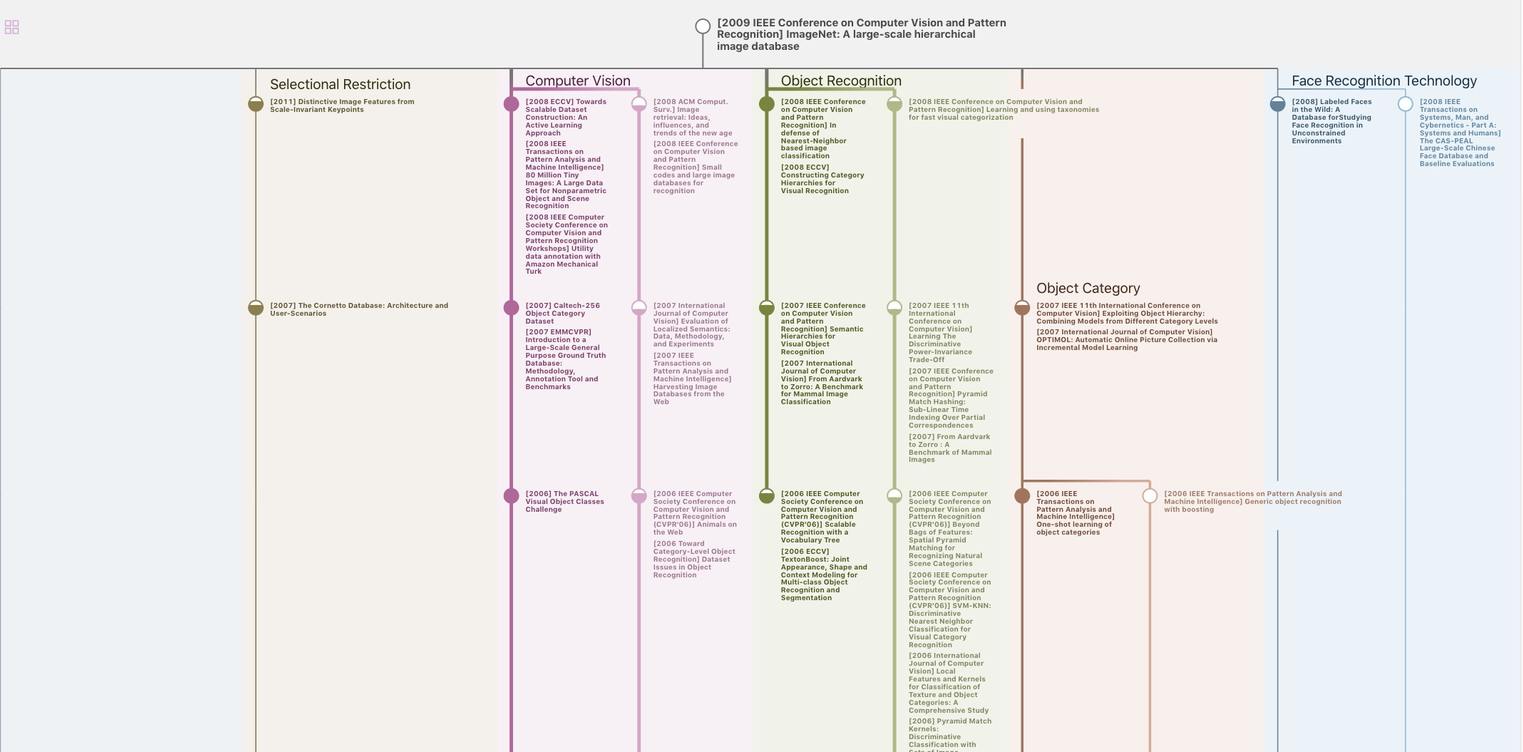
生成溯源树,研究论文发展脉络
Chat Paper
正在生成论文摘要