Energy-Efficient Multiprocessor-Based Computation and Communication Resource Allocation in Two-Tier Federated Learning Networks
IEEE Internet of Things Journal(2023)
摘要
In conventional federated learning (FL), multiple edge devices holding local data jointly train a machine learning model by communicating learning updates with a centralized aggregator without exchanging their data samples. Owing to the communication and computation bottleneck at the centralized aggregator and inaccurate learning model caused by the non-independent and identically distributed (IID) data, we here consider a two-tier FL network, in which Internet of Things (IoT) nodes are the core clients that hold data, the model aggregators at the middle tier are the low altitude aerial platforms (UAVs), and the model aggregator at the top-most layer is the high-altitude aerial platform (UAV with relatively high altitude). Under the assumption that each IoT node has parallel computing ability, we study the energy-efficient computation and communication resource allocation in such a network within some time budget. Upon formulating the problem as an optimization problem, we solve the computation and communication resource allocation problems as the separate subproblems within a time frame, and then propose an iterative algorithm to solve the entire problem jointly. More specifically, we solve both the energy-efficient computation and communication resource allocation subproblems using the dual decomposition technique, and then apply a bisection search-based recursive technique to solve the entire energy efficiency problem jointly. Moreover, we propose offline and online client scheduling schemes that not only select the optimal edge nodes for association but also assign workload to each client based on the data quality and workload constraint. With real data, extensive simulations are conducted to verify the effectiveness of the proposed resource allocation scheme. The results further reveal that the learning performance not only is dependent on the computation and communication energy consumption of the FL process but also the model divergence weight owing to the non-IID data at client IoT nodes.
更多查看译文
关键词
Computation and communication resource allocation,dual decomposition technique,energy-efficient resource allocation,federated learning (FL)
AI 理解论文
溯源树
样例
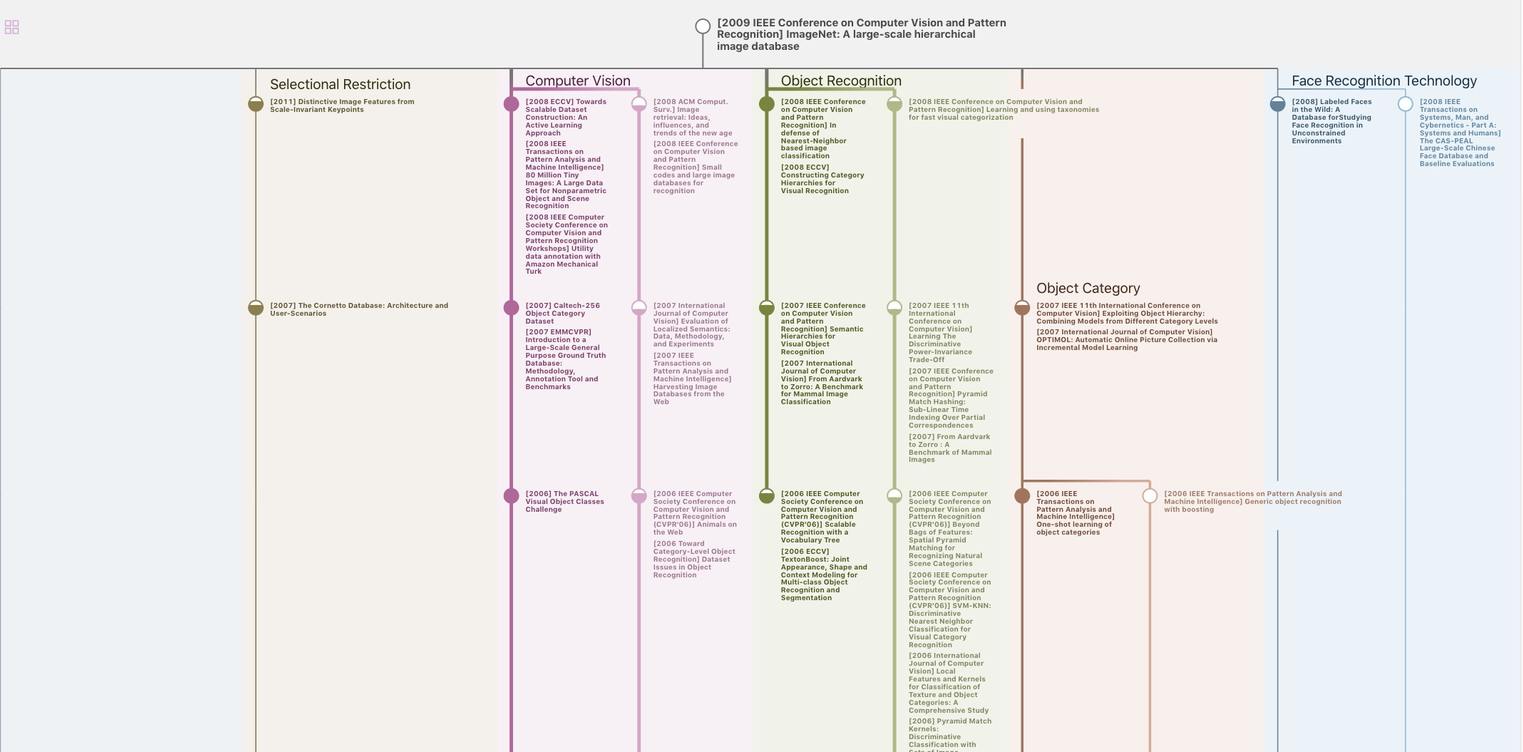
生成溯源树,研究论文发展脉络
Chat Paper
正在生成论文摘要